Deep Spiking Residual Shrinkage Network for Bearing Fault Diagnosis.
IEEE Transactions on Cybernetics(2022)
Abstract
Bearing fault diagnosis of electrical equipment has been a popular research area in recent years because there are often some faults during continuous operation in production due to the harsh working environment. However, the traditional fault signal processing methods rely on highly expert experience, and some parameters are difficult to be optimized by machine-learning methods. Thus, the satisfactory recognition accuracy of fault diagnosis cannot be achieved in the above methods. In this article, a new model based on the spiking neural network (SNN) is proposed, which is called deep the spiking residual shrinkage network (DSRSN) for bearing fault diagnosis. In the model, attention mechanisms and soft thresholding are introduced to improve the recognition rate under a high-level noise background. The higher recognition accuracy is obtained in the proposed model which is tested on the fault signal dataset under different noise intensities. Meanwhile, the training time is about treble as fast as the training time of the artificial neural network, which is reflecting the high efficiency of SNN.
MoreTranslated text
Key words
Bearing fault diagnosis,deep learning,soft thresh-olding,spiking neural network (SNN)
AI Read Science
Must-Reading Tree
Example
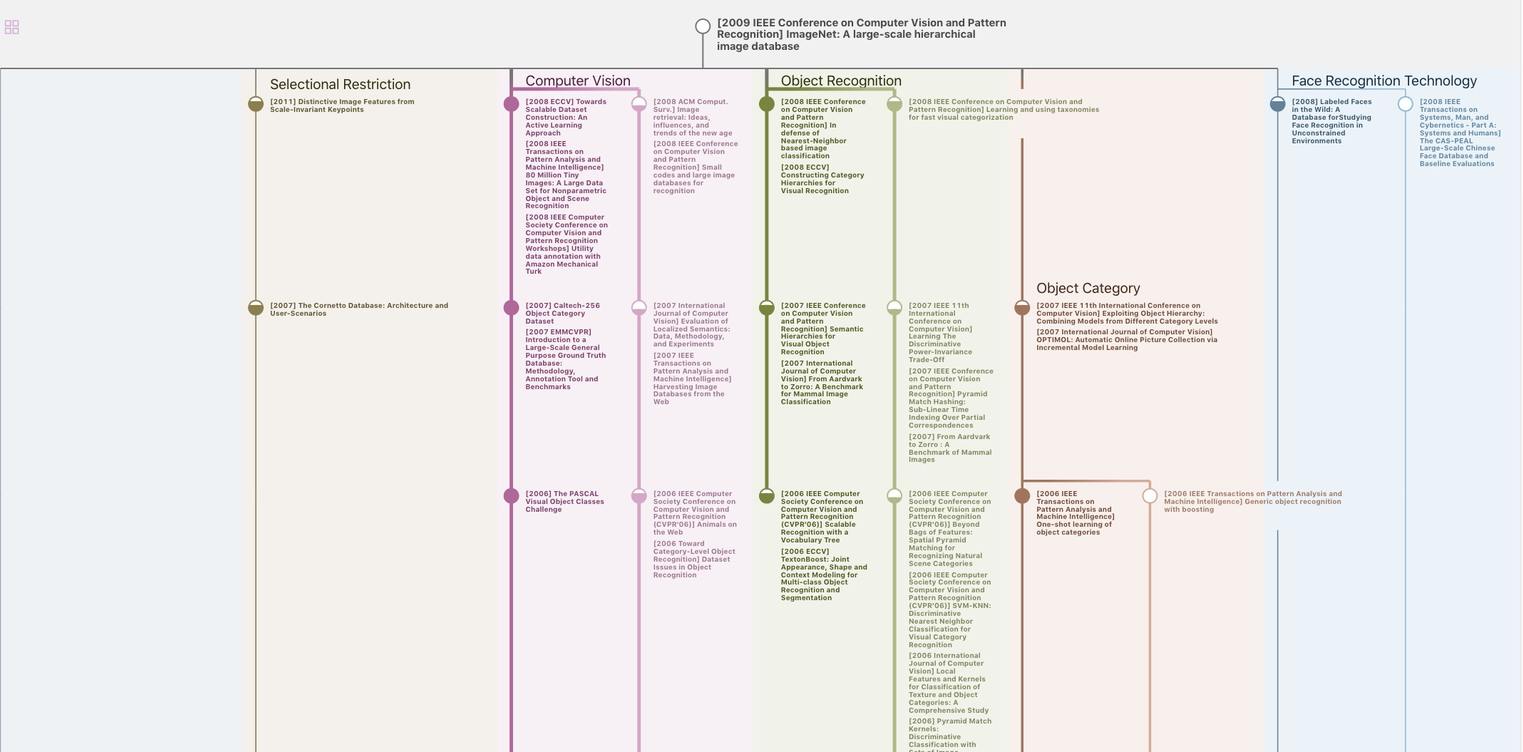
Generate MRT to find the research sequence of this paper
Chat Paper
Summary is being generated by the instructions you defined