Incrementally Adapting Pretrained Model Using Network Prior for Multi-Focus Image Fusion.
IEEE transactions on image processing : a publication of the IEEE Signal Processing Society(2024)
摘要
Multi-focus image fusion can fuse the clear parts of two or more source images captured at the same scene with different focal lengths into an all-in-focus image. On the one hand, previous supervised learning-based multi-focus image fusion methods relying on synthetic datasets have a clear distribution shift with real scenarios. On the other hand, unsupervised learning-based multi-focus image fusion methods can well adapt to the observed images but lack the general knowledge of defocus blur that can be learned from paired data. To avoid the problems of existing methods, this paper presents a novel multi-focus image fusion model by considering both the general knowledge brought by the supervised pretrained backbone and the extrinsic priors optimized on specific testing sample to improve the performance of image fusion. To be specific, the Incremental Network Prior Adaptation (INPA) framework is proposed to incrementally integrate features extracted from the pretrained strong baselines into a tiny prior network (6.9% parameters of the backbone network) to boost the performance for test samples. We evaluate our method on both synthetic and real-world public datasets (Lytro, MFI-WHU, and Real-MFF) and show that our method outperforms existing supervised learning-based methods and unsupervised learning based methods.
更多查看译文
关键词
multi-focus image fusion,model adaptation,deep image prior
AI 理解论文
溯源树
样例
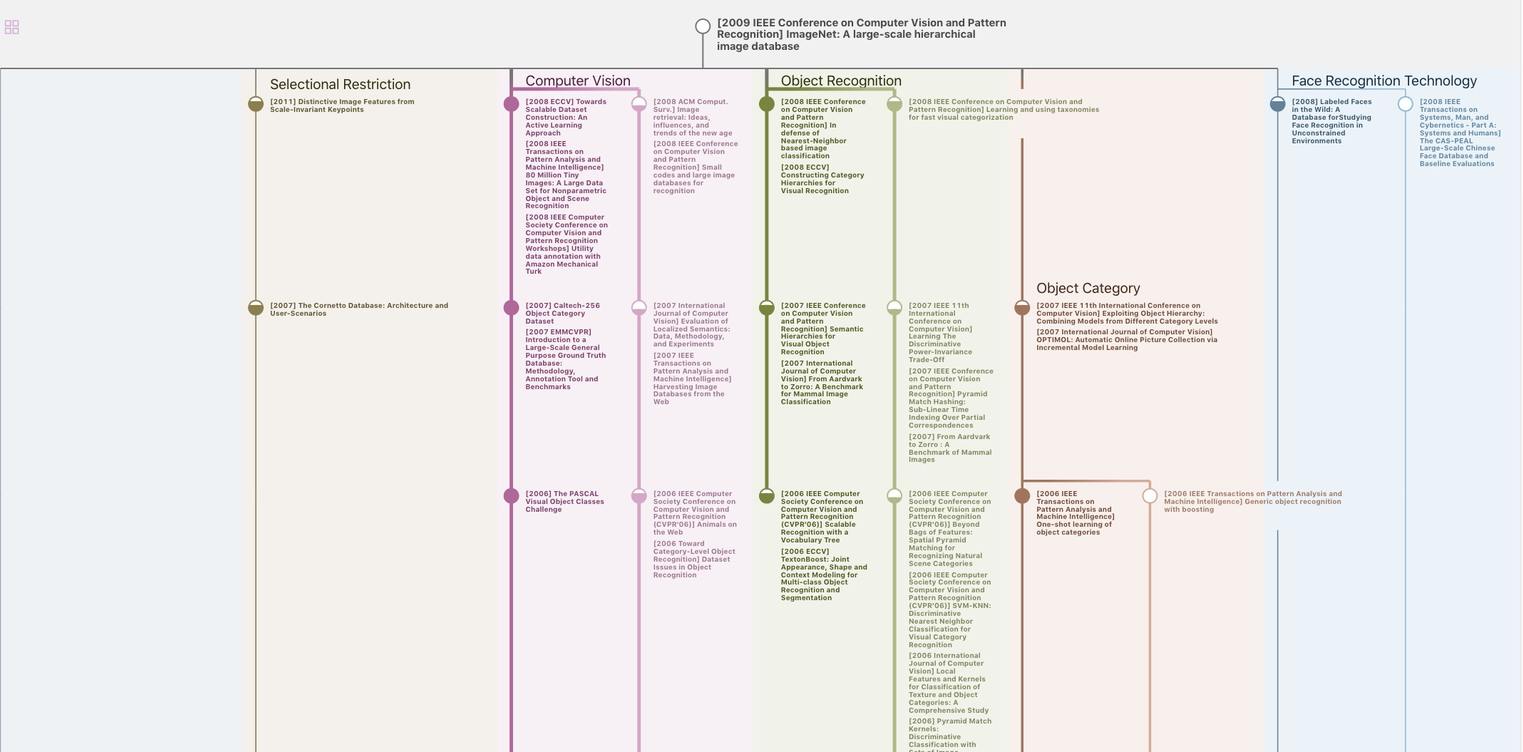
生成溯源树,研究论文发展脉络
Chat Paper
正在生成论文摘要