DPCF-Net: 2D Medical Image Segmentation Network Based on Dual-Path Cross-Fusion Encoder
2024 7th International Symposium on Autonomous Systems (ISAS)(2024)
Abstract
Precise medical image segmentation is a crucial step in treating and detecting different diseases, organs, and tissues. The labels of medical image datasets used for segmentation usually exhibit variations in size and shape, with background labels outweighing target labels. Existing methods struggle to extract feature information pertinent to segmentation tasks effectively, and they face challenges in designing extraction mechanisms for both local and global feature information, resulting in feature redundancy. We propose the Dual-Path Cross-Fusion Network (DPCF-Net), a medical image segmentation network based on a Dual-Path Cross-Fusion Encoder (DCF-Encoder) to address these issues. DCF-Encoder collaboratively utilizes large-kernel convolutional Encoder and small-kernel convolutional Encoder to extract both effective local and global feature information. Additionally, we introduce a fusion mechanism (2DEPA) for skip connections, enabling the effective fusion of feature maps from corresponding modules of the large-kernel and small-kernel convolutional Encoder. The fused feature maps are fed into the Decoder to obtain the final segmentation label map. Finally, we validate the effectiveness and robust generalization capability of DPCF-Net using two datasets. Experiments demonstrate that DPCF-Net outperforms the baseline networks’ segmentation performance and exhibits more robust generalization capabilities.
MoreTranslated text
Key words
Medical image segmentation,local features,global features,large-kernel convolution,fusion mechanism
AI Read Science
Must-Reading Tree
Example
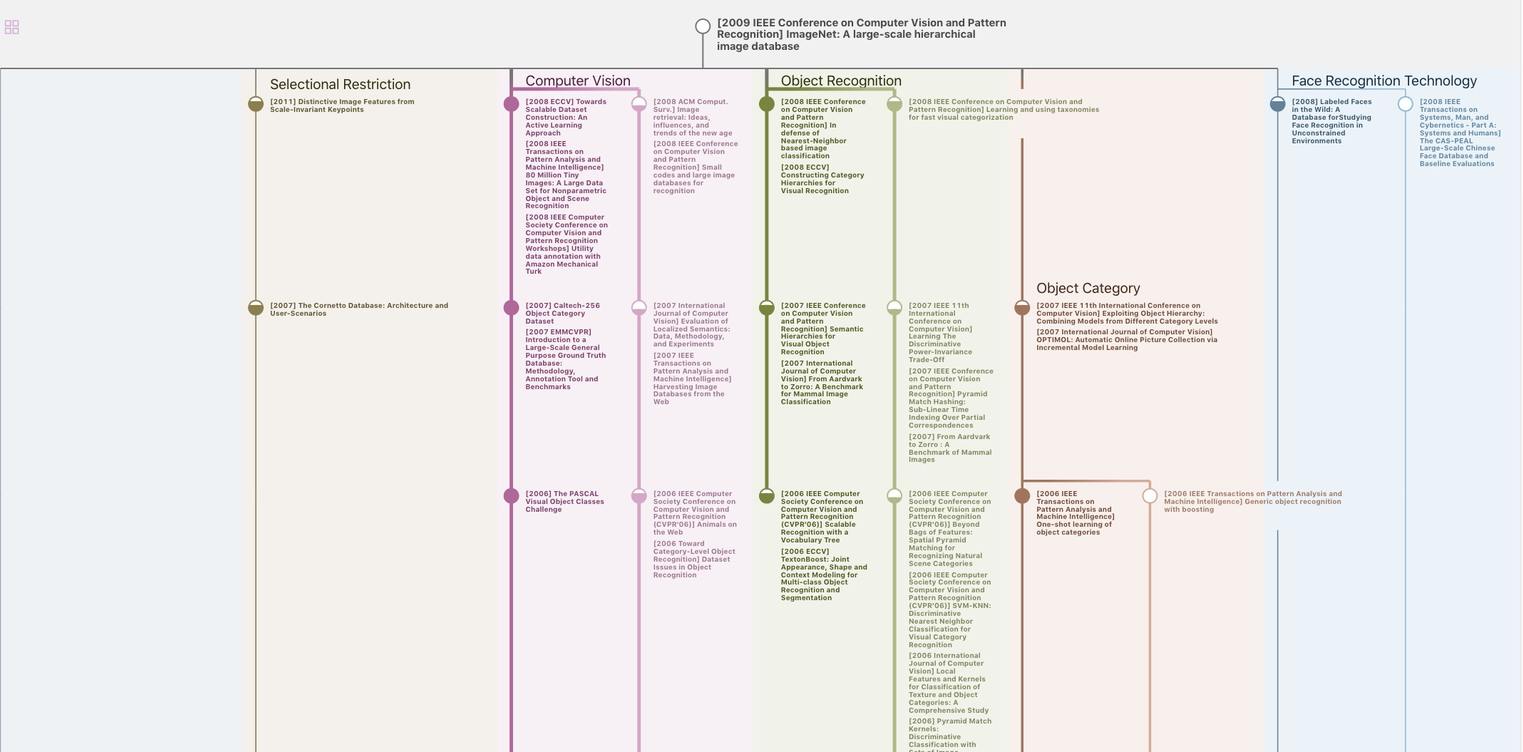
Generate MRT to find the research sequence of this paper
Chat Paper
Summary is being generated by the instructions you defined