Projection-Based Fast and Safe Policy Optimization for Reinforcement Learning
ICRA 2024(2024)
摘要
While reinforcement learning (RL) attracts increasing research attention, maximizing the return while keeping the agent safe at the same time remains an open problem. Motivated to address this challenge, this work proposes a new Fast and Safe Policy Optimization (FSPO) algorithm, which consists of three steps: the first step involves reward improvement update, the second step projects the policy to the neighborhood of the baseline policy to accelerate the optimization process, and the third step addresses the constraint violation by projecting the policy back onto the constraint set. Such a projection-based optimization can improve the convergence and learning performance. Unlike many existing works that require convex approximations for the objectives and constraints, this work exploits a first- order method to avoid expensive computations and high dimensional issues, enabling fast and safe policy optimization, especially for challenging tasks. Numerical simulation and physical experiments demonstrate that FSPO outperforms existing methods in terms of safety guarantees and task completion rate.
更多查看译文
关键词
Reinforcement Learning,Task and Motion Planning
AI 理解论文
溯源树
样例
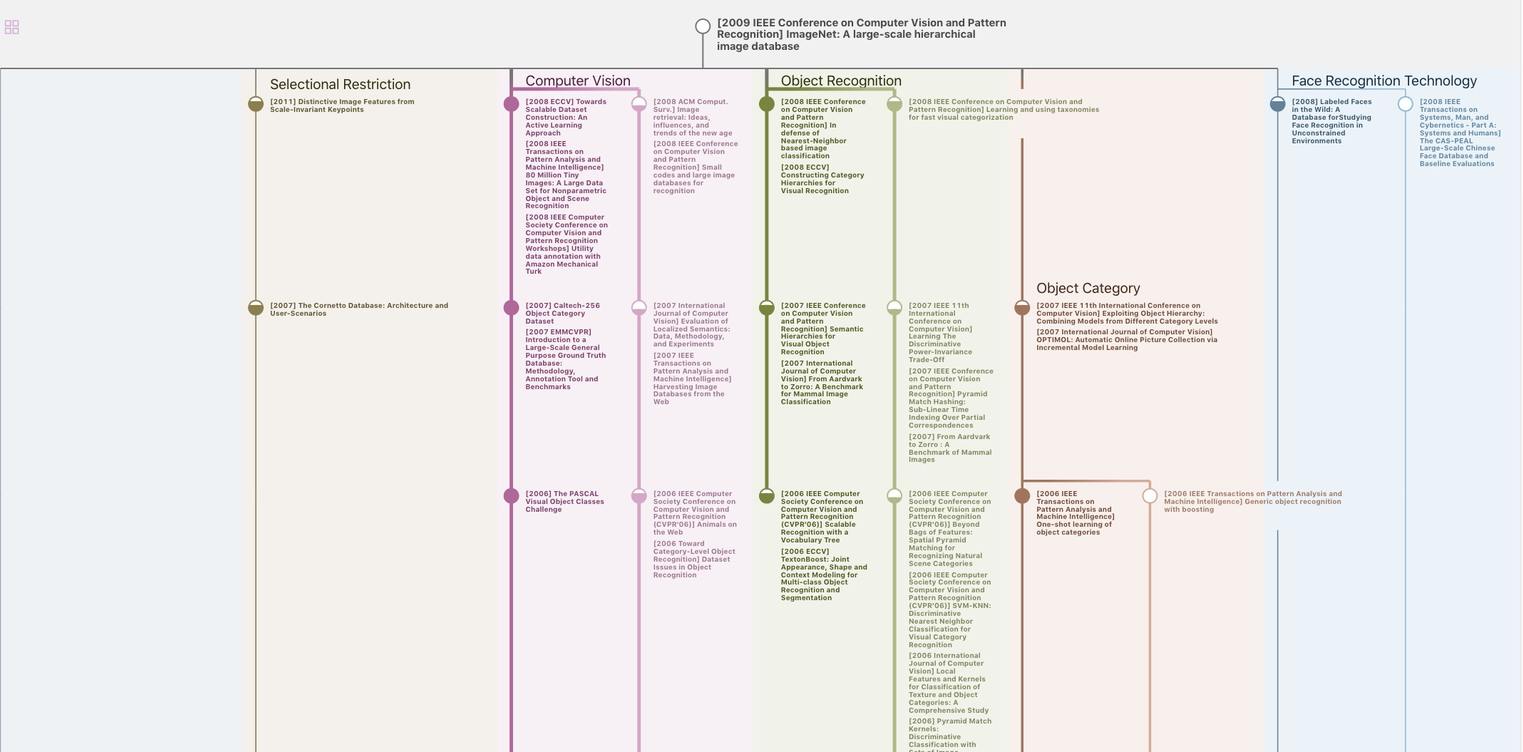
生成溯源树,研究论文发展脉络
Chat Paper
正在生成论文摘要