Addressing the Accuracy-Cost Trade-off in Material Property Prediction Using a Teacher-Student Strategy.
Journal of chemical theory and computation(2024)
Abstract
Deep learning has catalyzed a transformative shift in material discovery, offering a key advantage over traditional experimental and theoretical methods by significantly reducing associated costs. Models adept at predicting properties from chemical compositions alone do not require structural information. However, this cost-efficient approach compromises model precision, particularly in Chemical Composition-based Property Prediction Models (CPMs), which are notably less accurate than Structure-based Property Prediction Models (SPMs). Addressing this challenge, our study introduces a novel Teacher-Student (TS) strategy, where a pretrained SPM serves as an instructive 'teacher' to enhance the CPM's precision. This TS strategy successfully harmonizes low-cost exploration with high accuracy, achieving a significant 47.1% reduction in relative error in scenarios involving 100 data entries. We also evaluate the effectiveness of the proposed strategy by employing perovskites as a case study. This method represents a significant advancement in the exploration and identification of valuable materials, leveraging CPM's potential while overcoming its precision limitations.
MoreTranslated text
AI Read Science
Must-Reading Tree
Example
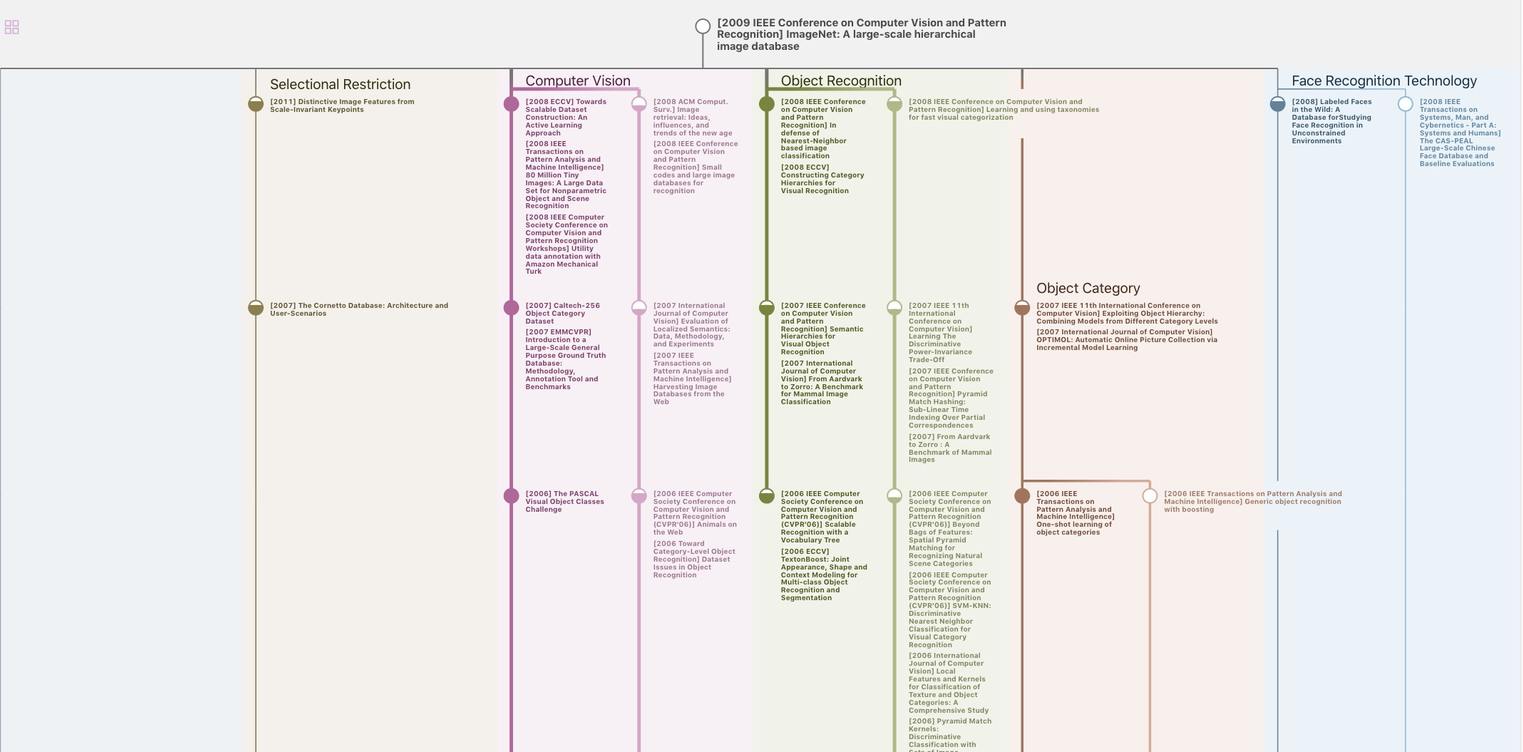
Generate MRT to find the research sequence of this paper
Chat Paper
Summary is being generated by the instructions you defined