Randomnet: clustering time series using untrained deep neural networks
Data Mining and Knowledge Discovery(2024)
摘要
Neural networks are widely used in machine learning and data mining. Typically, these networks need to be trained, implying the adjustment of weights (parameters) within the network based on the input data. In this work, we propose a novel approach, RandomNet, that employs untrained deep neural networks to cluster time series. RandomNet uses different sets of random weights to extract diverse representations of time series and then ensembles the clustering relationships derived from these different representations to build the final clustering results. By extracting diverse representations, our model can effectively handle time series with different characteristics. Since all parameters are randomly generated, no training is required during the process. We provide a theoretical analysis of the effectiveness of the method. To validate its performance, we conduct extensive experiments on all of the 128 datasets in the well-known UCR time series archive and perform statistical analysis of the results. These datasets have different sizes, sequence lengths, and they are from diverse fields. The experimental results show that the proposed method is competitive compared with existing state-of-the-art methods.
更多查看译文
关键词
Time series clustering,Scalable,Random kernels,Deep neural network
AI 理解论文
溯源树
样例
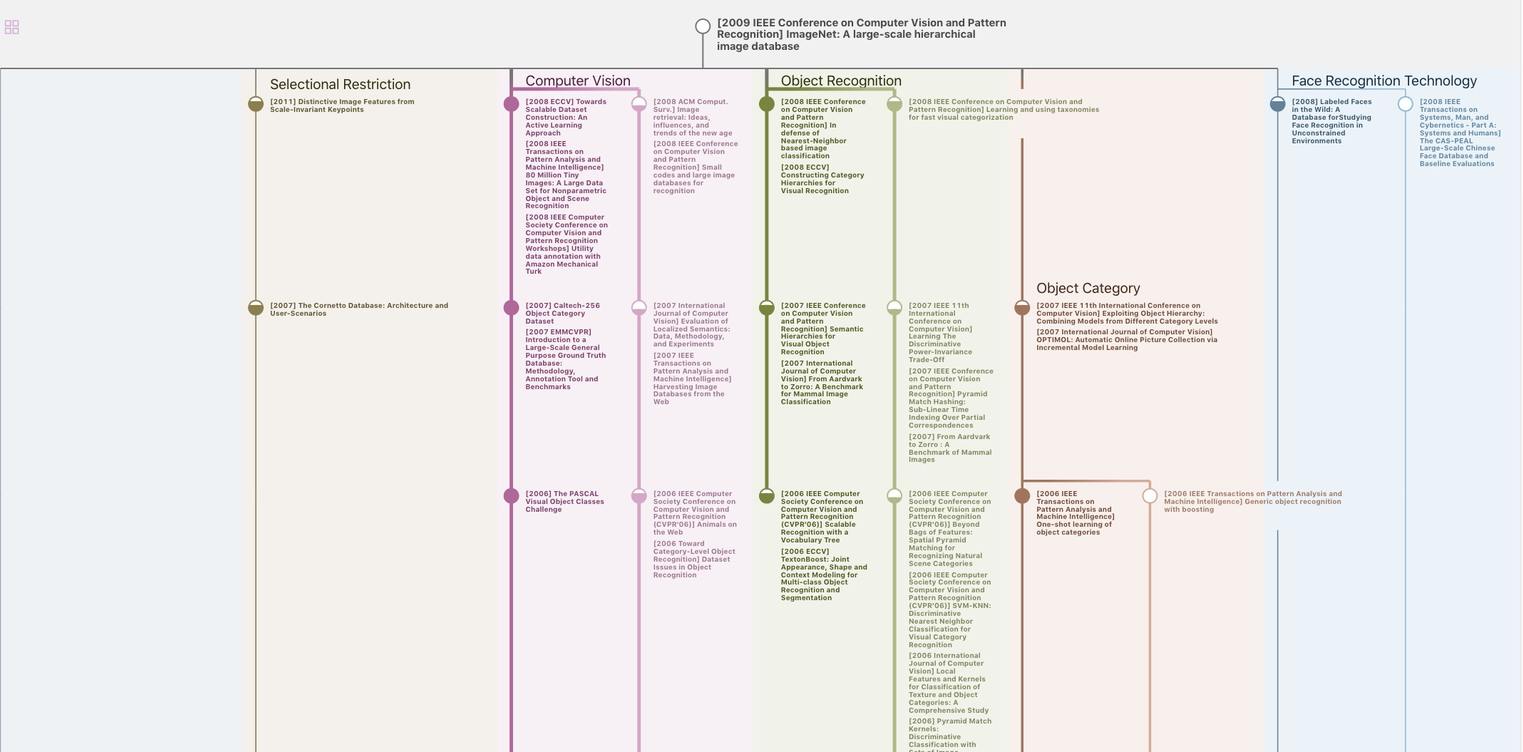
生成溯源树,研究论文发展脉络
Chat Paper
正在生成论文摘要