Resource Allocation for Dynamic Platoon Digital Twin Networks: A Multi-Agent Deep Reinforcement Learning Method
IEEE Transactions on Vehicular Technology(2024)
摘要
Vehicle driving in a platoon is an efficient and ecological driving solution. Introducing the concept of digital twin (DT) into the platoon to establish platoon digital twin (PDT) can improve the management efficiency and driving safety of the platoon. However, the joint allocation of multiple types of resources in a platoon digital twin network (PDTN) is an important issue for the successful implementation and maintenance of the PDT. In this paper, we investigate the resource allocation problem in a PDTN. By comprehensively considering the effects of high mobility of platooning vehicles, real-time nature of the DTs, and multi-vehicle cooperation, we propose a PDT utility optimization model for bandwidth and computation resource allocation. We formulate the dynamic resource allocation problem as an M-th order Markov decision process (MDP) and design a deep reinforcement learning (DRL)-based dynamic resource allocation (DRLDRA) method to solve it. To optimize the actions of the agent, we reshape the state in a smaller time granularity to better reflect the temporal variations of the state. Correspondingly, we design temporal feature extraction neural networks (TFENNs) based on multi-head self-attention (MHSA) mechanism and long short-term memory (LSTM) to extract the temporal features of the state. To improve the learning efficiency, a decentralized multi-agent deep deterministic policy gradient (DDPG)-based learning framework is proposed. Numerical results show that the DRLDRA method performs excellently and outperforms other benchmark methods.
更多查看译文
关键词
Digital twin,platoon,resource allocation,deep reinforcement learning
AI 理解论文
溯源树
样例
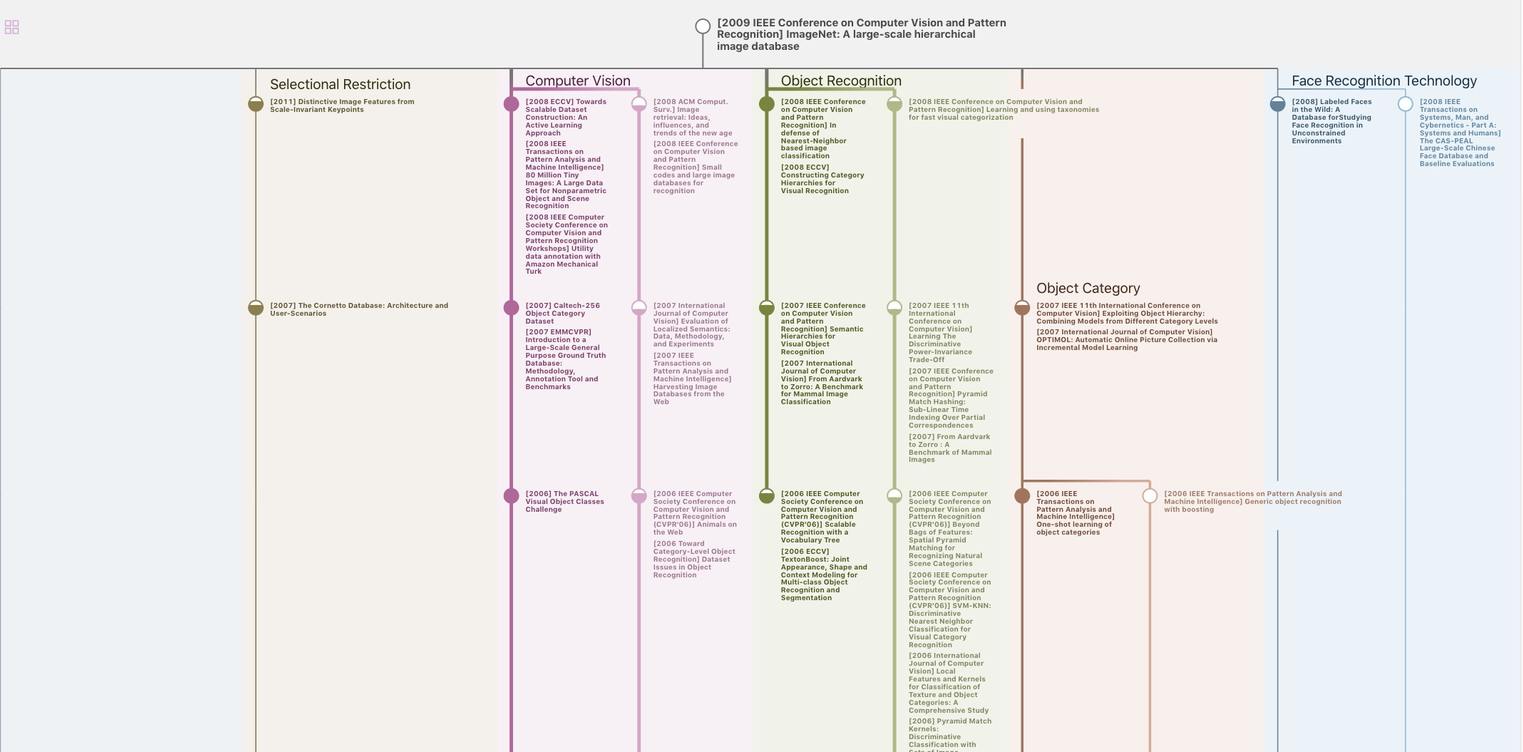
生成溯源树,研究论文发展脉络
Chat Paper
正在生成论文摘要