MFC-Bench: Benchmarking Multimodal Fact-Checking with Large Vision-Language Models
CoRR(2024)
摘要
Large vision-language models (LVLMs) have significantly improved multimodal
reasoning tasks, such as visual question answering and image captioning. These
models embed multimodal facts within their parameters, rather than relying on
external knowledge bases to store factual information explicitly. However, the
content discerned by LVLMs may deviate from actual facts due to inherent bias
or incorrect inference. To address this issue, we introduce MFC-Bench, a
rigorous and comprehensive benchmark designed to evaluate the factual accuracy
of LVLMs across three tasks: Manipulation, Out-of-Context, and Veracity
Classification. Through our evaluation on MFC-Bench, we benchmarked 12 diverse
and representative LVLMs, uncovering that current models still fall short in
multimodal fact-checking and demonstrate insensitivity to various forms of
manipulated content. We hope that MFC-Bench could raise attention to the
trustworthy artificial intelligence potentially assisted by LVLMs in the
future. The MFC-Bench and accompanying resources are publicly accessible at
https://github.com/wskbest/MFC-Bench, contributing to ongoing research in the
multimodal fact-checking field.
更多查看译文
AI 理解论文
溯源树
样例
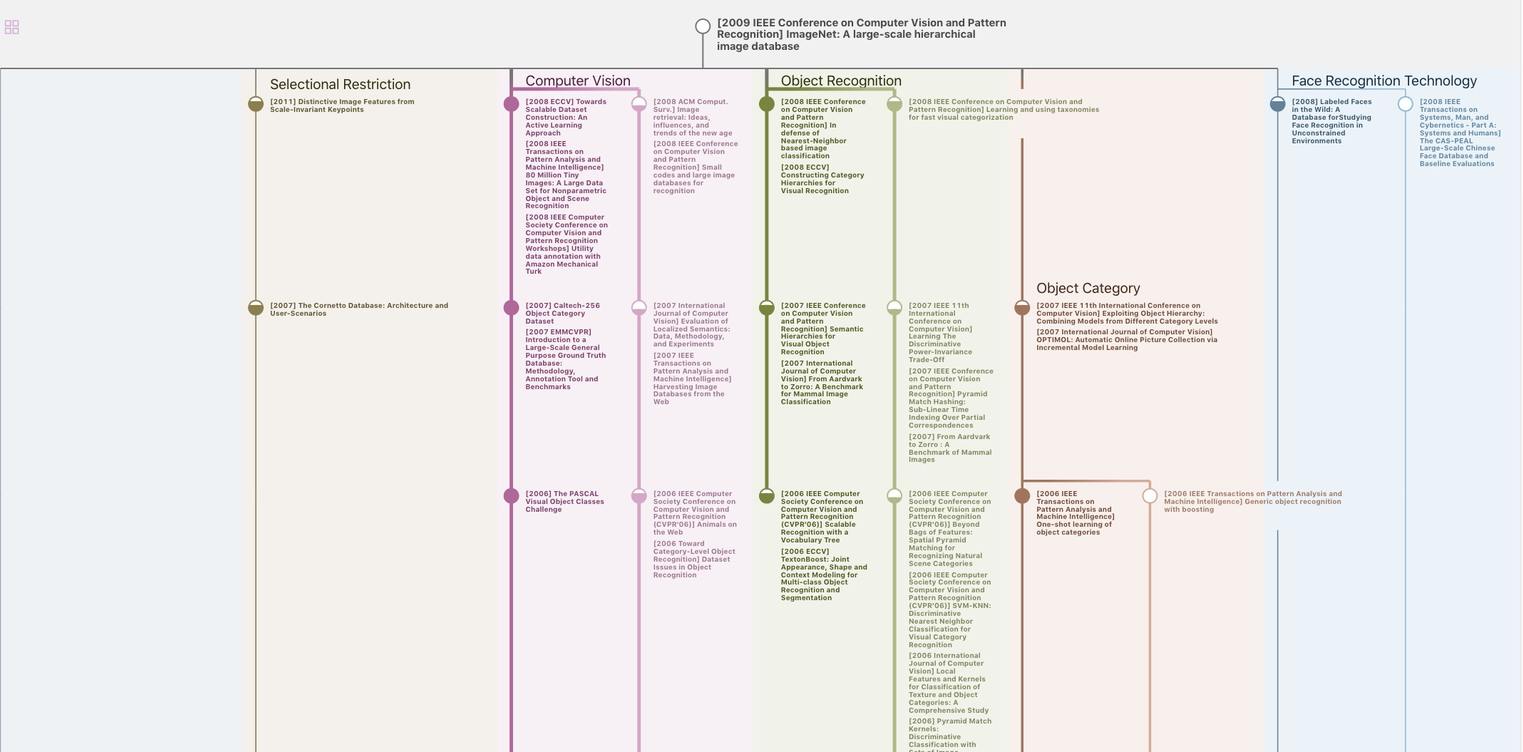
生成溯源树,研究论文发展脉络
Chat Paper
正在生成论文摘要