Prediction for geometric degradation of track on machine learning
semanticscholar(2023)
摘要
High track quality is the basis of ensuring the safe and comfortable operation of trains, and it is of great significance to accurately grasp the degradation law of track geometry to ensure track quality. Long Short-Term Memory (LSTM) network in machine learning has the function of remembering historical information, which can better predict the development trend of nonlinear time series, a prediction model of orbital geometric degradation based on LSTM is constructed by using historical data of track quality index (TQI). In order to verify the validity of the model, the test data of Lanzhou-Urumqi High-speed Railway Track Inspection Vehicle are selected. The results show that the prediction model of track geometric degradation based on LSTM established in this paper is effective and has high prediction accuracy.
更多查看译文
AI 理解论文
溯源树
样例
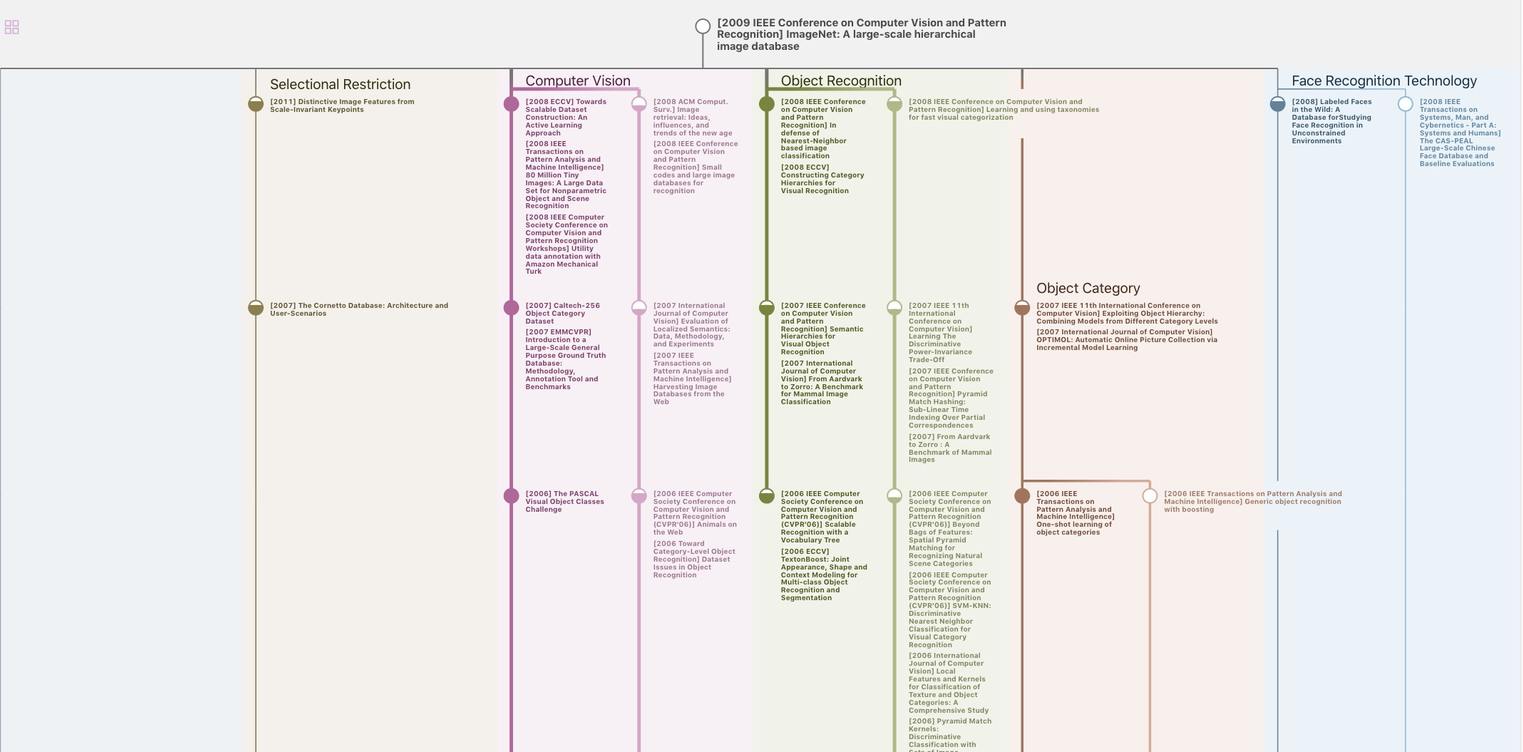
生成溯源树,研究论文发展脉络
Chat Paper
正在生成论文摘要