Data Petri Nets meet Probabilistic Programming (Extended version)
arxiv(2024)
摘要
Probabilistic programming (PP) is a programming paradigm that allows for
writing statistical models like ordinary programs, performing simulations by
running those programs, and analyzing and refining their statistical behavior
using powerful inference engines. This paper takes a step towards leveraging PP
for reasoning about data-aware processes. To this end, we present a systematic
translation of Data Petri Nets (DPNs) into a model written in a PP language
whose features are supported by most PP systems. We show that our translation
is sound and provides statistical guarantees for simulating DPNs. Furthermore,
we discuss how PP can be used for process mining tasks and report on a
prototype implementation of our translation. We also discuss further analysis
scenarios that could be easily approached based on the proposed translation and
available PP tools.
更多查看译文
AI 理解论文
溯源树
样例
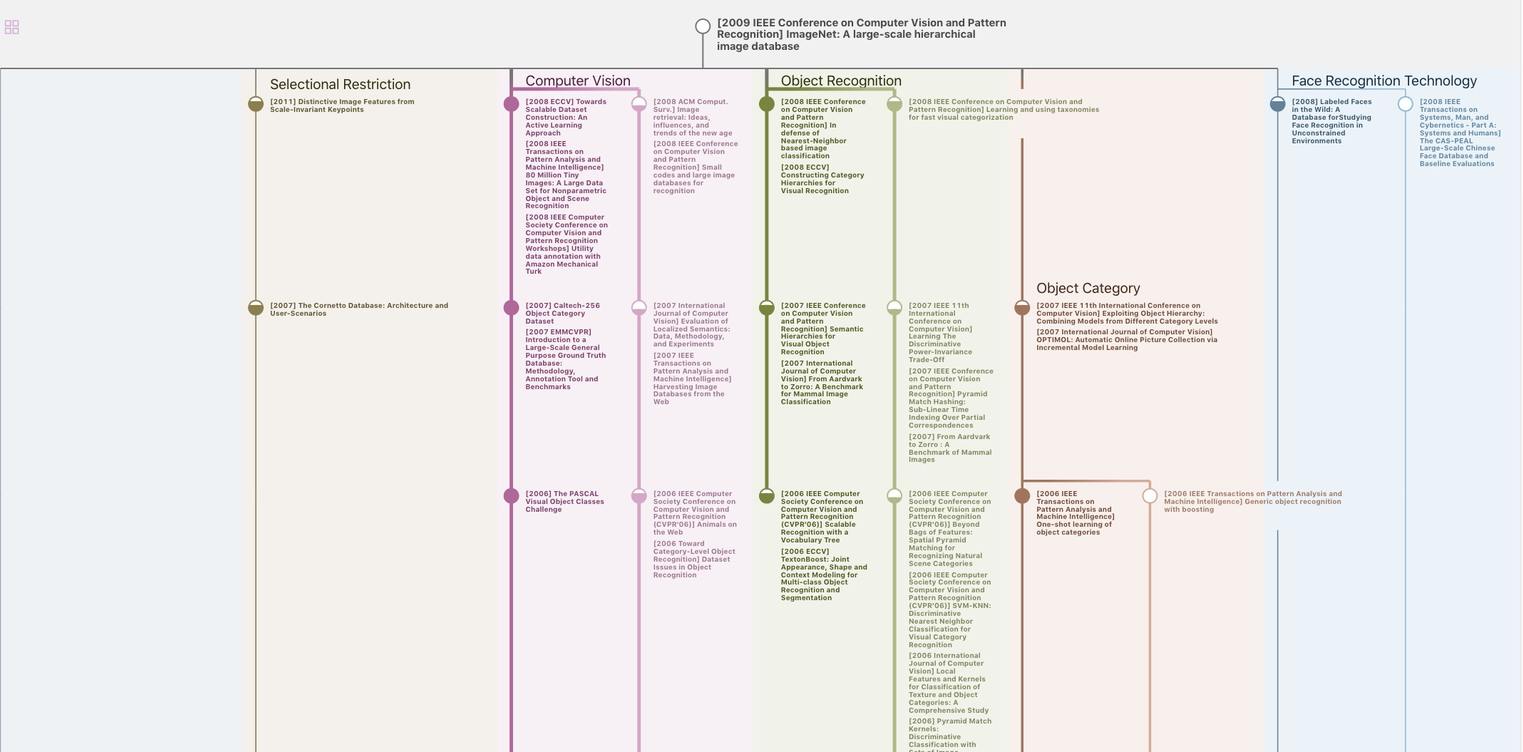
生成溯源树,研究论文发展脉络
Chat Paper
正在生成论文摘要