MRI Field-transfer Reconstruction with Limited Data: Regularization by Neural Style Transfer
arXivorg(2023)
摘要
Recent works have demonstrated success in MRI reconstruction using deep learning-based models. However, most reported approaches require training on a task-specific, large-scale dataset. Regularization by denoising (RED) is a general pipeline which embeds a denoiser as a prior for image reconstruction. The potential of RED has been demonstrated for multiple image-related tasks such as denoising, deblurring and super-resolution. In this work, we propose a regularization by neural style transfer (RNST) method to further leverage the priors from the neural transfer and denoising engine. This enables RNST to reconstruct a high-quality image from a noisy low-quality image with different image styles and limited data. We validate RNST with clinical MRI scans from 1.5T and 3T and show that RNST can significantly boost image quality. Our results highlight the capability of the RNST framework for MRI reconstruction and the potential for reconstruction tasks with limited data.
更多查看译文
AI 理解论文
溯源树
样例
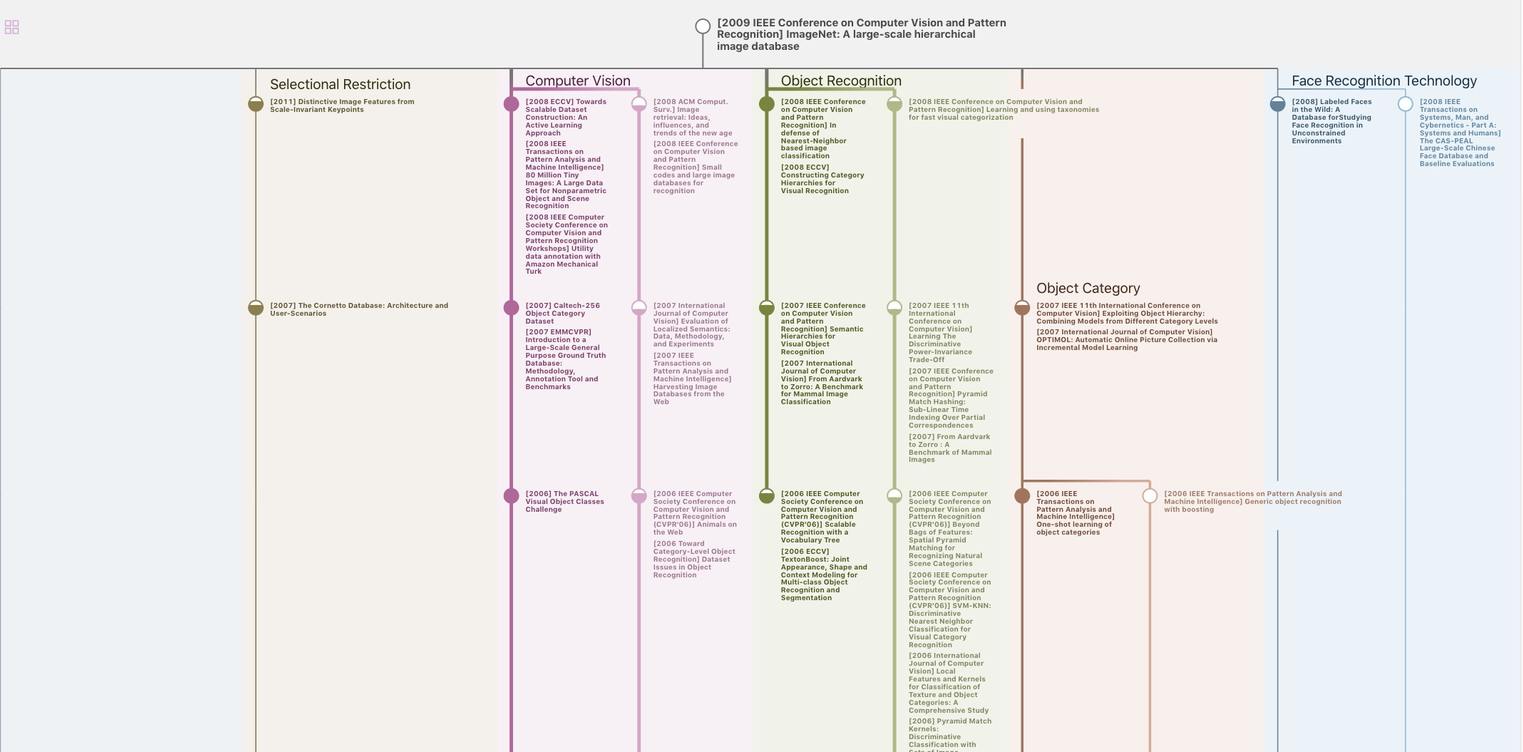
生成溯源树,研究论文发展脉络
Chat Paper
正在生成论文摘要