Knowledge-based Bi-correction model for achieving effective lag-free characteristic on daily urban water demand forecasting
Expert Systems with Applications(2024)
摘要
Water is an indispensable resource for urban residents, and accurate demand forecasting is crucial for the efficient management of smart cities. However, the non-stationary and nonlinear characteristics of daily water demand series pose significant challenges, often leading to lag forecasting issues and unreliable predictions. To address these challenges, this paper introduces a novel bi-correction model based on knowledge extraction, named the knowledge-based bi-correction model (KbBcM). The KbBcM leverages prior and posterior knowledge to achieve effective lag-free forecasting. The prior knowledge, in the form of sequence and feature information, is extracted using an autoregressive long short-term memory (AR-LSTM) network and a newly proposed lag penalty attention (LPA) module, respectively. Furthermore, a hybrid stacked LSTM network is employed to obtain primary forecasting results, which are then corrected using a masked weighted Markov chain based on posterior knowledge. Addressing the limitations of current metrics focused on absolute error, we also introduce the novel mean prediction trend effectiveness (MPTE) to comprehensively evaluate the effectiveness of lag-free forecasting results. Comparative experiments conducted on the urban water demand dataset of a megacity demonstrate the superiority of the KbBcM over baseline models. Additionally, ablation studies are performed to examine the effectiveness of the proposed sub-modules, further validating the robustness and reliability of the KbBcM in addressing the complexities of urban water demand forecasting.
更多查看译文
关键词
Lag-free forecasting,Urban water demand forecasting,Complex water system,Smart city
AI 理解论文
溯源树
样例
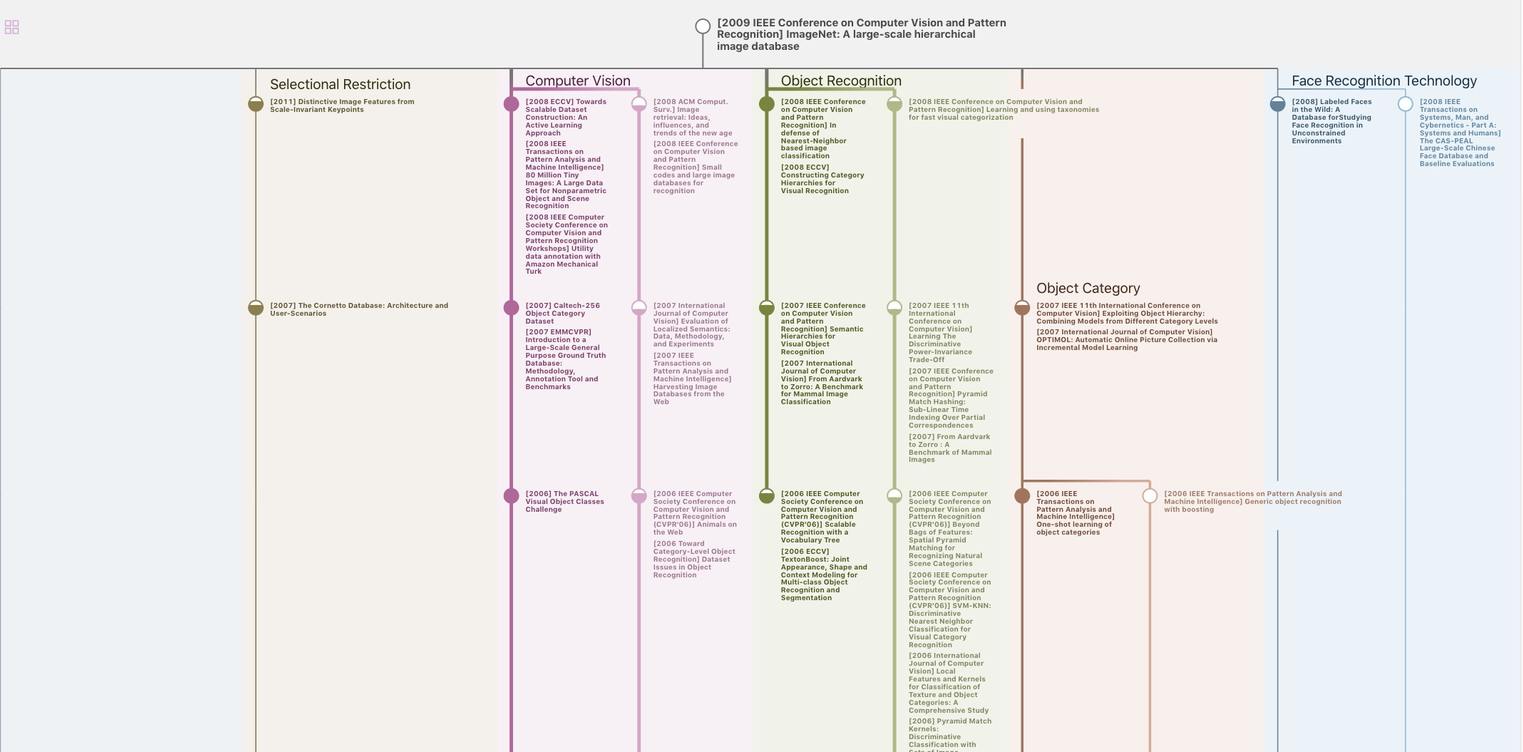
生成溯源树,研究论文发展脉络
Chat Paper
正在生成论文摘要