To Authenticity, and Beyond! Building Safe and Fair Generative AI Upon the Three Pillars of Provenance.
IEEE computer graphics and applications(2024)
Abstract
Provenance facts, such as who made an image and how, can provide valuable context for users to make trust decisions about visual content. Against a backdrop of inexorable progress in generative AI for computer graphics, over two billion people will vote in public elections this year. Emerging standards and provenance enhancing tools promise to play an important role in fighting fake news and the spread of misinformation. In this article, we contrast three provenance enhancing technologies-metadata, fingerprinting, and watermarking-and discuss how we can build upon the complementary strengths of these three pillars to provide robust trust signals to support stories told by real and generative images. Beyond authenticity, we describe how provenance can also underpin new models for value creation in the age of generative AI. In doing so, we address other risks arising with generative AI such as ensuring training consent, and the proper attribution of credit to creatives who contribute their work to train generative models. We show that provenance may be combined with distributed ledger technology to develop novel solutions for recognizing and rewarding creative endeavor in the age of generative AI.
MoreTranslated text
AI Read Science
Must-Reading Tree
Example
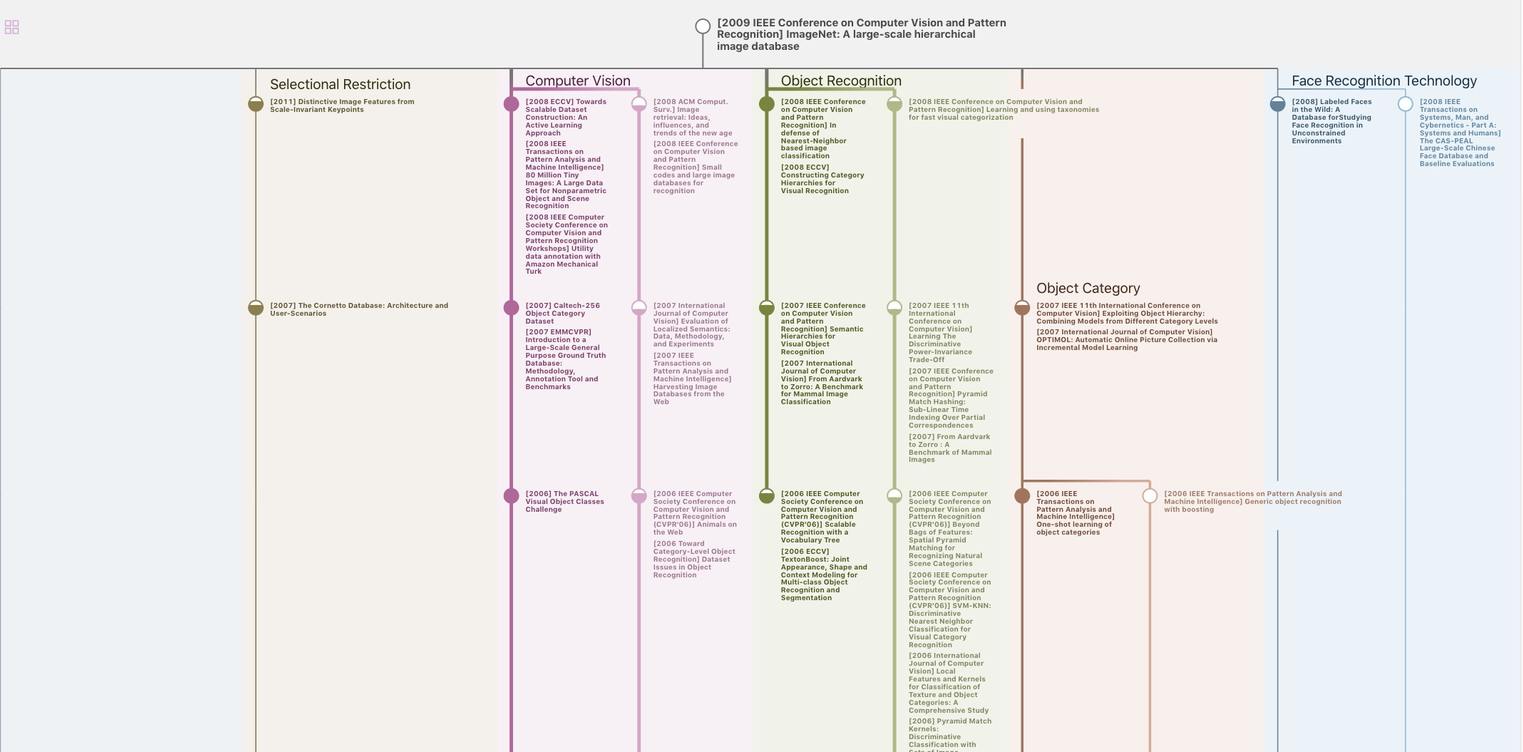
Generate MRT to find the research sequence of this paper
Chat Paper
Summary is being generated by the instructions you defined