Advancing High Resolution Vision-Language Models in Biomedicine
arxiv(2024)
摘要
Multi-modal learning has significantly advanced generative AI, especially in
vision-language modeling. Innovations like GPT-4V and open-source projects such
as LLaVA have enabled robust conversational agents capable of zero-shot task
completions. However, applying these technologies in the biomedical field
presents unique challenges. Recent initiatives like LLaVA-Med have started to
adapt instruction-tuning for biomedical contexts using large datasets such as
PMC-15M. Our research offers three key contributions: (i) we present a new
instruct dataset enriched with medical image-text pairs from Claude3-Opus and
LLaMA3 70B, (ii) we propose a novel image encoding strategy using hierarchical
representations to improve fine-grained biomedical visual comprehension, and
(iii) we develop the Llama3-Med model, which achieves state-of-the-art
zero-shot performance on biomedical visual question answering benchmarks, with
an average performance improvement of over 10
These advancements provide more accurate and reliable tools for medical
professionals, bridging gaps in current multi-modal conversational assistants
and promoting further innovations in medical AI.
更多查看译文
AI 理解论文
溯源树
样例
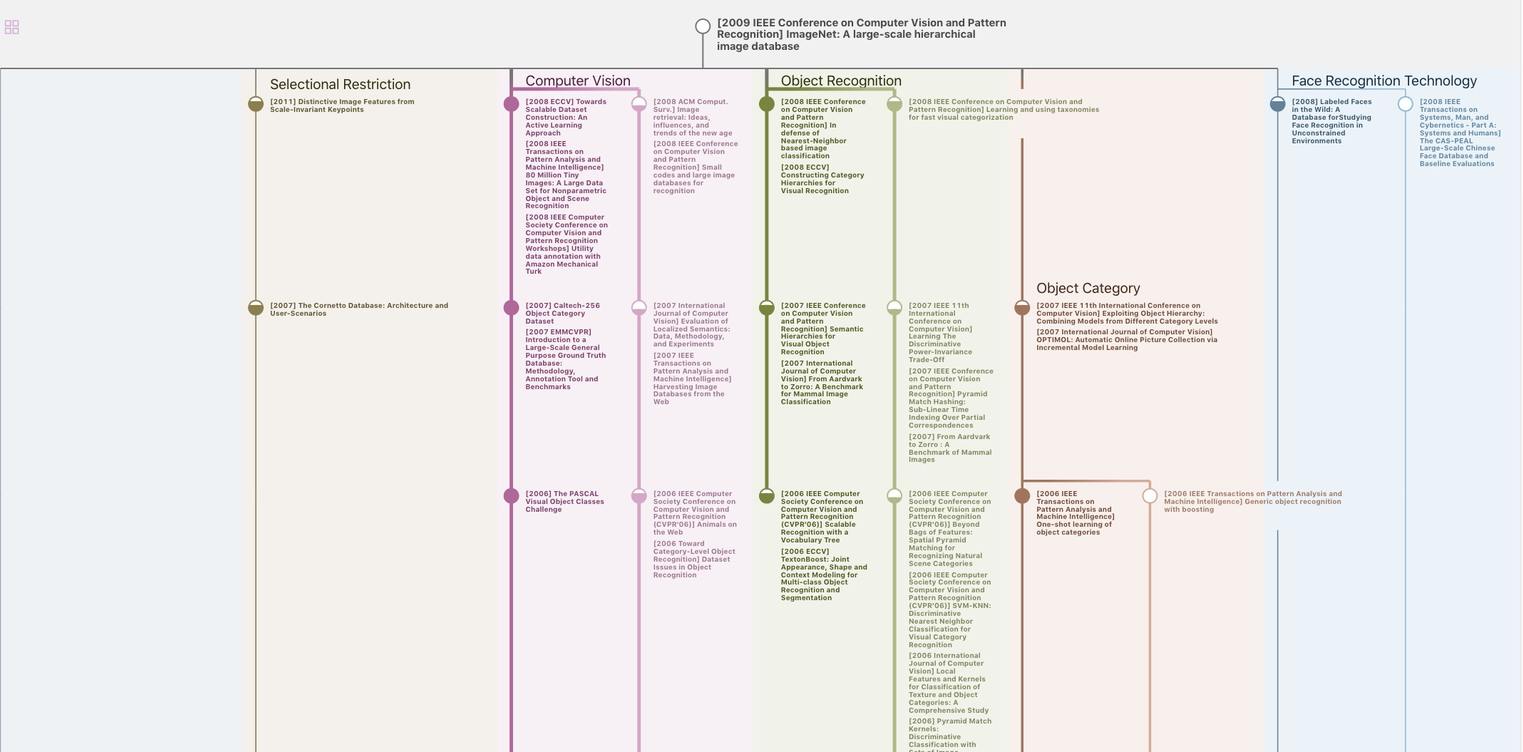
生成溯源树,研究论文发展脉络
Chat Paper
正在生成论文摘要