iDetector: A Novel Real-Time Intrusion Detection Solution for IoT Networks
IEEE Internet of Things Journal(2024)
Abstract
The rapid proliferation of IoT devices has brought about unprecedented convenience to people’s daily lives. However, this growth has also created opportunities for hackers to launch large-scale botnet attacks using these devices. As a result, it is critical to deploy real-time traffic classifiers on edge gateways to detect network intrusions and improve near-source protection capabilities. To this end, we propose iDetector, a novel realtime intrusion detection solution for IoT networks that is simple in structure and easy to reproduce. iDetector samples network conversations in real-time using a sliding sampling window and generates traffic samples that integrate multiple features. This allows the samples to accurately capture the patterns of each type of traffic. We propose the Non-linear Feature Transformation (NFT) algorithm based on the prior distribution of traffic features to increase the information entropy of the samples and thereby improve the classification performance. To enable deployment on edge gateways, we propose EdgeNet, a lightweight deep neural network model that utilizes Depthwise Separable Convolution and self-attention mechanism to enhance classification performance while reducing the number of model parameters. Experimental evaluations show that our solution outperforms state-of-the-art deep learning-based solutions in terms of classification performance and has faster classification speed on resource-constrained edge gateways.
MoreTranslated text
Key words
Traffic classification,botnet,internet of things
AI Read Science
Must-Reading Tree
Example
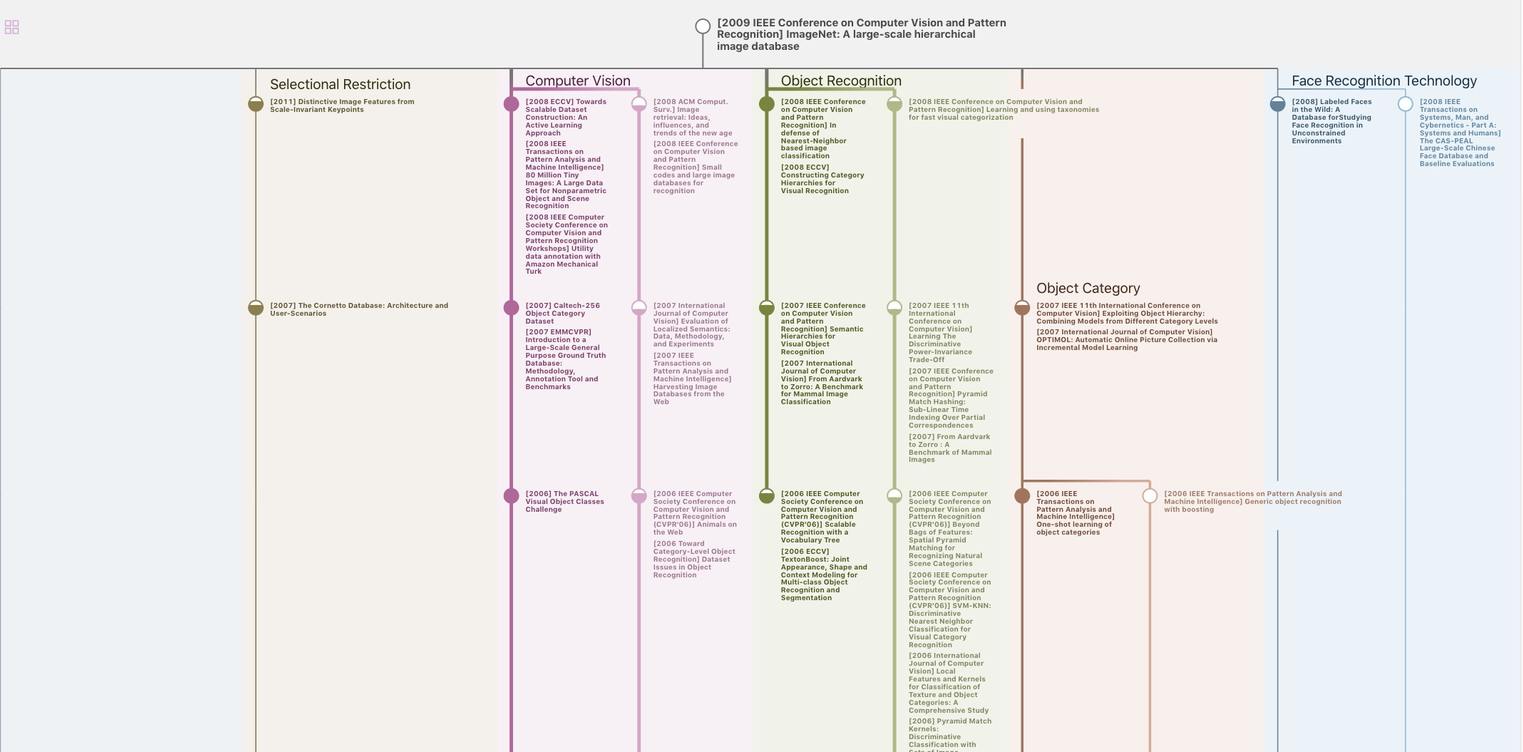
Generate MRT to find the research sequence of this paper
Chat Paper
Summary is being generated by the instructions you defined