Imagination Policy: Using Generative Point Cloud Models for Learning Manipulation Policies
arxiv(2024)
摘要
Humans can imagine goal states during planning and perform actions to match
those goals. In this work, we propose Imagination Policy, a novel multi-task
key-frame policy network for solving high-precision pick and place tasks.
Instead of learning actions directly, Imagination Policy generates point clouds
to imagine desired states which are then translated to actions using rigid
action estimation. This transforms action inference into a local generative
task. We leverage pick and place symmetries underlying the tasks in the
generation process and achieve extremely high sample efficiency and
generalizability to unseen configurations. Finally, we demonstrate
state-of-the-art performance across various tasks on the RLbench benchmark
compared with several strong baselines.
更多查看译文
AI 理解论文
溯源树
样例
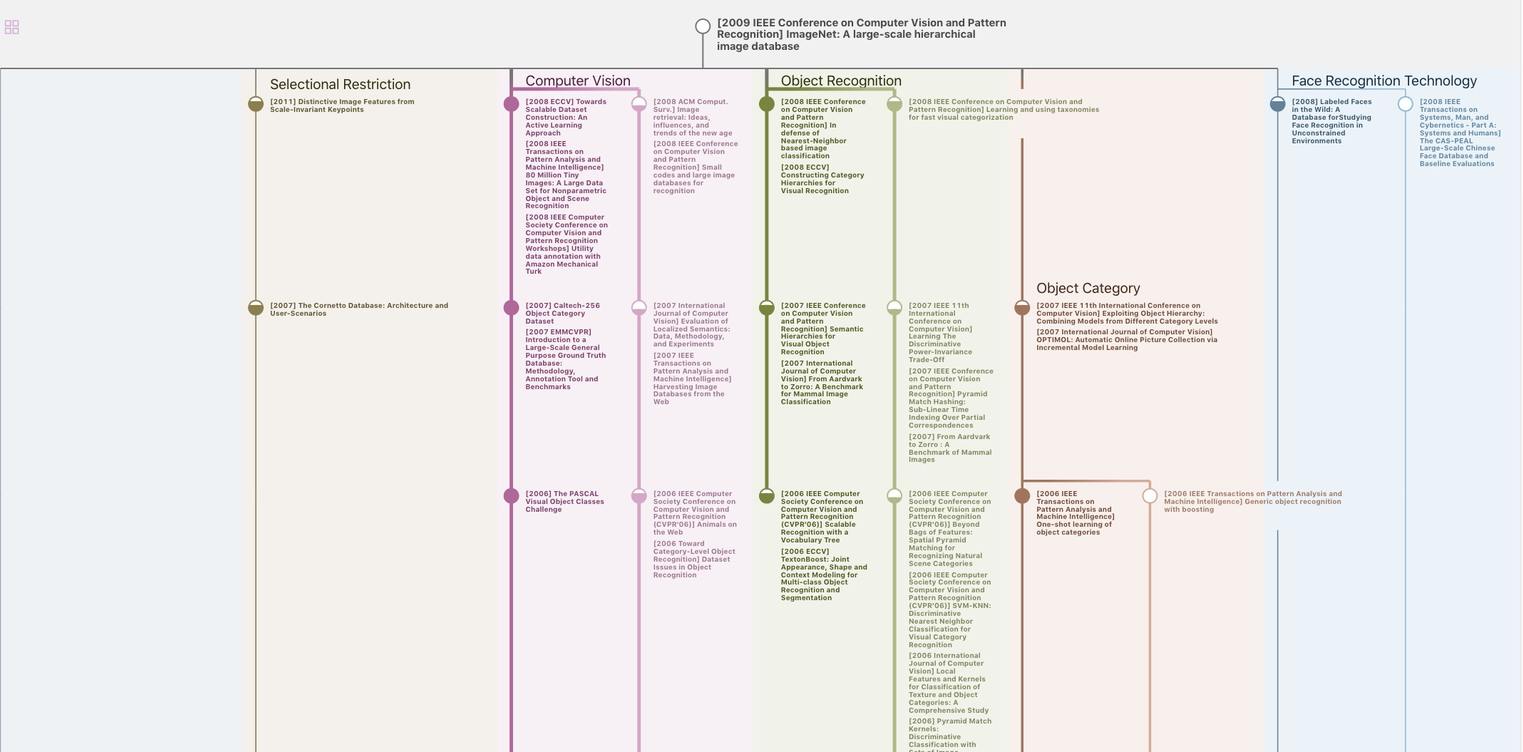
生成溯源树,研究论文发展脉络
Chat Paper
正在生成论文摘要