Privacy-Preserving Characterization and Data Publishing.
ICNC(2024)
Abstract
The increasing interest in collecting and publishing large amounts of data to public has raised significant privacy concerns. Many Privacy-Preserving Data Publishing (PPDP) techniques have been proposed to address these concerns. However, they often lack proper privacy assurance. In this paper, we first present a novel multi-variable privacy characterization and quantification model and analyze both prior and posterior adversarial belief about individual attribute values and the sensitivity of any identifier in privacy characterization using this model. We show that privacy is nearly impossible to be characterized by a single metric. Instead, we propose two metrics to quantify privacy leakage: distribution leakage and entropy leakage. Applying our framework and the proposed metrics, we can reveal limitations in existing PPDP schemes regarding privacy characterization and leakage. This research contributes to a better understanding and evaluation of these techniques, providing a foundation for the design and analysis of PPDP schemes.
MoreTranslated text
Key words
Data privacy,data security,data publishing,privacy quantification,privacy leakage
AI Read Science
Must-Reading Tree
Example
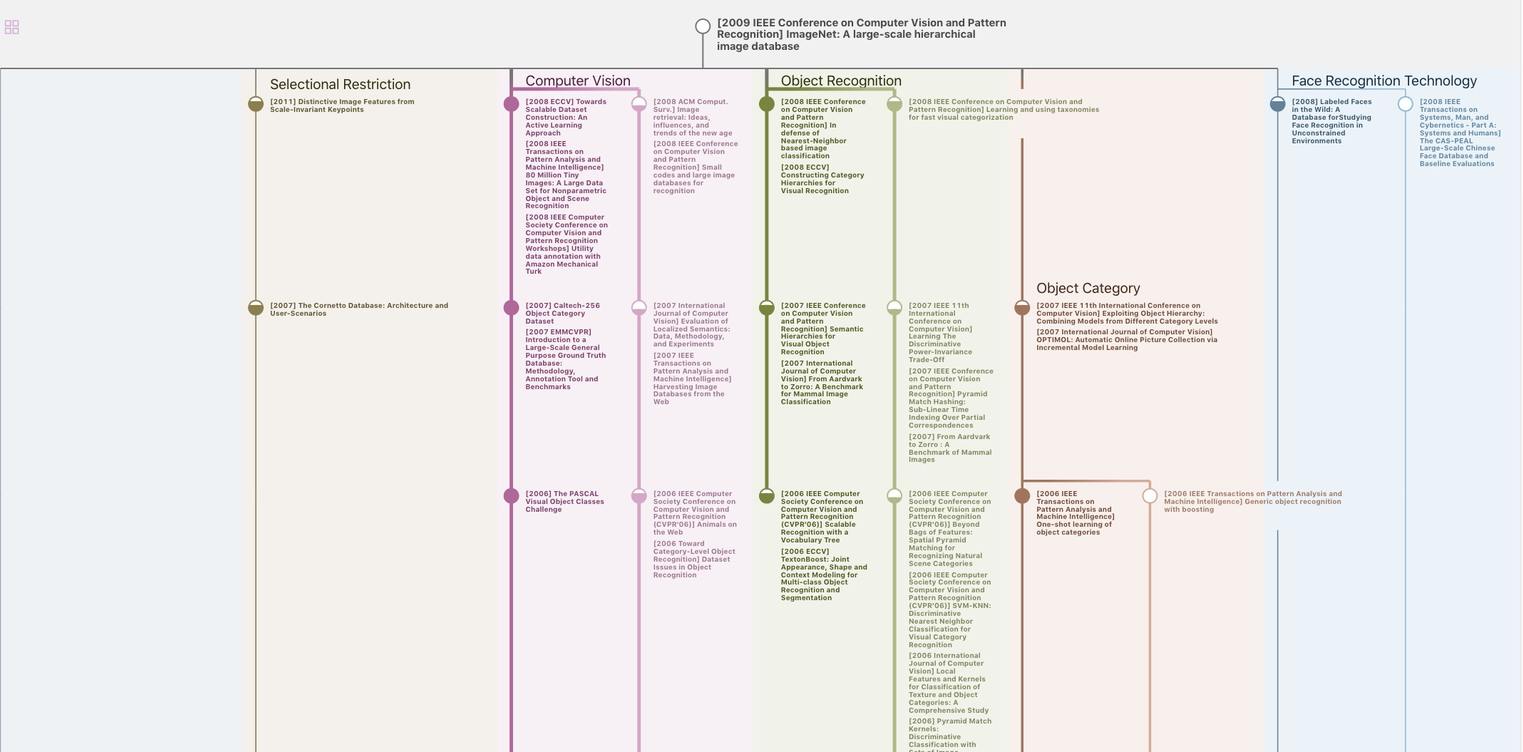
Generate MRT to find the research sequence of this paper
Chat Paper
Summary is being generated by the instructions you defined