Road Tunnels Defects Detection and Classification using Deep Learning.
International Conference on Automation, Robotics and Applications(2024)
Abstract
A two-step approach is presented in this paper for defect detection and classification in tunnel inspection images using data-driven technologies. In the first step, a denoising auto encoder based on convolutional neural network architecture is employed as an unsupervised defect detector. This detector identifies potential defective regions within a vast collection of inspection images. The annotation process is streamlined by the defect detector, precisely pinpointing defective areas and reducing the overall workload for annotators. The second step involves an ensemble defect classifier that combines a large vision transformer and a U-Net convolutional model to classify the extracted defective regions. The proposed methodology's effectiveness is demonstrated through its successful implementation on a robotic platform for automated visual inspection in the Egnatia Motorway in Greece. This implementation is part of the PILOTING project supported by the European Union's Horizon 2020 innovation action program.
MoreTranslated text
Key words
tunnel maintenance,defect detection,denoising auto encoder,defect classification,U-Net,Swin transformer
AI Read Science
Must-Reading Tree
Example
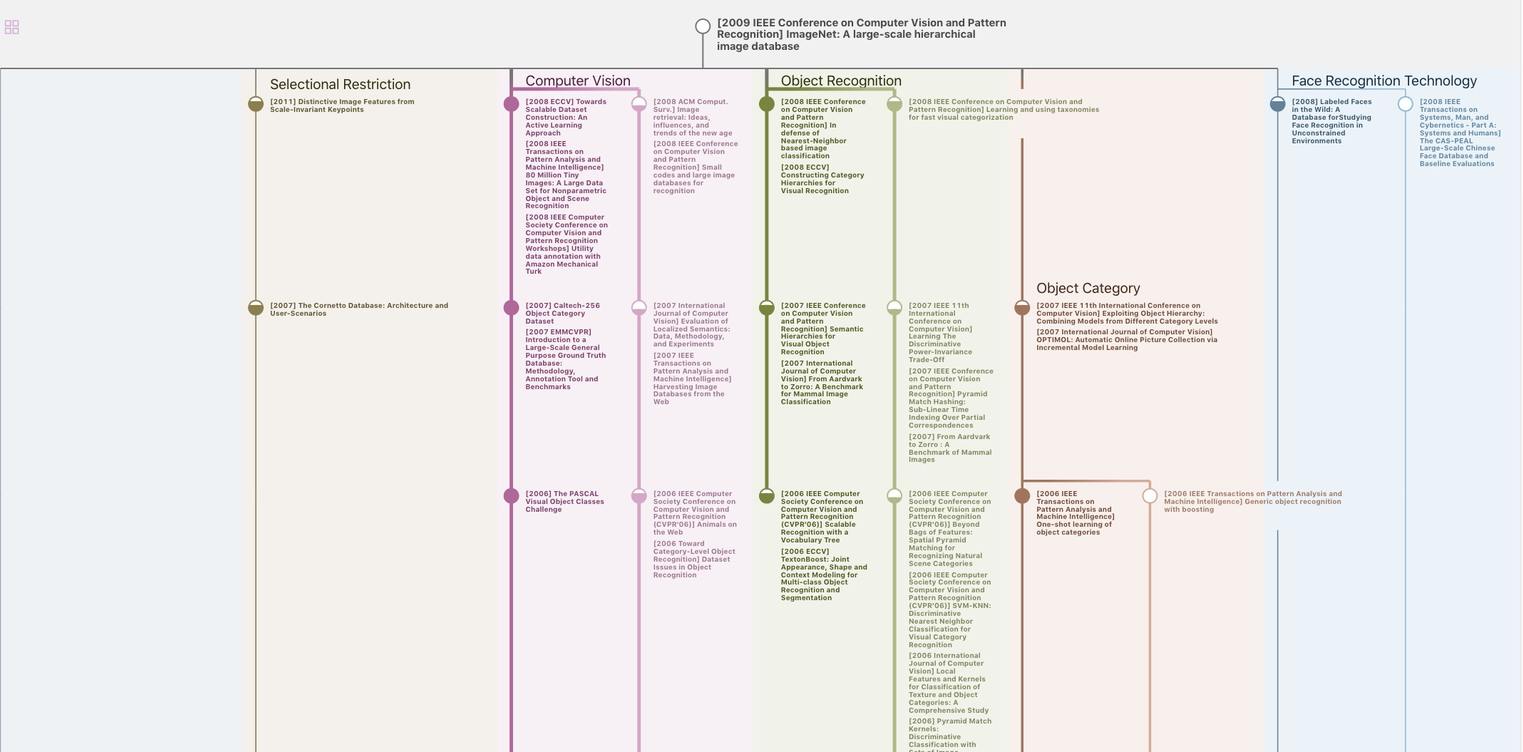
Generate MRT to find the research sequence of this paper
Chat Paper
Summary is being generated by the instructions you defined