Resource-Constraint Bayesian Optimization for Soft Processors on FPGAs.
International Symposium on Highly Efficient Accelerators and Reconfigurable Technologies(2024)
摘要
This paper introduces a Bayesian optimization method tailored for soft processors on FPGAs. It addresses the challenge of tuning soft processor parameters to align performance, power efficiency, and resource allocation with application-specific demands. It presents three innovations: a strategy based on a constraint function for enforcing usage constraints across various FPGA resources, a refined approach for managing restricted integer parameters beyond simple rounding, and a comprehensive evaluation via four case studies on an open-source parametric RISC-V design. A key advantage of the proposed approach over existing ones is that it enables users to set constraints on hardware resource usage, ensuring that the optimized design fits into the target FPGA. This method not only streamlines the optimization process but also ensures adherence to hardware resource constraints. Experimental results show that our proposed approach substantially outperforms existing methods for optimizing FPGA-based soft processors, achieving a 12% higher success rate in identifying the best processor design and requiring approximately 23% fewer optimization iterations.
更多查看译文
AI 理解论文
溯源树
样例
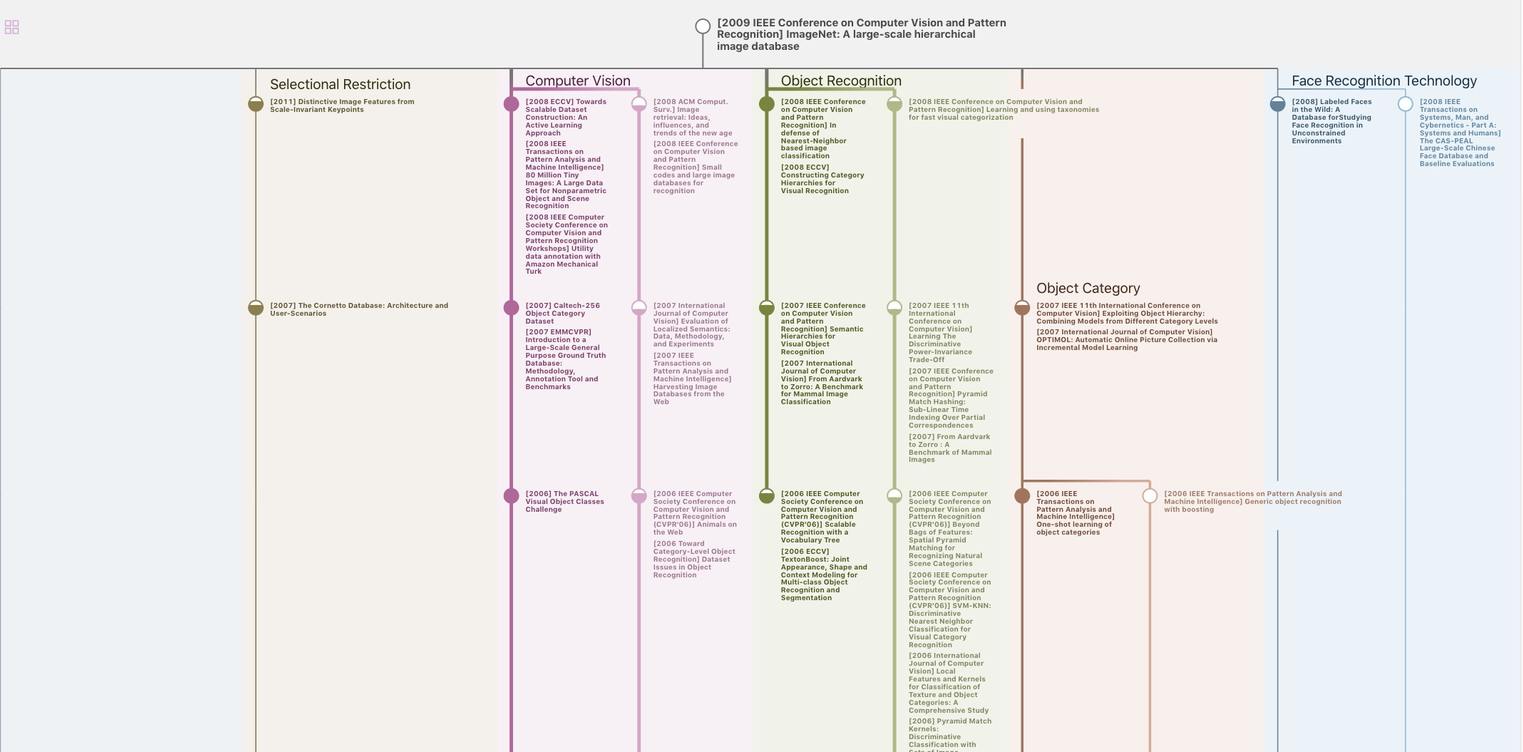
生成溯源树,研究论文发展脉络
Chat Paper
正在生成论文摘要