ONeK-SLAM: A Robust Object-Level Dense SLAM Based on Joint Neural Radiance Fields and Keypoints
ICRA 2024(2024)
摘要
Neural implicit representation has recently achieved significant advancements, especially in the field of SLAM(Simultaneous Localization and Mapping). Previous NeRF-based SLAM methods have difficulties with object-level localization and reconstruction and struggle in dynamic and illumination-varied environments. We propose ONeK-SLAM, a robust object-level SLAM system that effectively combines feature points and neural radiance fields. ONeK-SLAM uses the joint information at the object level to improve localization accuracy and enhance reconstruction details. Moreover, our approach detects and eliminates dynamic objects based on the joint errors, while also harnessing the illumination invariance offered by feature points. Consequently, ONeK-SLAM achieves high-precision localization and detailed object-level mapping, even in dynamic and illumination-varying environments. Our evaluations, conducted on three public datasets that include both dynamic and variable lighting sequences, demonstrate that our method outperforms recent NeRF-based SLAM method in both localization and reconstruction.
更多查看译文
关键词
SLAM,Localization,Mapping
AI 理解论文
溯源树
样例
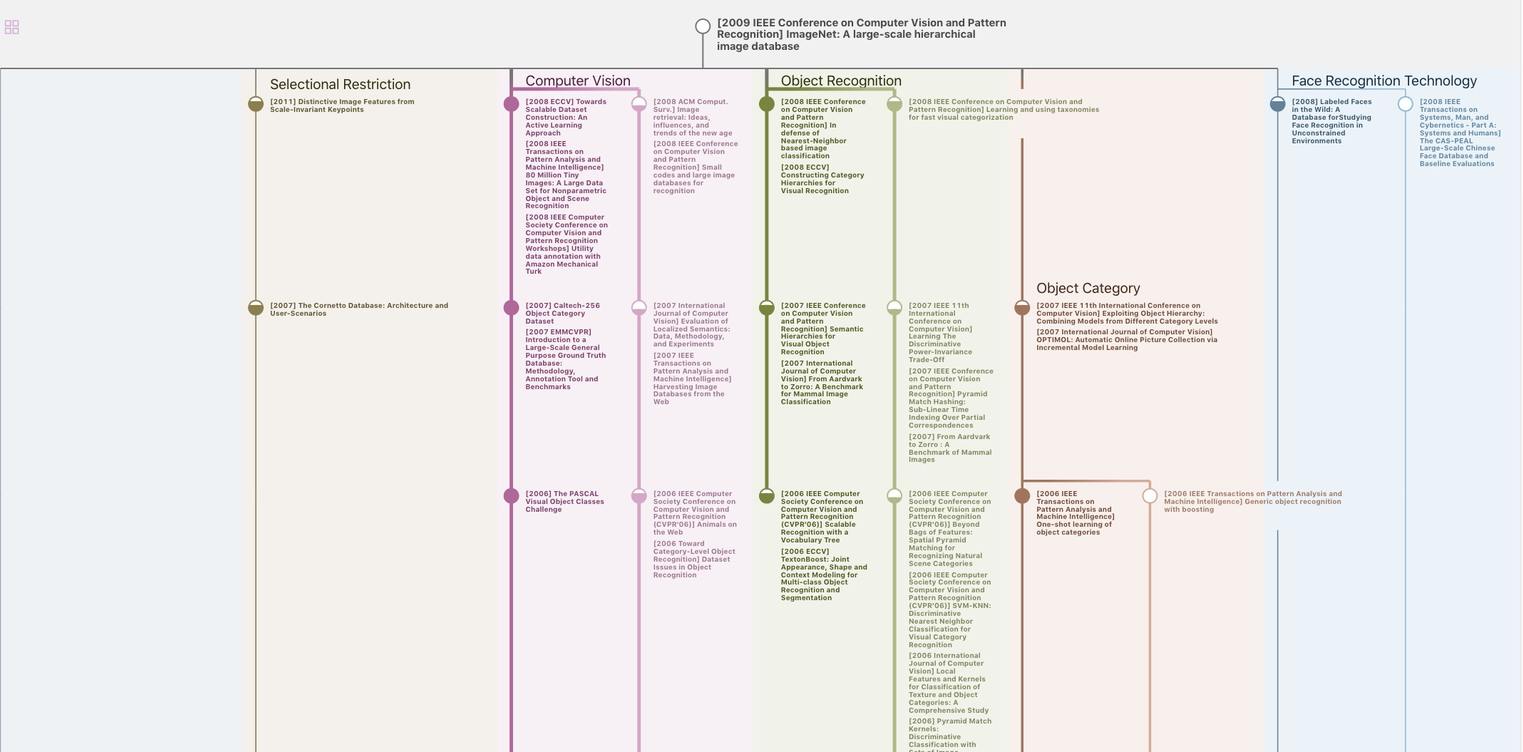
生成溯源树,研究论文发展脉络
Chat Paper
正在生成论文摘要