Adaptive Manipulation using Behavior Trees
arxiv(2024)
摘要
Many manipulation tasks use instances of a set of common motions, such as a
twisting motion for tightening or loosening a valve. However, different
instances of the same motion often require different environmental parameters
(e.g. force/torque level), and thus different manipulation strategies to
successfully complete; for example, grasping a valve handle from the side
rather than head-on to increase applied torque. Humans can intuitively adapt
their manipulation strategy to best suit such problems, but representing and
implementing such behaviors for robots remains an open question. We present a
behavior tree-based approach for adaptive manipulation, wherein the robot can
reactively select from and switch between a discrete set of manipulation
strategies during task execution. Furthermore, our approach allows the robot to
learn from past attempts to optimize performance, for example learning the
optimal strategy for different task instances. Our approach also allows the
robot to preempt task failure and either change to a more feasible strategy or
safely exit the task before catastrophic failure occurs. We propose a simple
behavior tree design for general adaptive robot behavior and apply it in the
context of industrial manipulation. The adaptive behavior outperformed all
baseline behaviors that only used a single manipulation strategy, markedly
reducing the number of attempts and overall time taken to complete the example
tasks. Our results demonstrate potential for improved robustness and efficiency
in task completion, reducing dependency on human supervision and intervention.
更多查看译文
AI 理解论文
溯源树
样例
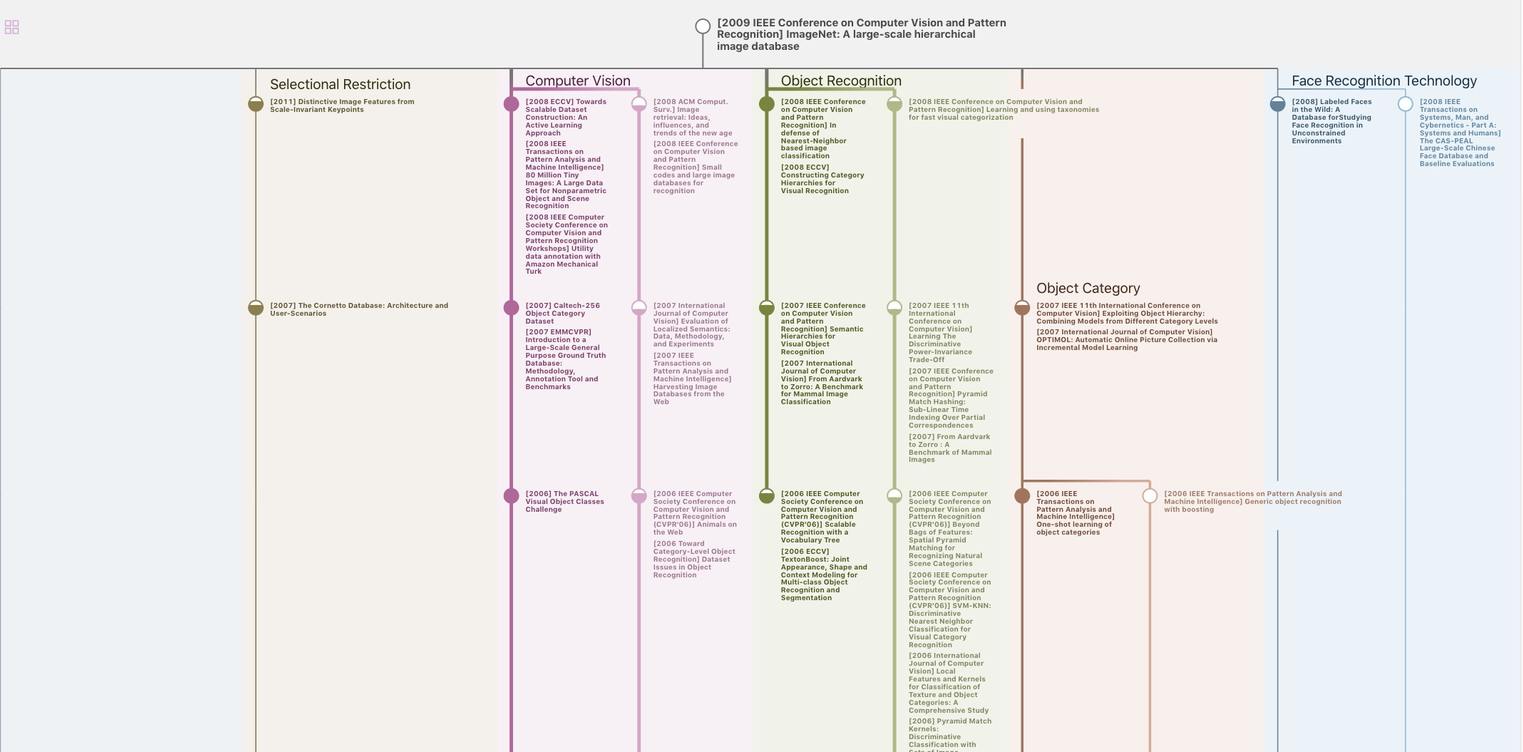
生成溯源树,研究论文发展脉络
Chat Paper
正在生成论文摘要