Self-training Large Language Models through Knowledge Detection
CoRR(2024)
Abstract
Large language models (LLMs) often necessitate extensive labeled datasets and
training compute to achieve impressive performance across downstream tasks.
This paper explores a self-training paradigm, where the LLM autonomously
curates its own labels and selectively trains on unknown data samples
identified through a reference-free consistency method. Empirical evaluations
demonstrate significant improvements in reducing hallucination in generation
across multiple subjects. Furthermore, the selective training framework
mitigates catastrophic forgetting in out-of-distribution benchmarks, addressing
a critical limitation in training LLMs. Our findings suggest that such an
approach can substantially reduce the dependency on large labeled datasets,
paving the way for more scalable and cost-effective language model training.
MoreTranslated text
AI Read Science
Must-Reading Tree
Example
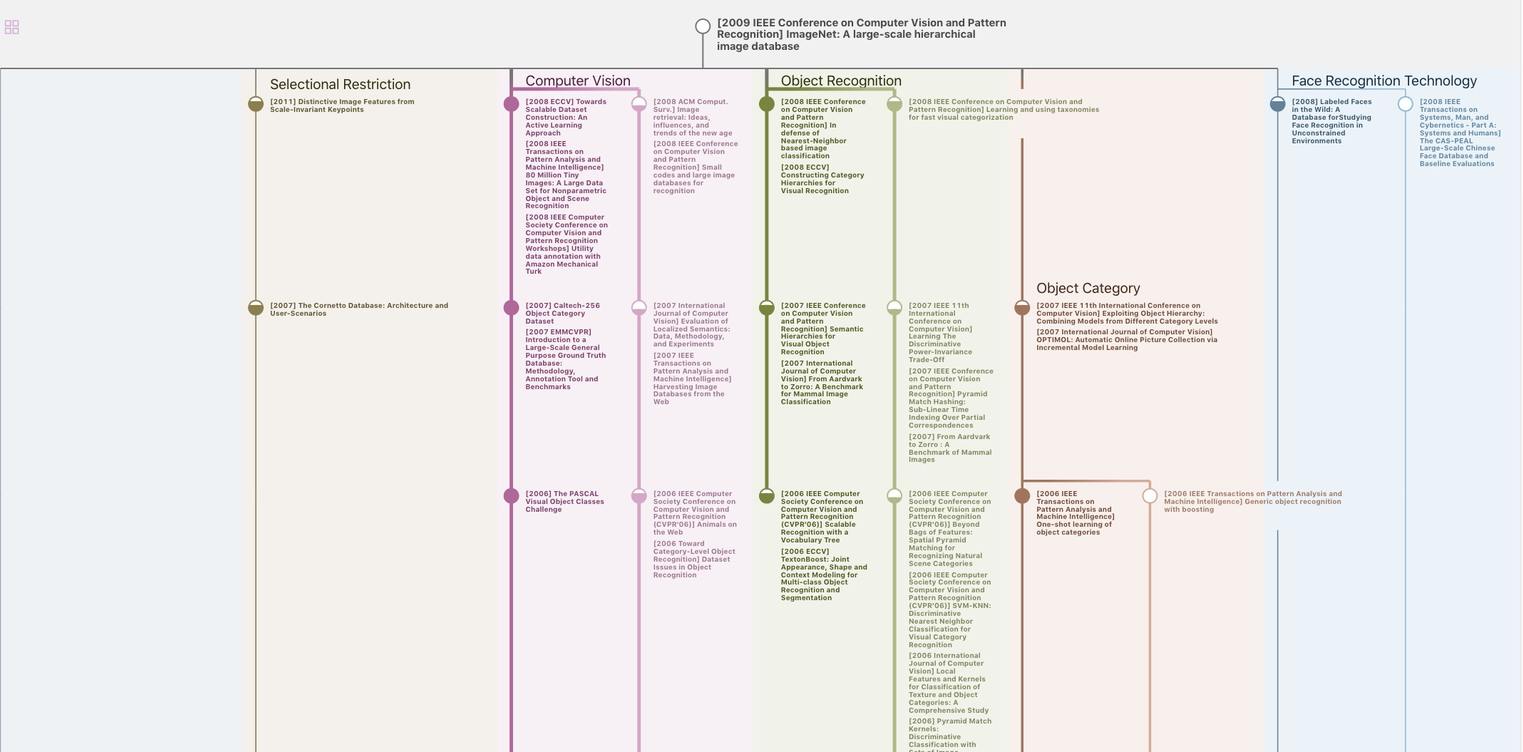
Generate MRT to find the research sequence of this paper
Chat Paper
Summary is being generated by the instructions you defined