Federated Graph Anomaly Detection via Contrastive Self-Supervised Learning.
IEEE transactions on neural networks and learning systems(2024)
摘要
Attribute graph anomaly detection aims to identify nodes that significantly deviate from the majority of normal nodes, and has received increasing attention due to the ubiquity and complexity of graph-structured data in various real-world scenarios. However, current mainstream anomaly detection methods are primarily designed for centralized settings, which may pose privacy leakage risks in certain sensitive situations. Although federated graph learning offers a promising solution by enabling collaborative model training in distributed systems while preserving data privacy, a practical challenge arises as each client typically possesses a limited amount of graph data. Consequently, naively applying federated graph learning directly to anomaly detection tasks in distributed environments may lead to suboptimal performance results. We propose a federated graph anomaly detection framework via contrastive self-supervised learning (CSSL) federated CSSL anomaly detection framework (FedCAD) to address these challenges. FedCAD updates anomaly node information between clients via federated learning (FL) interactions. First, FedCAD uses pseudo-label discovery to determine the anomaly node of the client preliminarily. Second, FedCAD employs a local anomaly neighbor embedding aggregation strategy. This strategy enables the current client to aggregate the neighbor embeddings of anomaly nodes from other clients, thereby amplifying the distinction between anomaly nodes and their neighbor nodes. Doing so effectively sharpens the contrast between positive and negative instance pairs within contrastive learning, thus enhancing the efficacy and precision of anomaly detection through such a learning paradigm. Finally, the efficiency of FedCAD is demonstrated by experimental results on four real graph datasets.
更多查看译文
关键词
Anomaly detection,attributed networks,contrastive self-supervised learning (CSSL),federated learning (FL)
AI 理解论文
溯源树
样例
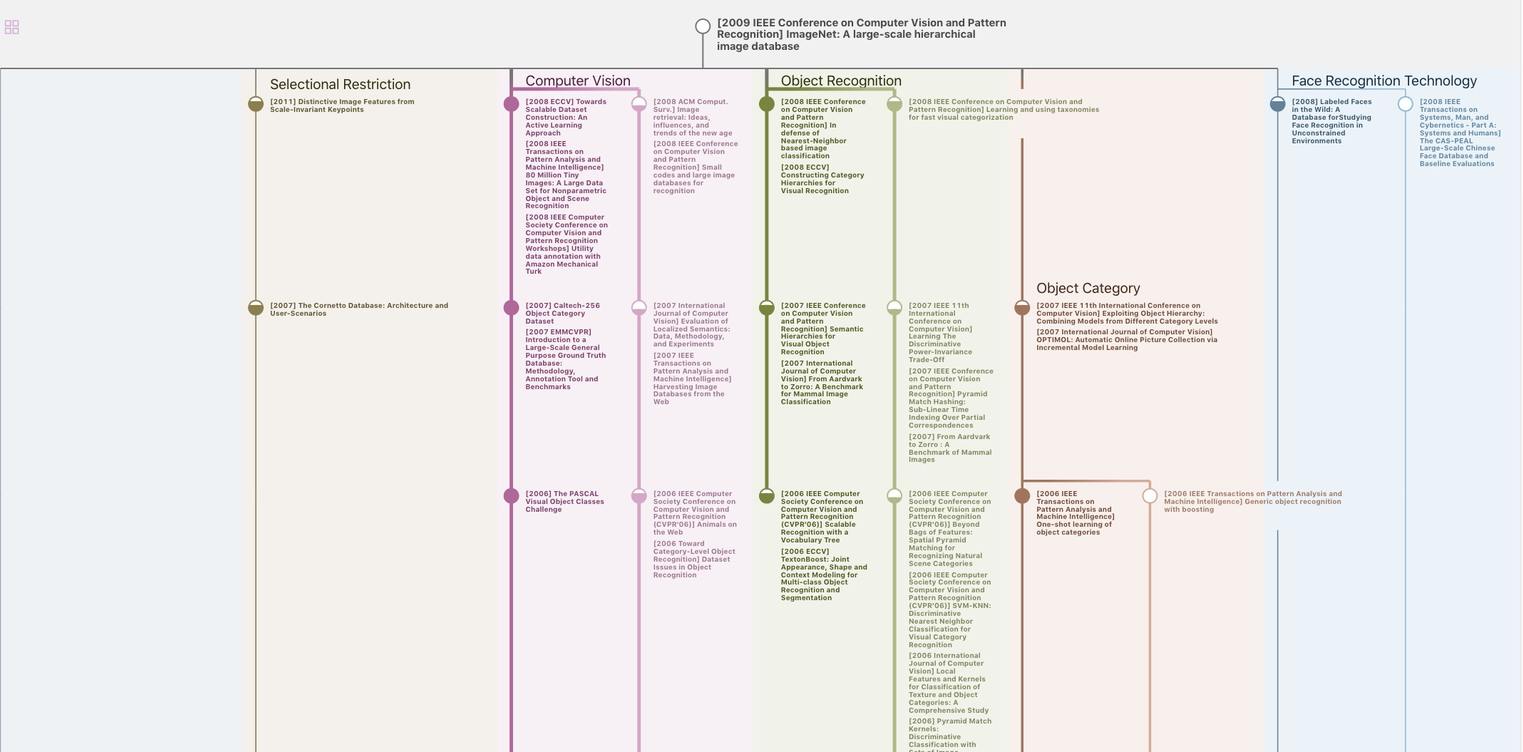
生成溯源树,研究论文发展脉络
Chat Paper
正在生成论文摘要