Development and validation of AI/ML derived splice-switching oligonucleotides
MOLECULAR SYSTEMS BIOLOGY(2024)
Abstract
Splice-switching oligonucleotides (SSOs) are antisense compounds that act directly on pre-mRNA to modulate alternative splicing (AS). This study demonstrates the value that artificial intelligence/machine learning (AI/ML) provides for the identification of functional, verifiable, and therapeutic SSOs. We trained XGboost tree models using splicing factor (SF) pre-mRNA binding profiles and spliceosome assembly information to identify modulatory SSO binding sites on pre-mRNA. Using Shapley and out-of-bag analyses we also predicted the identity of specific SFs whose binding to pre-mRNA is blocked by SSOs. This step adds considerable transparency to AI/ML-driven drug discovery and informs biological insights useful in further validation steps. We applied this approach to previously established functional SSOs to retrospectively identify the SFs likely to regulate those events. We then took a prospective validation approach using a novel target in triple negative breast cancer (TNBC), NEDD4L exon 13 (NEDD4Le13). Targeting NEDD4Le13 with an AI/ML-designed SSO decreased the proliferative and migratory behavior of TNBC cells via downregulation of the TGF beta pathway. Overall, this study illustrates the ability of AI/ML to extract actionable insights from RNA-seq data. Machine learning models are trained to identify functional splice-switching oligonucleotides (SSOs) and to predict the splicing factors (SFs) inhibited. SSOs are developed for a novel target in Triple Negative Breast Cancer.Three sources of splicing regulatory information are used to train interpretable XGboost models that predict binding sites for functional SSOs and allow for the identification of SFs regulated by an SSO. The model shows high predictive accuracy in SF-binding perturbation positions critical for AS regulation. A novel alternative splicing target is identified in TNBC, NEDD4L exon 13 (NEDD4Le13). Targeting NEDD4Le13 with the SSO predicted by the model decreases TNBC cell proliferation and migration via downregulation of the TGF beta pathway. Machine learning models are trained to identify functional splice-switching oligonucleotides (SSOs) and to predict the splicing factors (SFs) inhibited. SSOs are developed for a novel target in Triple Negative Breast Cancer.
MoreTranslated text
Key words
Alternative Splicing,Machine Learning,Splice-Switching Oligonucleotides,Splicing Factors,Triple Negative Breast Cancer
AI Read Science
Must-Reading Tree
Example
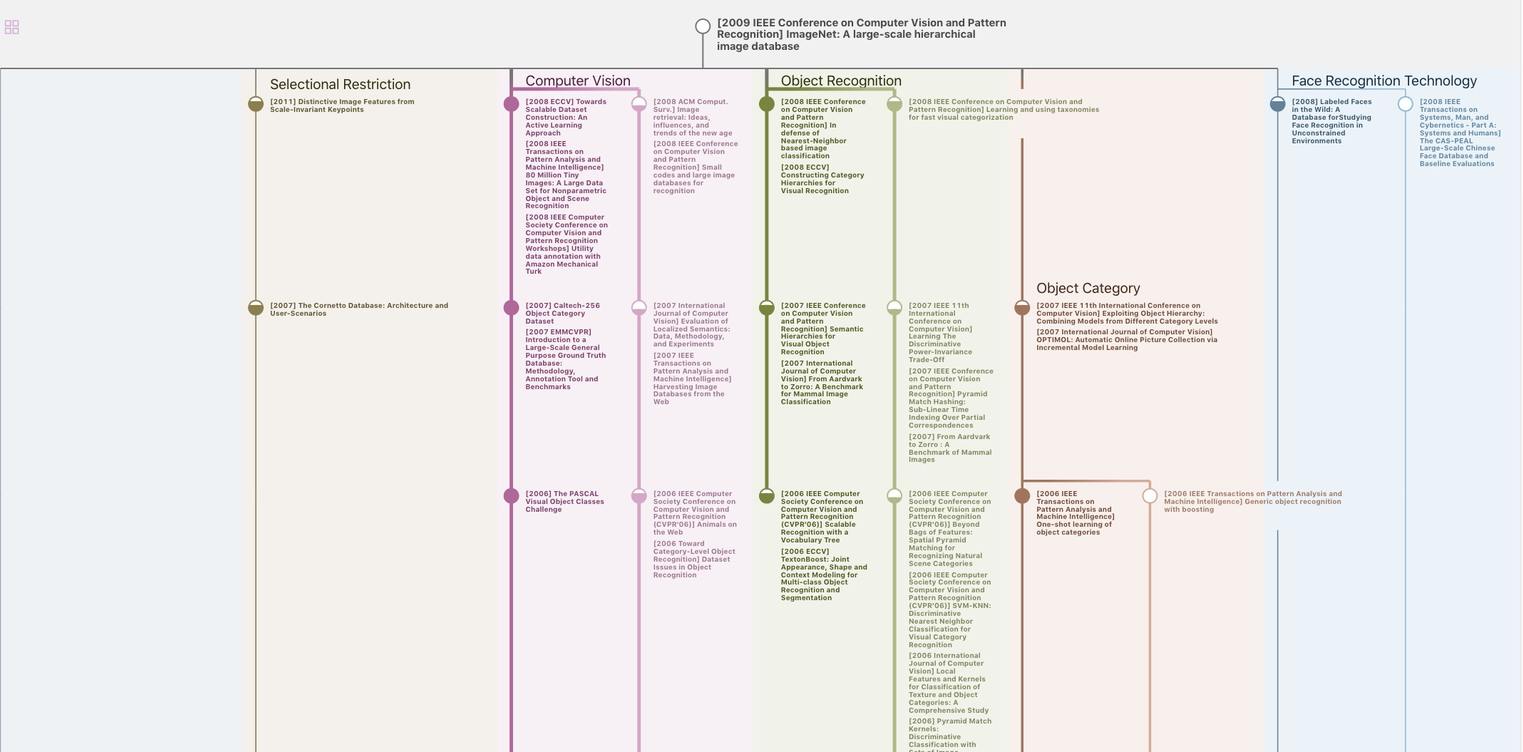
Generate MRT to find the research sequence of this paper
Chat Paper
Summary is being generated by the instructions you defined