VMD-IDBO-LSTM: Short-Term Photovoltaic Power Prediction Models Based on Similar Day Clustering
2024 7th International Conference on Advanced Algorithms and Control Engineering (ICAACE)(2024)
Abstract
In the context of the current energy crisis and increased environmental pollution, clean energy has received widespread attention, and solar photovoltaic power generation has been popularized and developed rapidly. Aiming at the problems of always uncertainty and low prediction accuracy of PV power generation, a short-term PV power prediction model based on similar day clustering and variational modal decomposition (VMD), improved dung beetle algorithm (IDBO) optimized long-short-term memory network (LSTM) is proposed. Firstly, K-means clustering algorithm is used to classify the original data into similar daily samples of three different weather types, namely sunny, cloudy and rainy days, and VMD is used to decompose the original power data to obtain a number of different subsequences; secondly, an LSTM prediction model is established for the different subsequences, and IDBO is used to optimize the parameters of the LSTM network; Finally, the predictions of each subsequence are superimposed and reconstructed to obtain the final PV power prediction results. The simulation analysis verifies that the prediction accuracy of the VMD-IDBO-LSTM model is better than other models under different weather types, and it can fit the actual PV power curve better, which verifies the validity of the model.
MoreTranslated text
Key words
photovoltaic power generation,K-means cluster,variational mode decomposition,improved dung beetle optimizer,long short-term memory
AI Read Science
Must-Reading Tree
Example
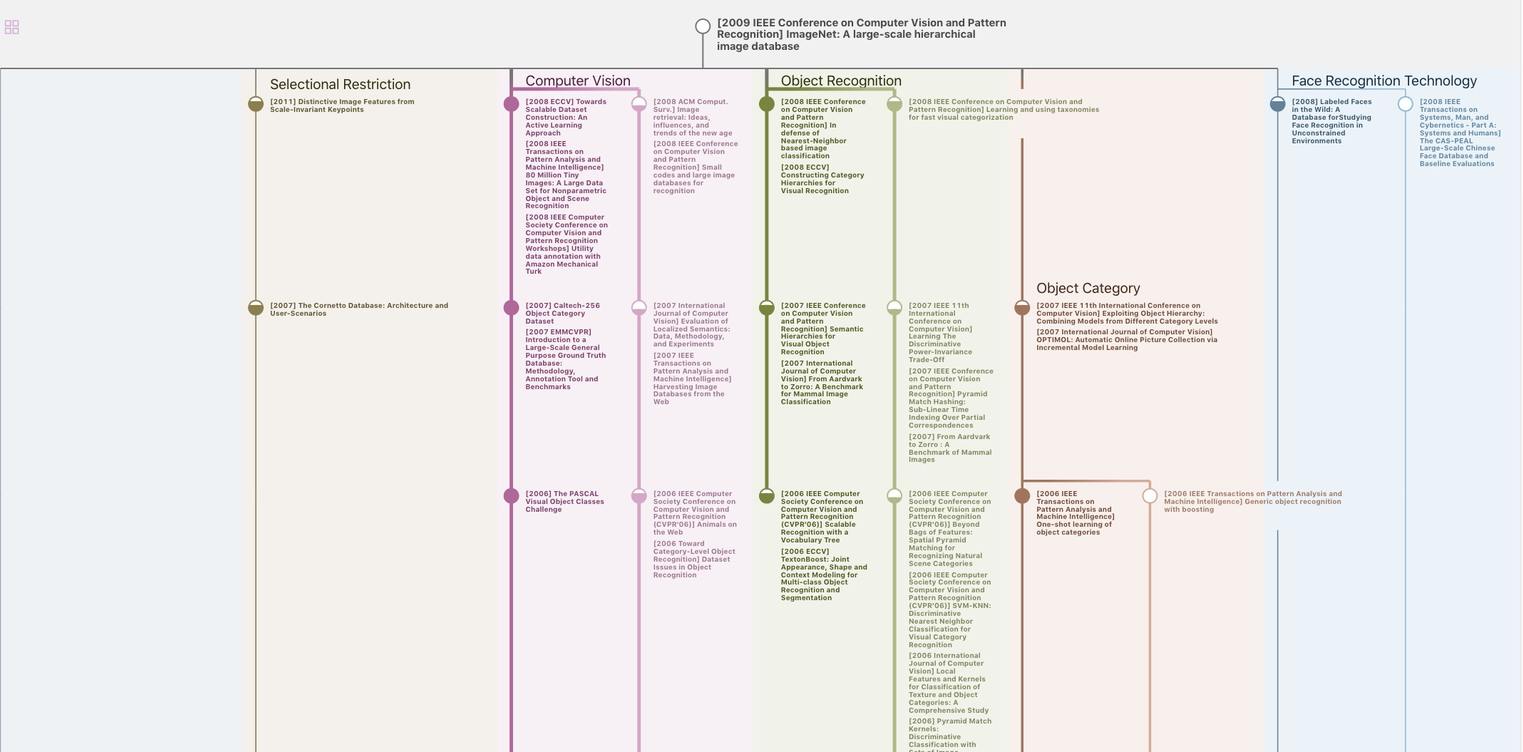
Generate MRT to find the research sequence of this paper
Chat Paper
Summary is being generated by the instructions you defined