Supervised learning of enhancer-promoter specificity based on genome-wide perturbation studies highlights areas for improvement in learning.
Bioinformatics (Oxford, England)(2024)
摘要
MOTIVATION:Understanding the rules that govern enhancer-driven transcription remains a central unsolved problem in genomics. Now with multiple massively parallel enhancer perturbation assays published, there are enough data that we can utilize to learn to predict enhancer-promoter relationships in a data-driven manner.
RESULTS:We applied machine learning to one of the largest enhancer perturbation studies integrated with transcription factor and histone modification ChIP-seq. The results uncovered a discrepancy in the prediction of genome-wide data compared to data from targeted experiments. Relative strength of contact was important for prediction, confirming the basic principle of EP regulation. Novel features such as the density of the enhancers/promoters in the genomic region was found to be important, highlighting our lack of understanding on how other elements in the region contribute to the regulation. Several TF peaks were identified that improved the prediction by identifying the negatives and reducing False Positives. In summary, integrating genomic assays with enhancer perturbation studies increased the accuracy of the model, and provided novel insights into the understanding of enhancer-driven transcription.
AVAILABILITY:The trained models, data and the source code are available at http://doi.org/10.5281/zenodo.11290386 and https://github.com/HanLabUNLV/sleps.
SUPPLEMENTARY INFORMATION:Supplementary data are available at Bioinformatics online.
更多查看译文
AI 理解论文
溯源树
样例
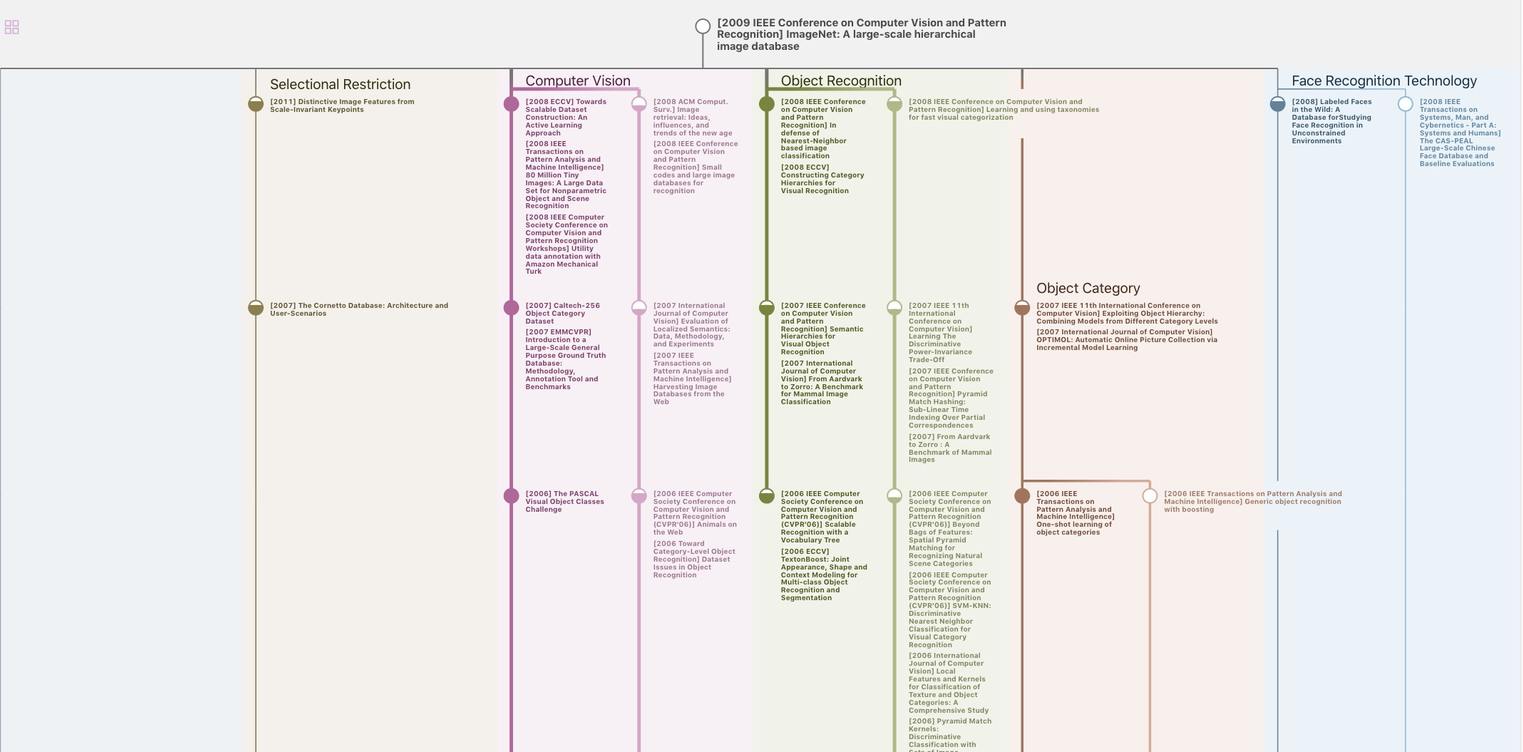
生成溯源树,研究论文发展脉络
Chat Paper
正在生成论文摘要