Determining water and solute permeability of reverse osmosis membrane using a data-driven machine learning pipeline
Journal of Water Process Engineering(2024)
摘要
Water (A) and solute permeability (B) are the key parameters characterizing the performance of reverse osmosis (RO) membranes. However, determining A and B requires multiple times of experiments that are time-consuming. Thus, developing a method to estimate A and B quickly has been encouraged. This study introduces a data-driven machine learning (ML) pipeline designed to predict A and B for RO membranes. The ML pipeline addresses all stages for estimating A and B, from data preprocessing to practical guidance on developed models. In particular, the membrane composition data, an overlooked essential component detailing A and B, was employed as features for the ML models. Sixteen ML models were tested, and categorical boost (CatBoost) and extremely randomized trees (ET) were selected as the best models for A and B, respectively. The selected ML models were retrained using the minimum yet effective features identified through feature importance analysis. Consequently, the retrained ML models exhibited high accuracy in predicting A and B (Radj,test2 = 0.925/MAEtest = 0.246 for A; Radj,test2 = 0.986/MAEtest = 0.069 for B), demonstrating the viability of a data-driven approach for parameter estimation. Moreover, this study highlights that RO membrane performance can be gauged effectively using membrane composition data and a limited set of operating conditions.
更多查看译文
关键词
Machine learning,Parameters,Reverse osmosis membrane,Solute permeability,Water permeability
AI 理解论文
溯源树
样例
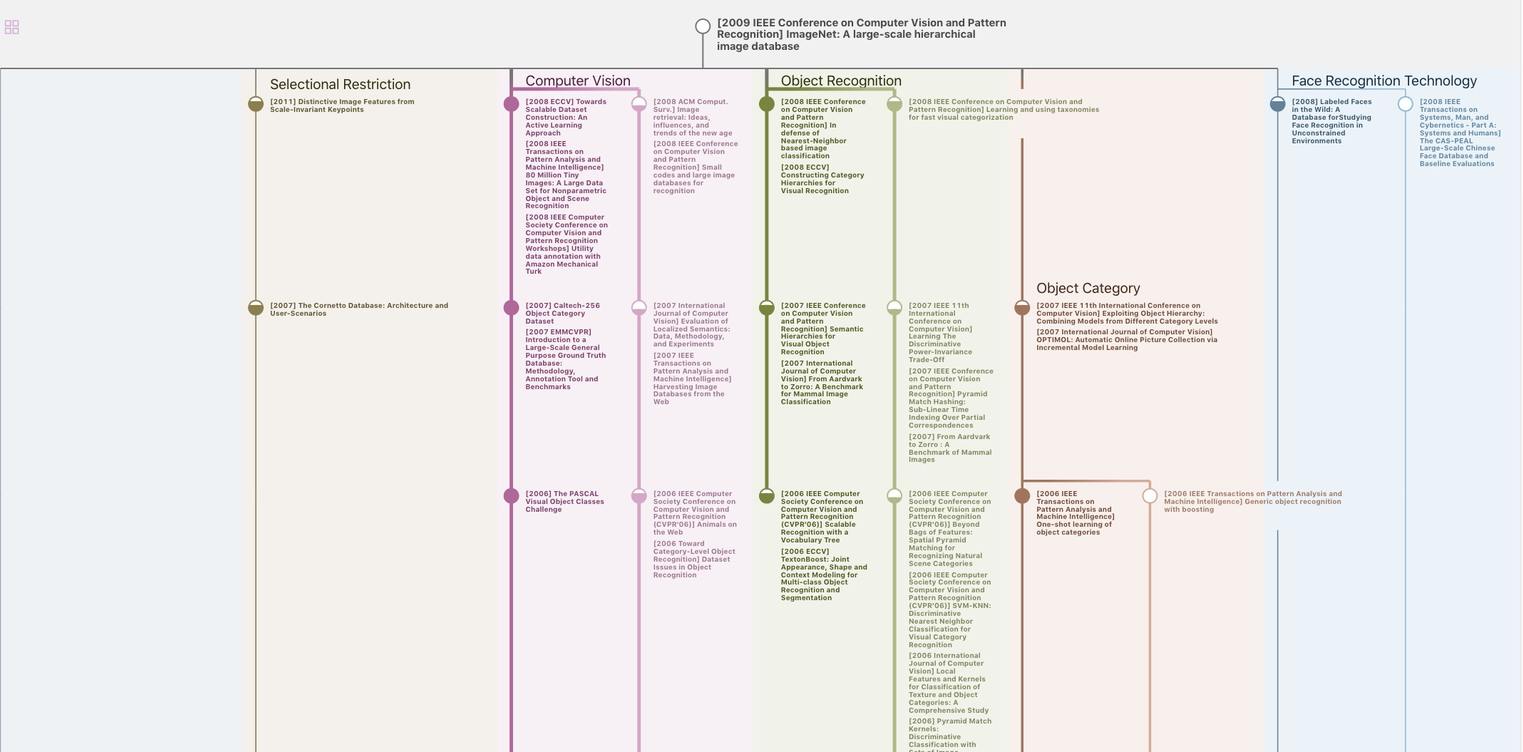
生成溯源树,研究论文发展脉络
Chat Paper
正在生成论文摘要