Factual Confidence of LLMs: on Reliability and Robustness of Current Estimators
arxiv(2024)
Abstract
Large Language Models (LLMs) tend to be unreliable in the factuality of their
answers. To address this problem, NLP researchers have proposed a range of
techniques to estimate LLM's confidence over facts. However, due to the lack of
a systematic comparison, it is not clear how the different methods compare to
one another. To fill this gap, we present a survey and empirical comparison of
estimators of factual confidence. We define an experimental framework allowing
for fair comparison, covering both fact-verification and question answering.
Our experiments across a series of LLMs indicate that trained hidden-state
probes provide the most reliable confidence estimates, albeit at the expense of
requiring access to weights and training data. We also conduct a deeper
assessment of factual confidence by measuring the consistency of model behavior
under meaning-preserving variations in the input. We find that the confidence
of LLMs is often unstable across semantically equivalent inputs, suggesting
that there is much room for improvement of the stability of models' parametric
knowledge. Our code is available at
(https://github.com/amazon-science/factual-confidence-of-llms).
MoreTranslated text
AI Read Science
Must-Reading Tree
Example
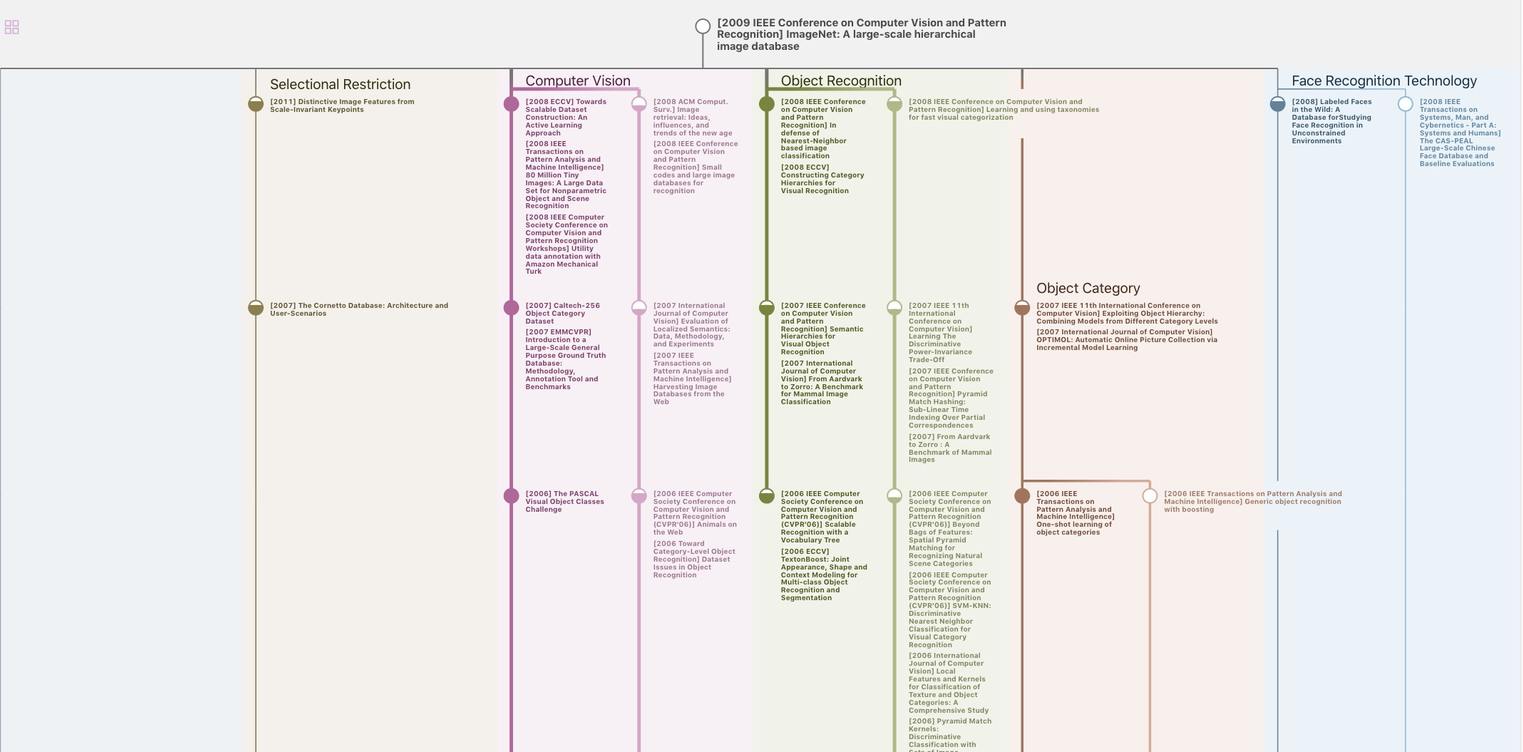
Generate MRT to find the research sequence of this paper
Chat Paper
Summary is being generated by the instructions you defined