LeapFrog: Getting the Jump on Multi-Scale Materials Simulations Using Machine Learning
arxiv(2024)
Abstract
The development of novel materials in recent years has been accelerated
greatly by the use of computational modelling techniques aimed at elucidating
the complex physics controlling microstructure formation in materials, the
properties of which control material function. One such technique is the phase
field method, a field theoretic approach that couples various thermophysical
fields to microscopic order parameter fields that track the phases of
microstructure. Phase field models are framed as multiple, non-linear, partial
differential equations, which are extremely challenging to compute efficiently.
Recent years have seen an explosion of computational algorithms aimed at
enhancing the efficiency of phase field simulations. One such technique,
adaptive mesh refinement (AMR), dynamically adapts numerical meshes to be
highly refined around steep spatial gradients of the PDE fields and coarser
where the fields are smooth. This reduces the number of computations per time
step significantly, thus reducing the total time of computation. What AMR
doesn't do is allow for adaptive time stepping. This work combines AMR with a
neural network algorithm that uses a U-Net with a Convolutional Long-Short Term
Memory (CLSTM) base to accelerate phase field simulations. Our neural network
algorithm is described in detail and tested in on simulations of directional
solidification of a dilute binary alloy, a paradigm that is highly practical
for its relevance to the solidification of alloys.
MoreTranslated text
AI Read Science
Must-Reading Tree
Example
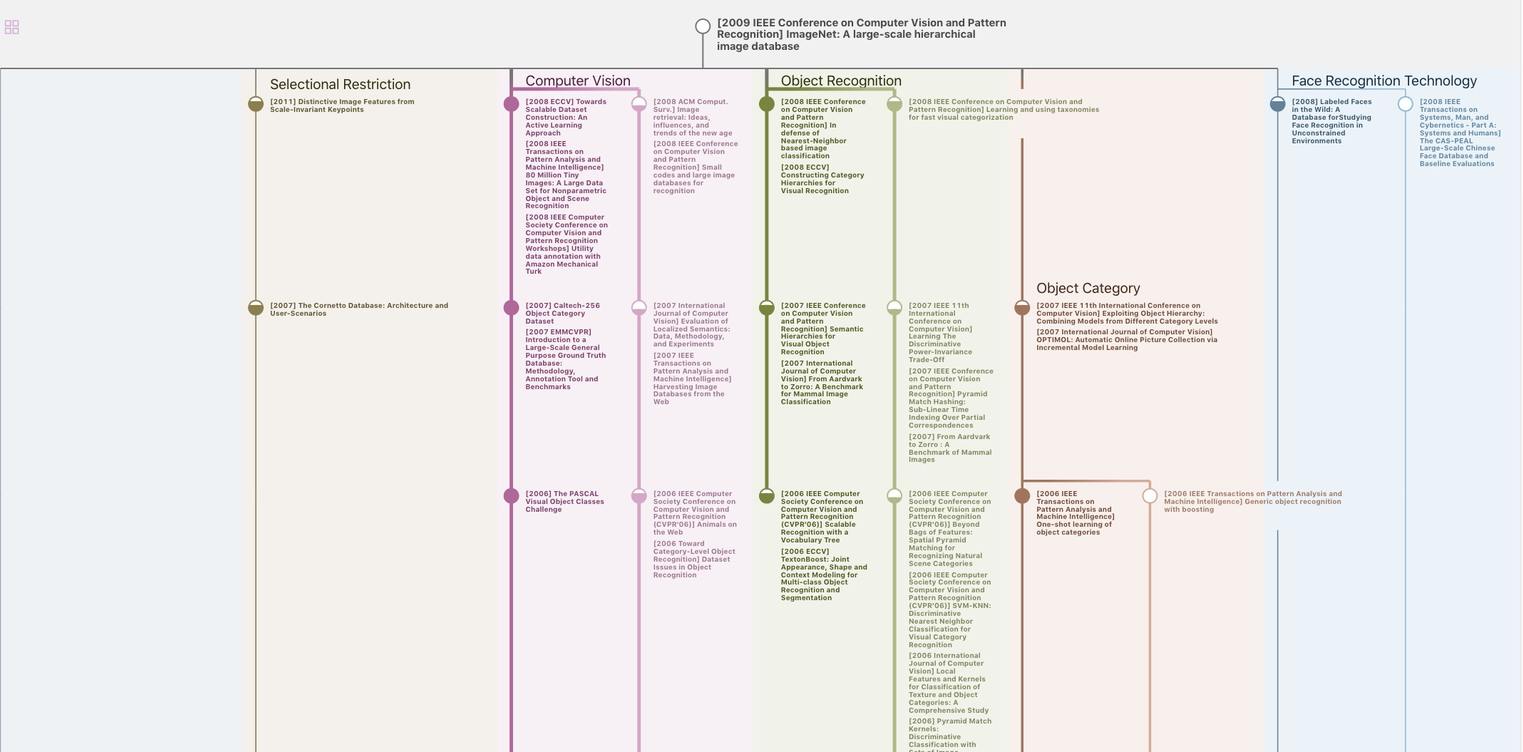
Generate MRT to find the research sequence of this paper
Chat Paper
Summary is being generated by the instructions you defined