Leveraging Deep Learning Models for Accurate and Reproducible Cardiac Measurements in Echocardiography
2024 International Conference on E-mobility, Power Control and Smart Systems (ICEMPS)(2024)
摘要
The primary metrics recorded during ultrasound sessions focused on cardiac assessment are focused on the size of the heart's features, its two chambers and pre-chambers. Presently, these measurements are predominantly conducted manually, relying on the subjective judgment of medical professionals. A sonographer takes a transthoracic echocardiogram by first applying a viscous ultrasound gel on the patient at five distinct physical locations and placing the transducer on these positions at various angles. Each angle captures slightly different perspectives of the heart called views. From each view we can make out cutouts of cardiac structures and see motion of valves and walls. This study introduces an innovative approach to cardiac assessment which delegates the evaluation task to a trained algorithm. This has the potential to expedite the measurement process and help clinicians in the interpretation of the results by proposing common cardiac issues based on pre-trained data. In the paper we provide a process for intelligent extraction of diameters along the left ventricular outflow tract (LVOT) and aortic apparatus using only the ultrasound image. We show that it is possible to successfully train a model which can extract the measurements of the diameter of the LVOT in mid systole, the diameter of the aorta annulus (AA) in mid-systole, the diameter at the aortic sinus of Valsalva (ASV) at end diastole, and the diameter of the Sino tubular junction (SJ) at end diastole. With these measures extracted, the model uses machine learning to automate the diagnostics process which can bring diagnostics closer to patients’ homes.
更多查看译文
关键词
machine learning,echocardiography,DICOM images,neural networks
AI 理解论文
溯源树
样例
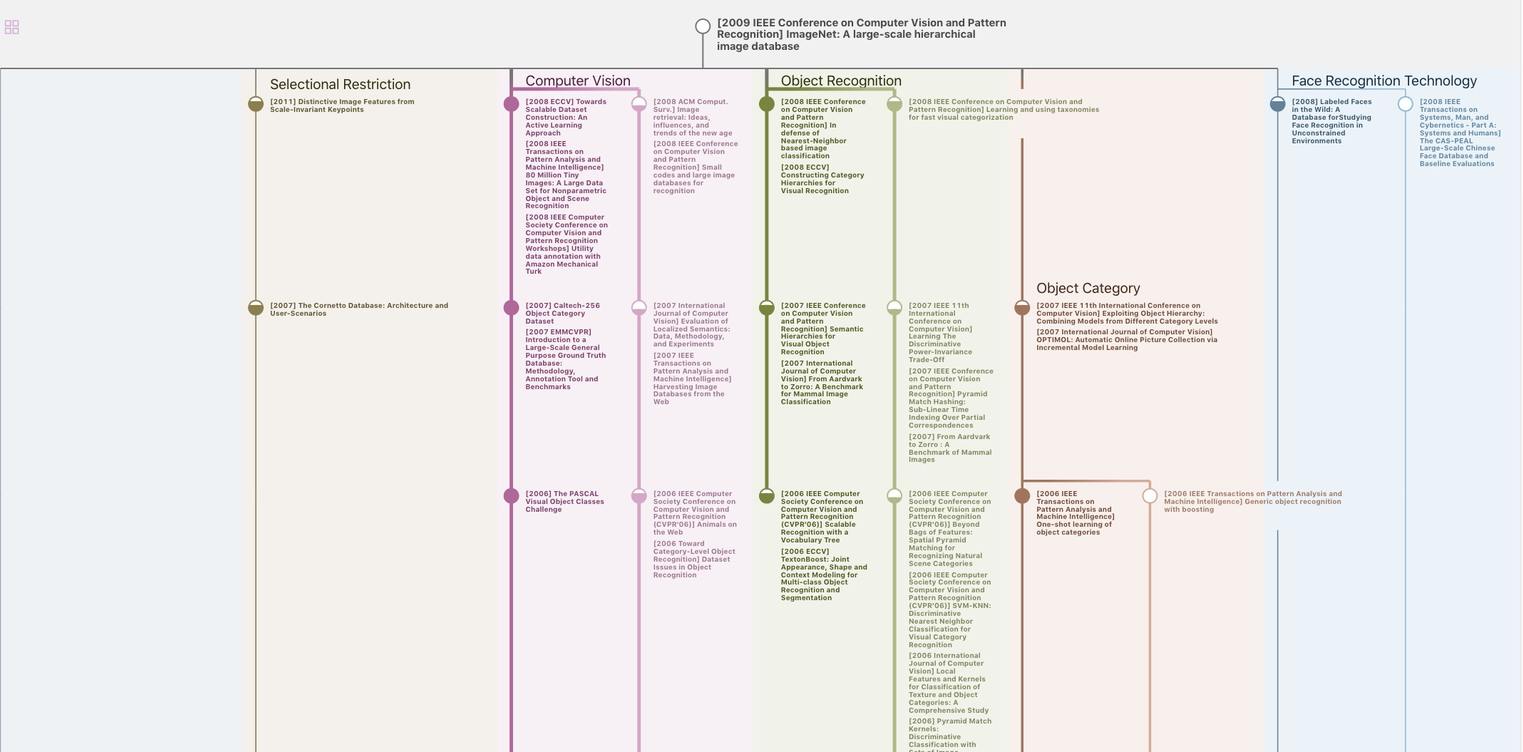
生成溯源树,研究论文发展脉络
Chat Paper
正在生成论文摘要