Enhancing sample efficiency for temperature control in ded with reinforcement learning and moose framework
PROCEEDINGS OF ASME 2023 INTERNATIONAL MECHANICAL ENGINEERING CONGRESS AND EXPOSITION, IMECE2023, VOL 3(2023)
Abstract
Directed Energy Deposition (DED) is crucial in additive manufacturing for various industries like aerospace, automotive, and biomedical. Precise temperature control is essential due to high-power lasers and dynamic environmental changes. Employing Reinforcement Learning (RL) can help with temperature control, but challenges arise from standardization and sample efficiency. In this study, a model-based Reinforcement Learning (MBRL) approach is used to train a DED model, improving control and efficiency. Computational models evaluate melt pool geometry and temporal characteristics during the process. The study employs the Allen-Cahn phase field (AC-PF) model using the Finite Element Method (FEM) with the Multi-physics Object-Oriented Simulation Environment (MOOSE). MBRL, specifically Dyna-Q+, outperforms traditional Q-learning, requiring fewer samples. Insights from this research aid in advancing RL techniques for laser metal additive manufacturing.
MoreTranslated text
Key words
MOOSE,DED,Reinforcement Learning,Model-Based,Q-learning,Dyna-Q
AI Read Science
Must-Reading Tree
Example
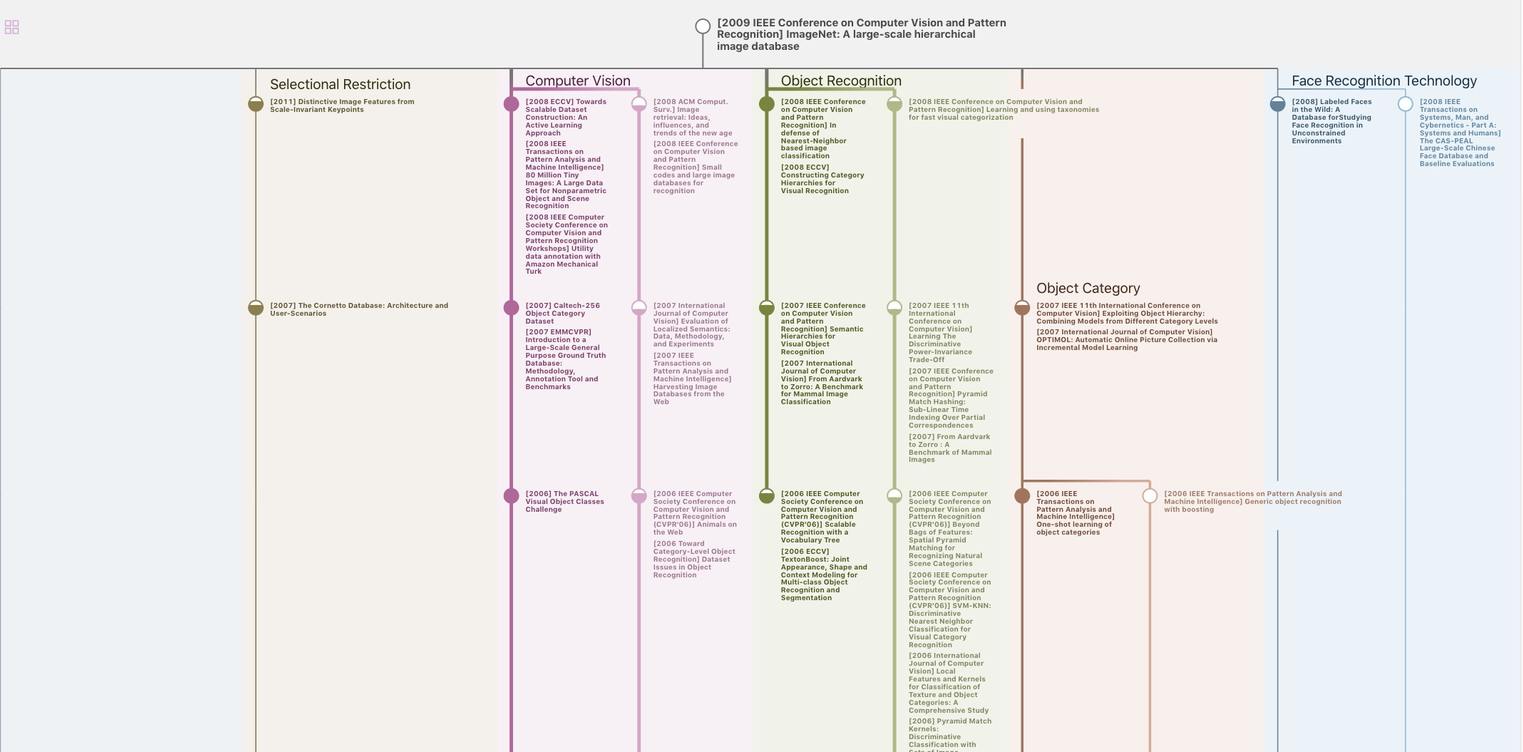
Generate MRT to find the research sequence of this paper
Chat Paper
Summary is being generated by the instructions you defined