NIR-sighted: A Programmable Streaming Architecture for Low-Energy Human-Centric Vision Applications
ACM Transactions on Embedded Computing Systems(2023)
摘要
Human studies often rely on wearable lifelogging cameras that capture videos of individuals and their surroundings to aid in visual confirmation or recollection of daily activities like eating, drinking and smoking. However, this may include private or sensitive information that may cause some users to refrain from using such monitoring devices. Also, short battery lifetime and large form factors reduce applicability for long-term capture of human activity. Solving this triad of interconnected problems is challenging due to wearable embedded systems’ energy, memory and computing constraints. Inspired by this critical use case and the unique design problem, we developed NIR-sighted, an architecture for wearable video cameras which navigates this design space via three key ideas: i) Reduce storage and enhance privacy by discarding masked pixels and frames. ii) Enable programmers to generate effective masks with low computational overhead. iii) Enable the use of small MCUs by moving masking and compression off-chip. Combined together in an end-to-end system, NIR-sighted’s masking capabilities and off-chip compression hardware shrinks systems, stores less data, and enables programmer-defined obfuscation to yield privacy enhancement. The user’s privacy is enhanced significantly as nowhere in the pipeline is any part of the image stored before it is obfuscated. We design a wearable camera called NIR-sightedCam based on this architecture; it is compact and can record IR and grayscale video at 16 and 20+fps respectively for 26 hours nonstop (59 hours with IR disabled) at a fraction of comparable platforms power draw. NIR-sightedCam includes a low-power FPGA which implements our mJPEG compress/obfuscate hardware, Blindspot. We additionally show the potential for privacy-enhancing function and clinical utility via an in-lab eating study, validated by a nutritionist.
更多查看译文
AI 理解论文
溯源树
样例
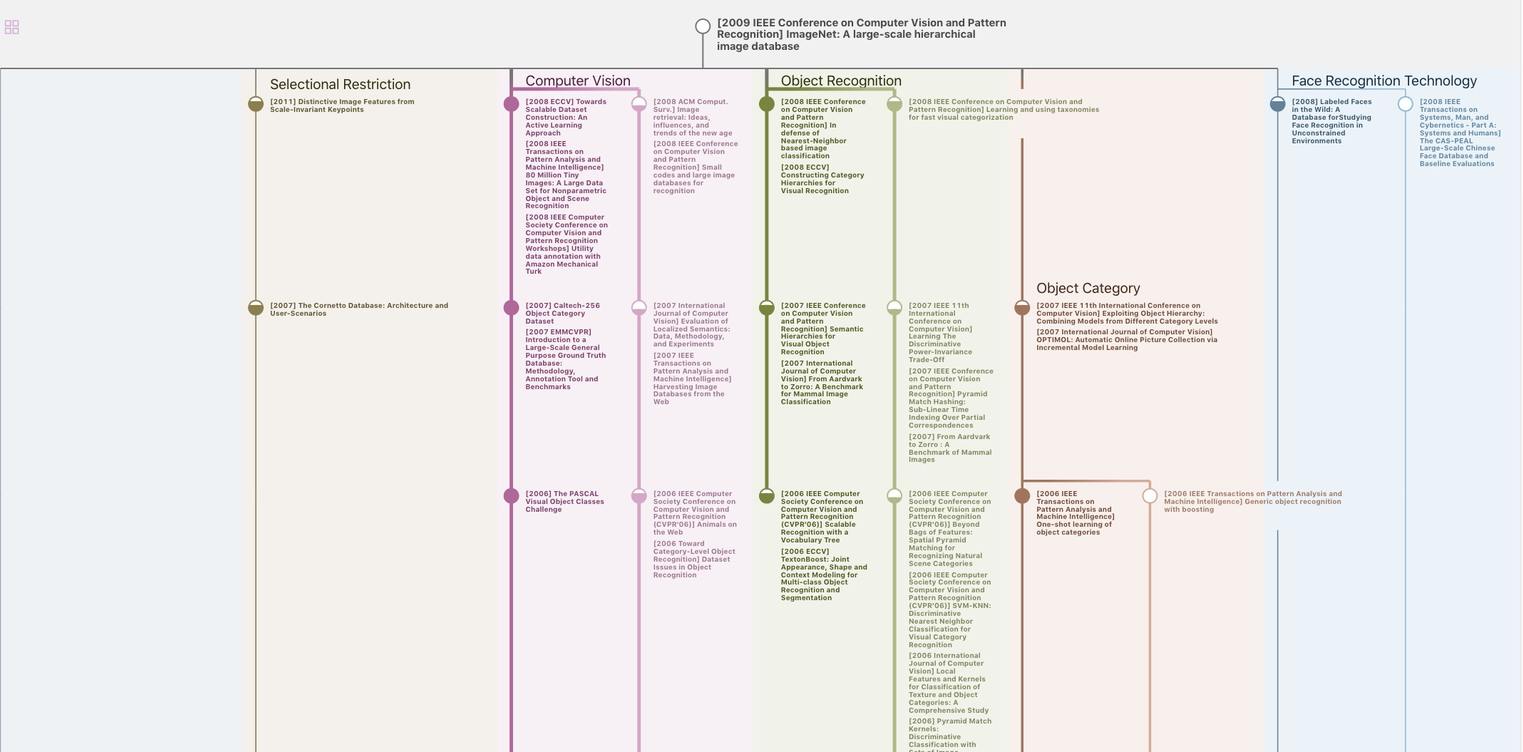
生成溯源树,研究论文发展脉络
Chat Paper
正在生成论文摘要