Exploring technology fusion by combining latent Dirichlet allocation with Doc2vec: a case of digital medicine and machine learning
Scientometrics(2024)
摘要
As a driving force behind innovation, technological fusion has emerged as a prevailing trend in knowledge innovation. However, current research lacks the semantic analysis and identification of knowledge fusion across technological domains. To bridge this gap, we propose a strategy that combines the latent Dirichlet allocation (LDA) topic model and the Doc2vec neural network semantic model to identify fusion topics across various technology domains. Then, we fuse the semantic information of patents to measure the characteristics of fusion topics in terms of knowledge diversity, homogeneity and cohesion. Applying this method to a case study in the fields of digital medicine and machine learning, we identify six fusion topics from two technology domains, revealing two distinct trends: diffusion from the center to the periphery and clustering from the periphery to the center. The study shows that the fusion measure of topic-semantic granularity can reveal the variability of technology fusion processes at a profound level. The proposed research method will benefit scholars in conducting multi-domain technology fusion research and gaining a deeper understanding of the knowledge fusion process across technology domains from a semantic perspective.
更多查看译文
关键词
Technology fusion,Latent Dirichlet allocation,Doc2vec,Semantic information,Fusion metrics
AI 理解论文
溯源树
样例
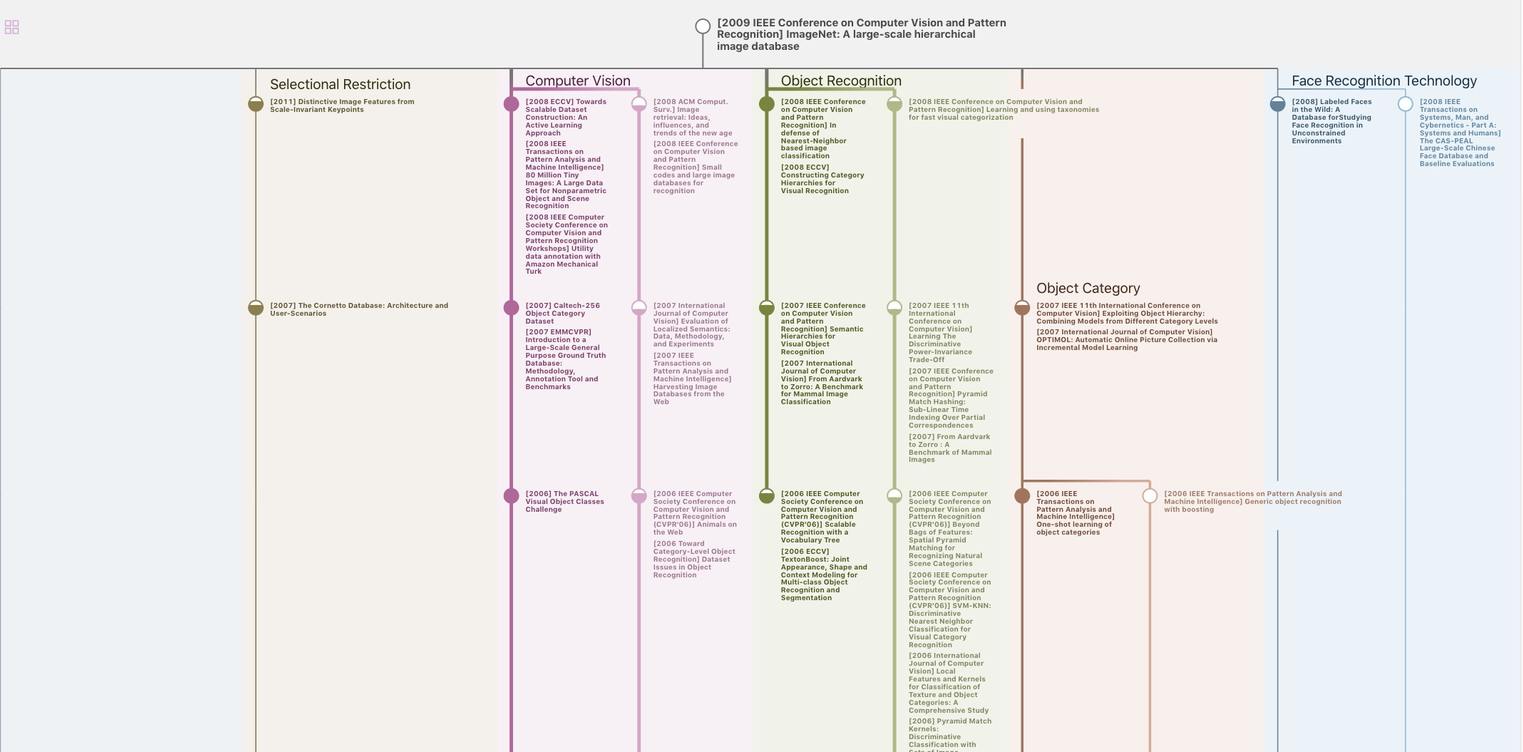
生成溯源树,研究论文发展脉络
Chat Paper
正在生成论文摘要