Malware Detection for Portable Executables Using a Multi-input Transformer-Based Approach.
ICNC(2024)
摘要
Malware is one of the leading cybersecurity challenges, as it disrupts the normal use of devices, causes financial losses, and steals user information. Deep learning-based methods have been increasingly used in the malware analysis field recently. In this work, we propose a novel multi-input Transformer-based approach for detecting malicious Portable Executable (PE) files. The PE raw bytes were partitioned into different byte sequences as multiple inputs in our proposed multi-input framework. This framework is comprised of convolutional neural networks (CNNs) and Transformer networks and is capable of independent learning of each input, thereby enabling a more expressive representation of the data. As a result, it is possible to capture both local spatial and time-series features, resulting in greater data comprehension. Our proposed approach outperforms the two reference methods, a LightGBM and a CNN-based model, as indicated by four metrics: accuracy, recall, precision, and F1 score.
更多查看译文
关键词
malware detection,deep learning,Portable Executable (PE) files,Transformer,multi-input deep learning
AI 理解论文
溯源树
样例
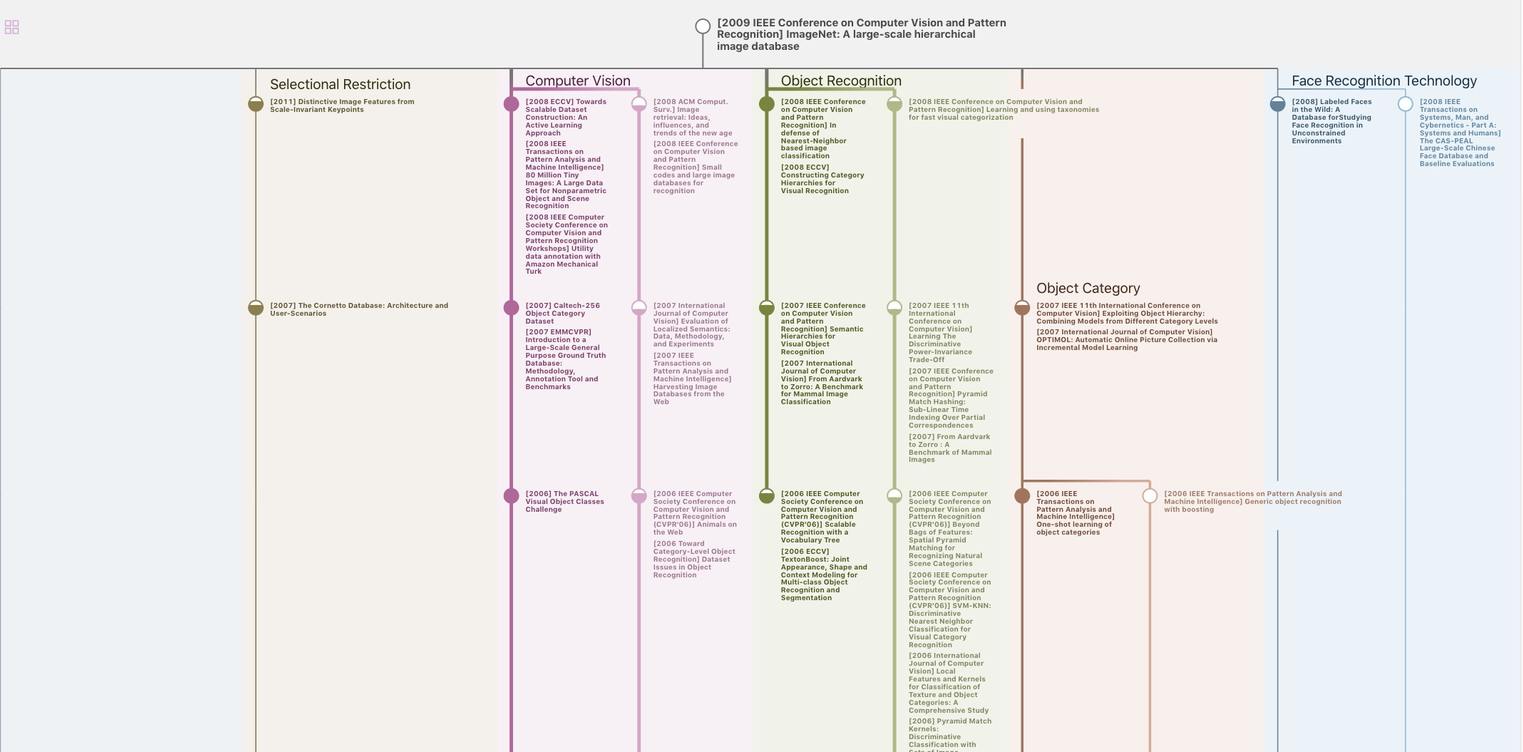
生成溯源树,研究论文发展脉络
Chat Paper
正在生成论文摘要