MR-BEN: A Comprehensive Meta-Reasoning Benchmark for Large Language Models
arxiv(2024)
摘要
Large language models (LLMs) have shown increasing capability in
problem-solving and decision-making, largely based on the step-by-step
chain-of-thought reasoning processes. However, it has been increasingly
challenging to evaluate the reasoning capability of LLMs. Concretely, existing
outcome-based benchmarks begin to saturate and become less sufficient to
monitor the progress. To this end, we present a process-based benchmark MR-BEN
that demands a meta reasoning skill, where LMs are asked to locate and analyse
potential errors in automatically generated reasoning steps. MR-BEN is a
comprehensive benchmark comprising 5,975 questions collected from human
experts, covering various subjects such as physics, chemistry, logic, coding,
and more. Through our designed metrics for assessing meta-reasoning on this
benchmark, we identify interesting limitations and weaknesses of current LLMs
(open-source and closed-source models). For example, open-source models are
seemingly comparable to GPT-4 on outcome-based benchmarks, but they lag far
behind on our benchmark, revealing the underlying reasoning capability gap
between them. Our dataset and codes are available on
https://randolph-zeng.github.io/Mr-Ben.github.io/.
更多查看译文
AI 理解论文
溯源树
样例
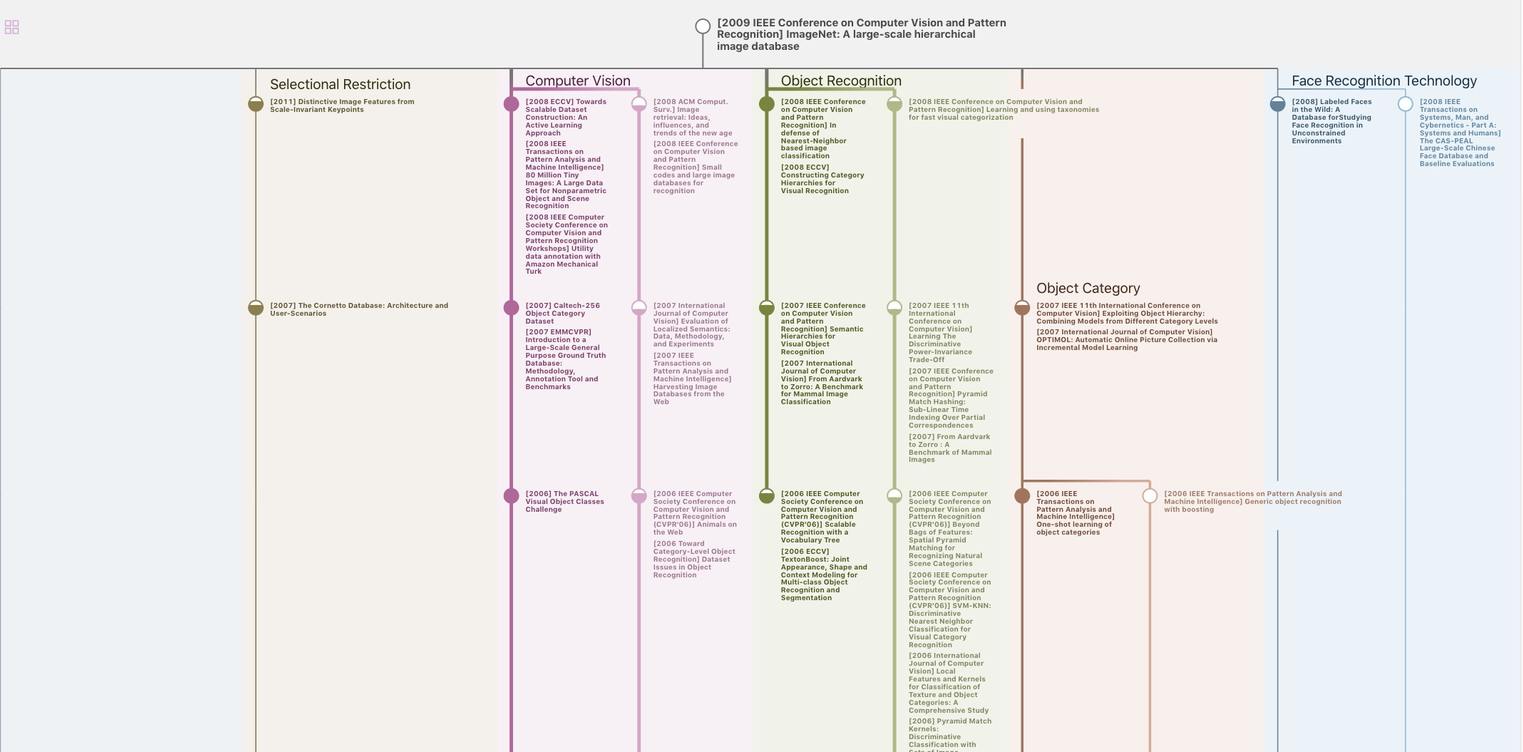
生成溯源树,研究论文发展脉络
Chat Paper
正在生成论文摘要