WE-Validate: An Open-Source Framework For Wind Power Validation
2024 IEEE Conference on Technologies for Sustainability (SusTech)(2024)
摘要
Grid operators rely on historical weather time series at existing and planned wind power plants to make informed decisions when planning for a future power grid with a very high penetration of renewable power. While synthetic wind power time series have been developed using historical weather models, their validation with actual power production data remains complex because of variations in modeling practices and methodologies. This paper introduces the WE-Validate framework, originally designed for wind speed validation and now enhanced for wind power validation with a graphical user interface to support users with minimal programming experience. Validation of the wind power with WE-Validate is based on robust metrics: the root mean squared error (RMSE), centered RMSE, average bias, average percent bias, mean absolute error, mean absolute percent error, cross correlation, and calculations of the ramp magnitude, rate, and duration. This paper showcases WE-Validate with validation of the synthetically derived power for a wind plant in Washington state for July to December 2018. The synthetic power was validated by comparing two datasets with observations. This comparison shows that both series are strongly correlated with the observed data across monthly aggregations, despite a persistent negative bias. The metrics within WE-Validate facilitate immediate insight into the utility of the comparison datasets, enabling analysis across multiple dimensions. This user-friendly, open-source tool can be extended beyond wind power, making it a valuable resource for system planners and operators in different domains.
更多查看译文
关键词
Wind Power,Time Series,Root Mean Square Error,Mean Square Error,Root Mean,Observational Data,Cross-correlation,December 2018,Mean Absolute Error,Power Plants,Power Grid,Graphical User Interface,Comparison Of Datasets,Washington State,Actual Production,Ramp Rate,Power Production,Mean Absolute Percentage Error,Numerical Weather Prediction,Utility Grid,Power Series,Validation Metrics,Transparency Framework,Department Of Energy,October 2018,Spread Of Data,Validation Framework,Reanalysis Datasets,July 2018
AI 理解论文
溯源树
样例
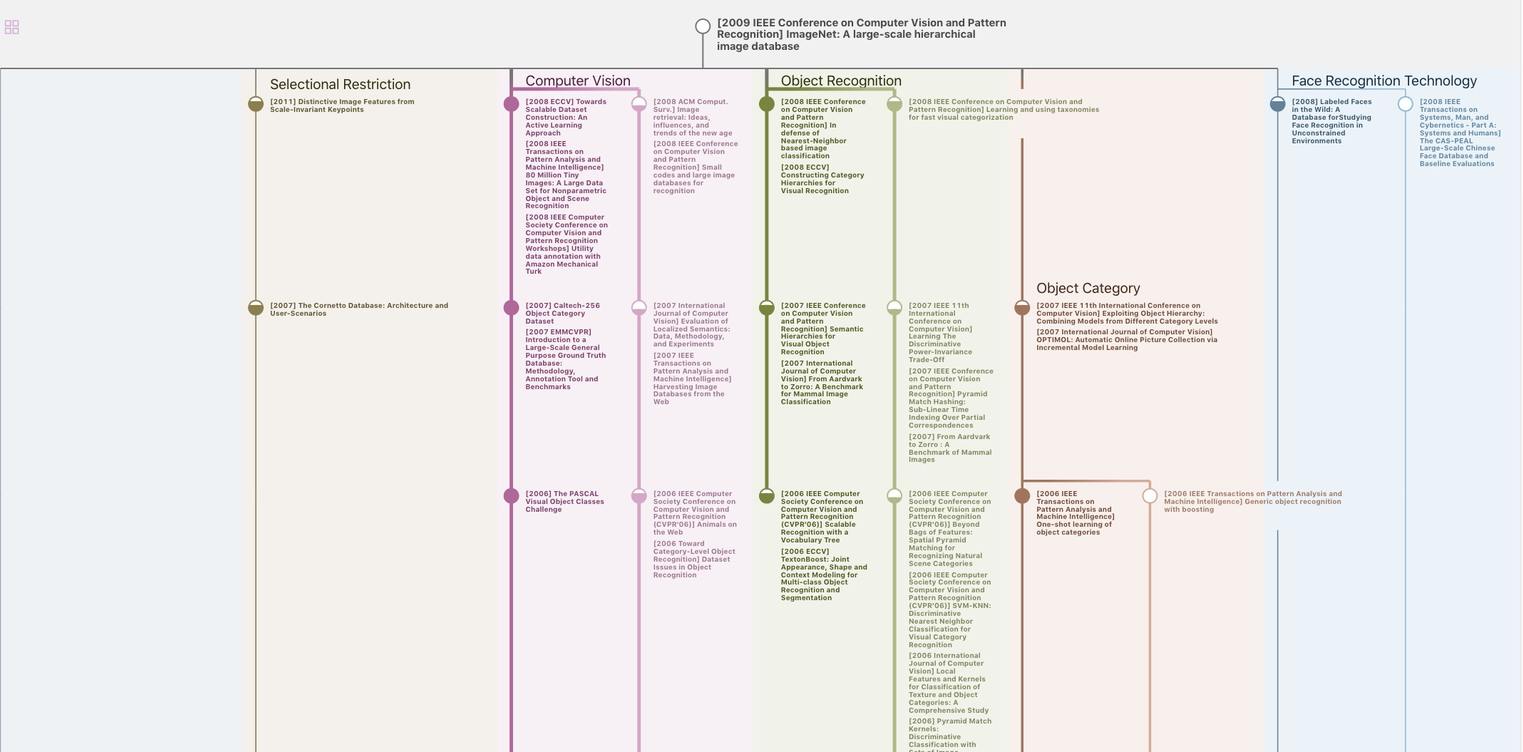
生成溯源树,研究论文发展脉络
Chat Paper
正在生成论文摘要