Evaluating machine learning prediction techniques and their impact on proactive resource provisioning for cloud environments
The Journal of Supercomputing(2024)
摘要
Cloud computing has several benefits over traditional systems, such as scalability and high availability. However, these benefits, to be eventuated, require efforts in the area of resource provisioning and scaling, to match resources to current and future demand, and this is not always trivial to achieve. Since workload may fluctuate substantially in cloud environments, over-provisioning is a common practice to avoid abrupt quality of service (QoS) drops that may result in service level agreement (SLA) violations, but at the price of increased provisioning costs and energy consumption. Workload prediction is one of the strategies by which efficiency and operational cost of a clouds can be improved. Therefore, in this paper, we show the potential benefits of a proactive resource provisioning scheme augmented by three of the most promising machine learning prediction techniques in this context, namely ARIMA, MLP, and GRU, that are known to be able to cope with the dynamic behavior of our target applications. We analyze the trade-off between resource consumption and quality of service using SLA violations in web workloads, considering real case provisioning requirements and constraints, extensively simulating and analyzing the impact of prediction and scaling intervals, and publishing all used tools and datasets to allow reproducibility. Simulation experiments with a proactive approach are executed using real traces from NASA and Wikipedia workloads and achieved a reduction of 40
更多查看译文
关键词
Cloud computing,Workload prediction,Machine learning,Resource management
AI 理解论文
溯源树
样例
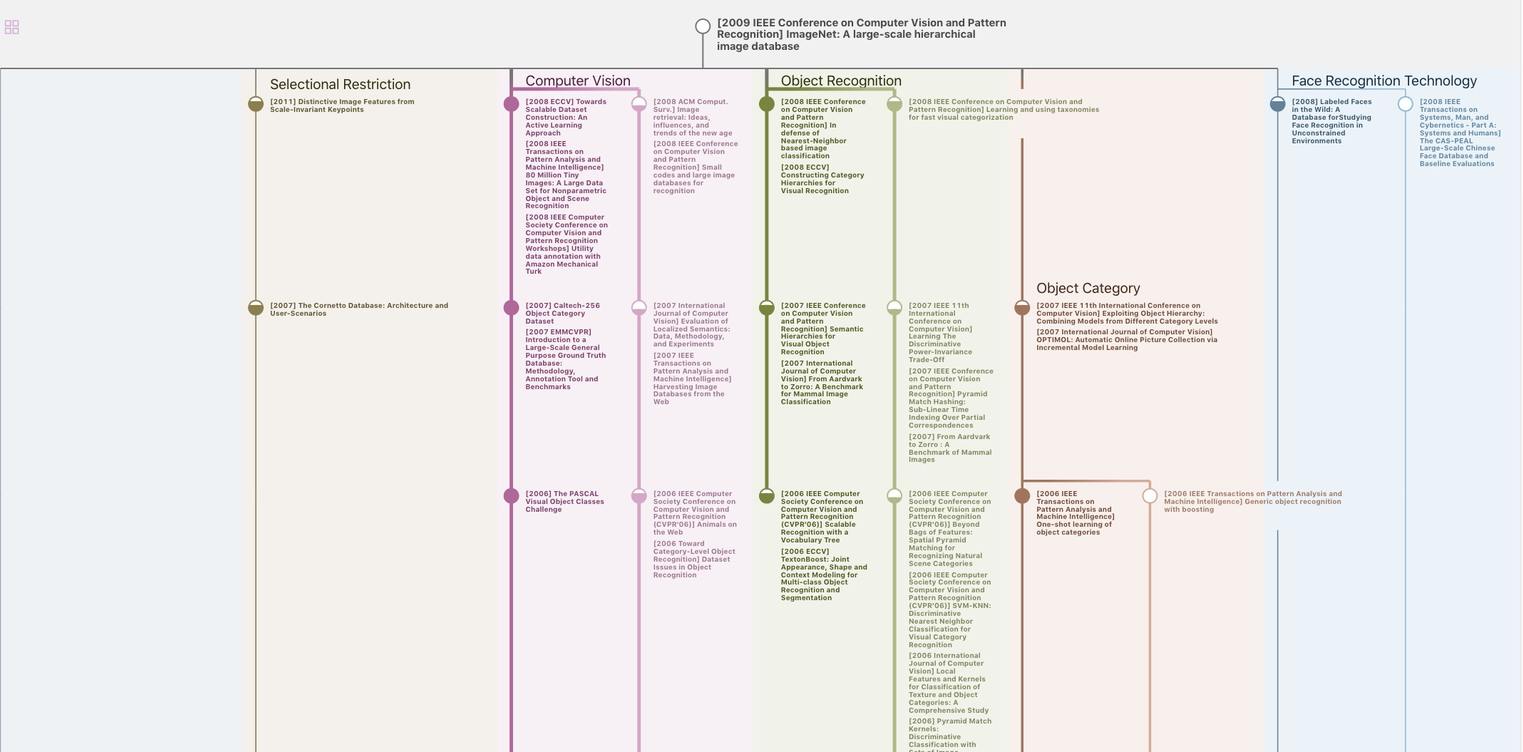
生成溯源树,研究论文发展脉络
Chat Paper
正在生成论文摘要