Synthetic surrogates improve power for genome-wide association studies of partially missing phenotypes in population biobanks.
Nature genetics(2024)
Abstract
Within population biobanks, incomplete measurement of certain traits limits the power for genetic discovery. Machine learning is increasingly used to impute the missing values from the available data. However, performing genome-wide association studies (GWAS) on imputed traits can introduce spurious associations, identifying genetic variants that are not associated with the original trait. Here we introduce a new method, synthetic surrogate (SynSurr) analysis, which makes GWAS on imputed phenotypes robust to imputation errors. Rather than replacing missing values, SynSurr jointly analyzes the original and imputed traits. We show that SynSurr estimates the same genetic effect as standard GWAS and improves power in proportion to the quality of the imputations. SynSurr requires a commonly made missing-at-random assumption but relaxes the requirements of existing imputation methods by not requiring correct model specification. We present extensive simulations and ablation analyses to validate SynSurr and apply it to empower the GWAS of dual-energy X-ray absorptiometry traits within the UK Biobank.
MoreTranslated text
AI Read Science
Must-Reading Tree
Example
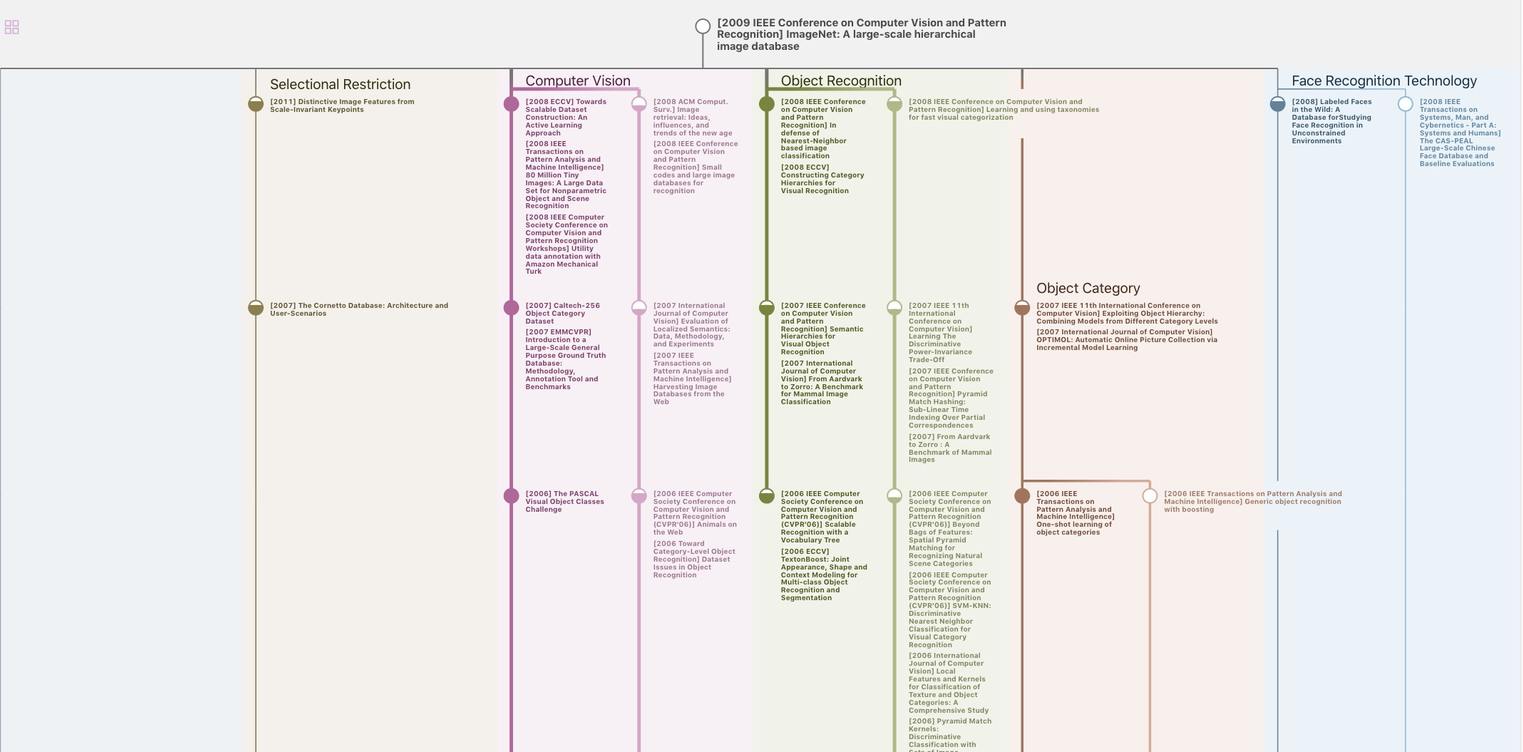
Generate MRT to find the research sequence of this paper
Chat Paper
Summary is being generated by the instructions you defined