Novel 3D photosynthetic traits derived from the fusion of UAV LiDAR point cloud and multispectral imagery in wheat
Remote Sensing of Environment(2024)
Abstract
Photosynthesis is the material basis for crop growth and yield formation. Rapid and real-time monitoring of photosynthetic parameters is essential for crop stress monitoring, light use efficiency assessment, and yield prediction. Methods based on passive optical remote sensing can accurately monitor the photosynthetic traits of crops. However, they are limited in capturing one- or two-dimensional information, and cannot obtain three-dimensional (3D) photosynthetic traits in crops. To address this issue, this study quantifies the 3D photosynthetic trait of wheat based on a digital surface model which fused a light detection and ranging (LiDAR) point cloud with multispectral imagery, both of which were acquired using an unmanned aerial vehicle (UAV). First, we constructed a model to estimate the key photosynthetic parameters (canopy chlorophyll content, fraction of absorbed photosynthetically active radiation, and canopy net photosynthetic rate) based on selected multi-spectral indices in wheat. Second, we applied the model to a point cloud that had been fused with spectral reflectance imagery to generate the 3D spatial and vertical distributions of the above three photosynthetic parameters, and then estimated the 3D photosynthetic traits. Third, we analyzed the 3D photosynthetic traits of wheat in terms of cultivars, growth stages, and cultivation management, and assessed the ability of the derived metrics based on the 3D photosynthetic traits to predict yield. The results showed that the LiDAR point cloud fused with multispectral images could accurately characterize the 3D spatial distribution of the photosynthetic parameters of wheat under different conditions. The photosynthetic traits of the different horizontal layers (upper, middle, and lower) of the canopy were statistically different. In addition, two new developed 3D metrics (CPn_P75th and CCC_P75th), which were derived from the 3D photosynthetic parameters based on the fusion of point cloud and multispectral data, could predict yield with a higher accuracy and greater robustness than traditional methodology of agronomic traits or remote sensing. This study demonstrate that the fusion of LiDAR point cloud and multispectral imagery is feasible for the accurate quantification of 3D photosynthetic traits and the screening of high-yielding genetic cultivars in crops. In addition, we found that multispectral point clouds generated from UAV multispectral imagery using structure from motion (SfM) combined with multi-view stereo (MVS) algorithms (SfM-MVS) can also be used to estimate the 3D spatial distributions of photosynthetic parameters in crops. Despite the fact that the multispectral point cloud by SfM-MVS is sparse and incomplete, this method has potential application in the study of 3D spatial distribution of crop parameters.
MoreTranslated text
Key words
LiDAR,Multispectral,Fusion,3D photosynthetic traits,Wheat,Yield
AI Read Science
Must-Reading Tree
Example
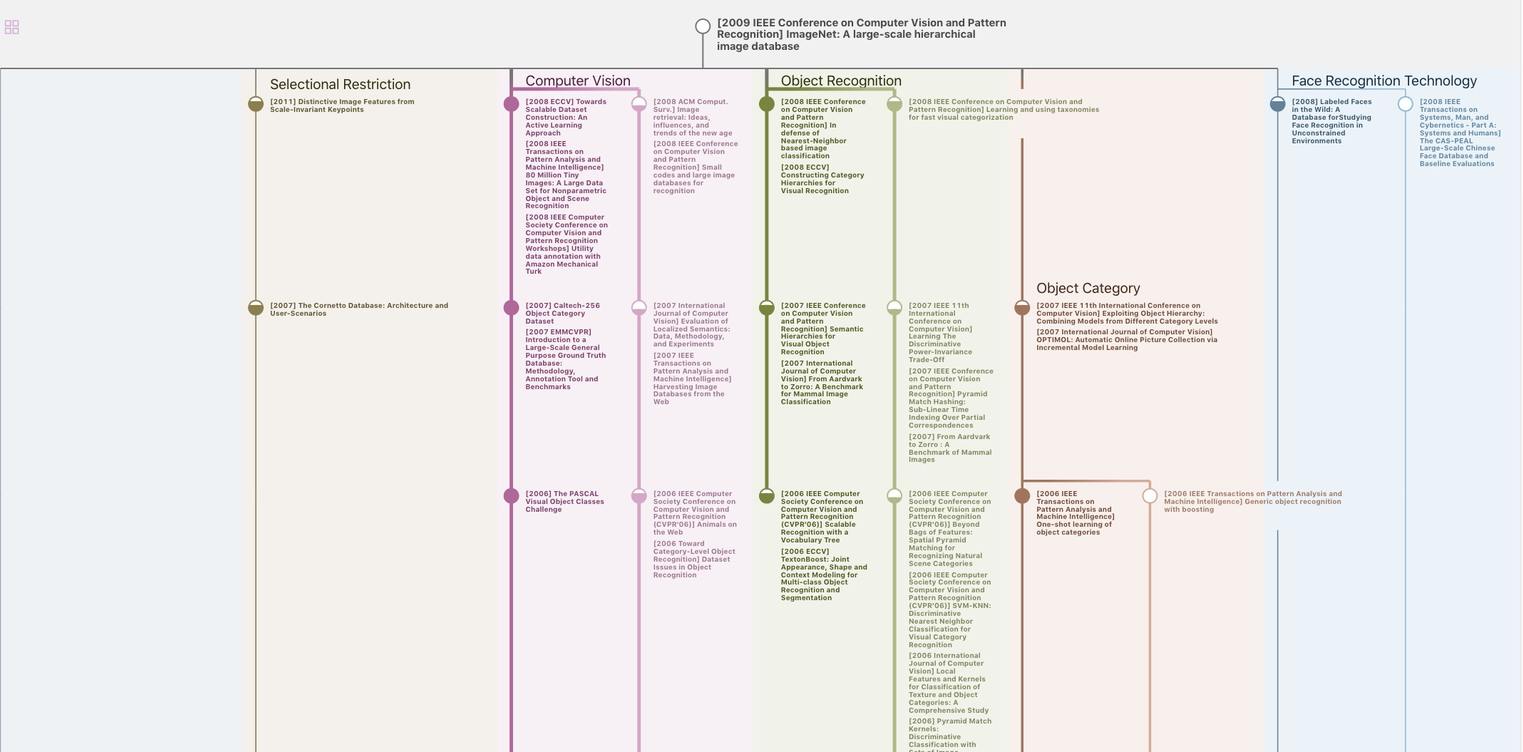
Generate MRT to find the research sequence of this paper
Chat Paper
Summary is being generated by the instructions you defined