Machine learning-enabled discovery of multi-resonance TADF molecules: Unraveling PLQY predictions from molecular structures
Chemical Engineering Journal(2024)
Abstract
Unlocking the potential of multi-resonance thermally activated delayed fluorescence (MR-TADF) molecules for advanced organic light-emitting diode applications requires an insightful understanding of the relationship between molecular structures and photoluminescence quantum yield (PLQY). Utilizing molecular descriptors as inputs for machine learning (ML) algorithms, further illuminated by SHapley Additive exPlanations (SHAP) to interpret the ML model outcomes, this method effectively connects molecular structures to PLQY, providing targeted guidance for molecular design. A vast molecular library is generated via variational autoencoders, allowing for a comprehensive exploration of molecular space beyond conventional chemical intuition. High-throughput virtual screening, combined with our PLQY-focused model and a secondary model for emission peak wavelength prediction, efficiently identify promising candidates with blue-emitting properties. The robustness of our predictions is substantiated through quantum chemistry calculations. The integrative methodology proposed in this work not only streamlines the discovery of MR-TADF molecules but also provides a replicable framework for the intelligent design of other optoelectronic materials.
MoreTranslated text
Key words
Machine Learning,Multi-resonance thermally activated delayed fluorescence,DFT Calculations,High-throughput virtual screening,Photoluminescence quantum yield
AI Read Science
Must-Reading Tree
Example
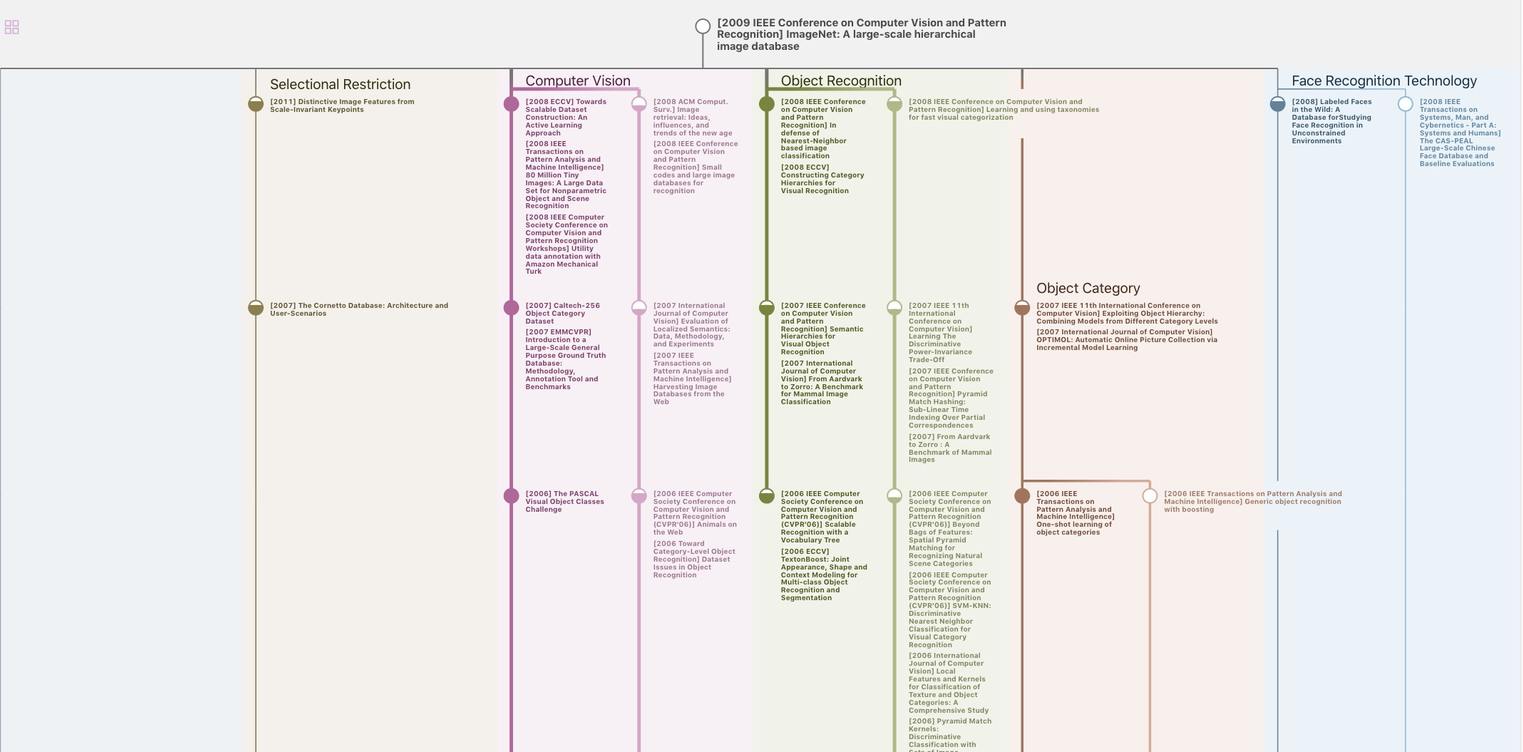
Generate MRT to find the research sequence of this paper
Chat Paper
Summary is being generated by the instructions you defined