CGFTrans: Cross-Modal Global Feature Fusion Transformer for Medical Report Generation.
IEEE journal of biomedical and health informatics(2024)
摘要
Medical report generation, as a cross-modal automatic text generation task, can be highly significant both in research and clinical fields. The core is to generate diagnosis reports in clinical language from medical images. However, several limitations persist, including a lack of global information, inadequate cross-modal fusion capabilities, and high computational demands. To address these issues, we propose cross-modal global feature fusion Transformer (CGFTrans) to extract global information meanwhile reduce computational strain. Firstly, we introduce mesh recurrent network to capture inter-layer information at different levels to address the absence of global features. Then, we design feature fusion decoder and define 'mid-fusion' strategy to separately fuse visual and global features with medical report embeddings, which enhances the ability of the cross-modal joint learning. Finally, we integrate shifted window attention into Transformer encoder to alleviate computational pressure and capture pathological information at multiple scales. Extensive experiments conducted on three datasets demonstrate that the proposed method achieves average increments of 2.9%, 1.5%, and 0.7% in terms of the BLEU-1, METEOR and ROUGE-L metrics, respectively. Besides, it achieves average increments -22.4% and 17.3% training time and images throughput, respectively.
更多查看译文
关键词
Medical report generation,Transformer,Multimodal Learning,Feature fusion,Global feature
AI 理解论文
溯源树
样例
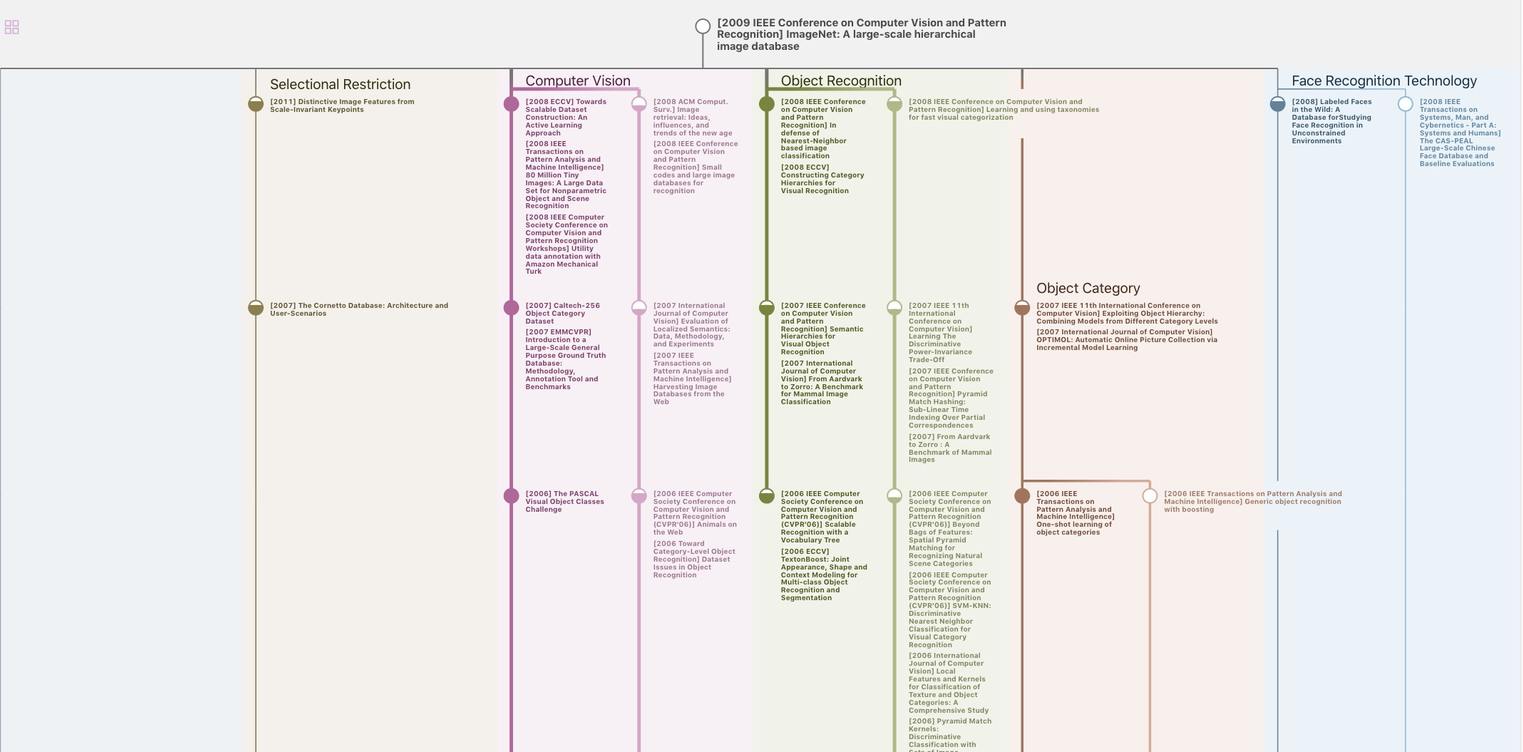
生成溯源树,研究论文发展脉络
Chat Paper
正在生成论文摘要