Occam's Razor for Self Supervised Learning: What is Sufficient to Learn Good Representations?
CoRR(2024)
Abstract
Deep Learning is often depicted as a trio of data-architecture-loss. Yet,
recent Self Supervised Learning (SSL) solutions have introduced numerous
additional design choices, e.g., a projector network, positive views, or
teacher-student networks. These additions pose two challenges. First, they
limit the impact of theoretical studies that often fail to incorporate all
those intertwined designs. Second, they slow-down the deployment of SSL methods
to new domains as numerous hyper-parameters need to be carefully tuned. In this
study, we bring forward the surprising observation that–at least for
pretraining datasets of up to a few hundred thousands samples–the additional
designs introduced by SSL do not contribute to the quality of the learned
representations. That finding not only provides legitimacy to existing
theoretical studies, but also simplifies the practitioner's path to SSL
deployment in numerous small and medium scale settings. Our finding answers a
long-lasting question: the often-experienced sensitivity to training settings
and hyper-parameters encountered in SSL come from their design, rather than the
absence of supervised guidance.
MoreTranslated text
AI Read Science
Must-Reading Tree
Example
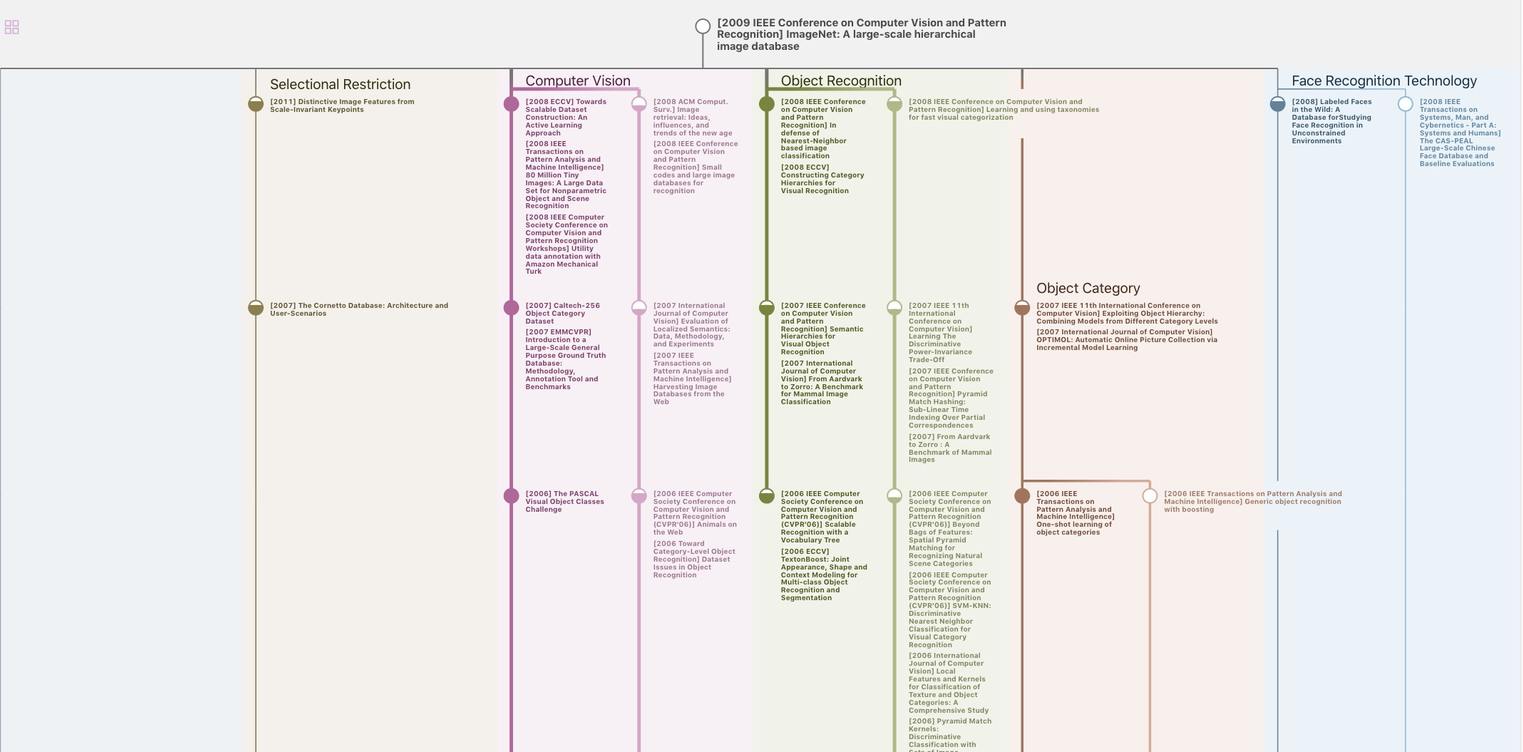
Generate MRT to find the research sequence of this paper
Chat Paper
Summary is being generated by the instructions you defined