Learning Interaction Constraints for Robot Manipulation Via Set Correspondences
ICRA 2024(2024)
摘要
Cross-pose estimation between rigid objects is a fundamental building block for robotic applications. In this paper, we propose a new cross-pose estimation method that predicts correspondences on a set level as opposed to a point level. This contrasts methods that predict cross-pose from per-point correspondences, which can encounter optimization problems for objects with symmetries, since each point may have multiple valid correspondences. Our method, SCAlign, consists of a Set Correspondence Network (SCN) which predicts these sets and their correspondences, and an alignment module to compute their relative cross-pose. Taking point clouds of two objects as input, SCN predicts a set label for each point such that such that points that share a set label form a cross object correspondence. The alignment module then computes the cross-pose as the SE(3) transformation that aligns these set correspondences. We compare SCAlign against other cross- pose estimation baselines on a synthetically generated dataset, SynWidth, which contains randomly generated width-mate objects with symmetric or near-symmetric intercepts. SCAlign significantly outperforms the baselines on this challenging dataset. Additionally, we show that set correspondences can be leveraged to distinguish positive and negative matches between pegs and holes. Robot experiments further validate the practical application of this approach.
更多查看译文
关键词
Perception for Grasping and Manipulation,Deep Learning for Visual Perception
AI 理解论文
溯源树
样例
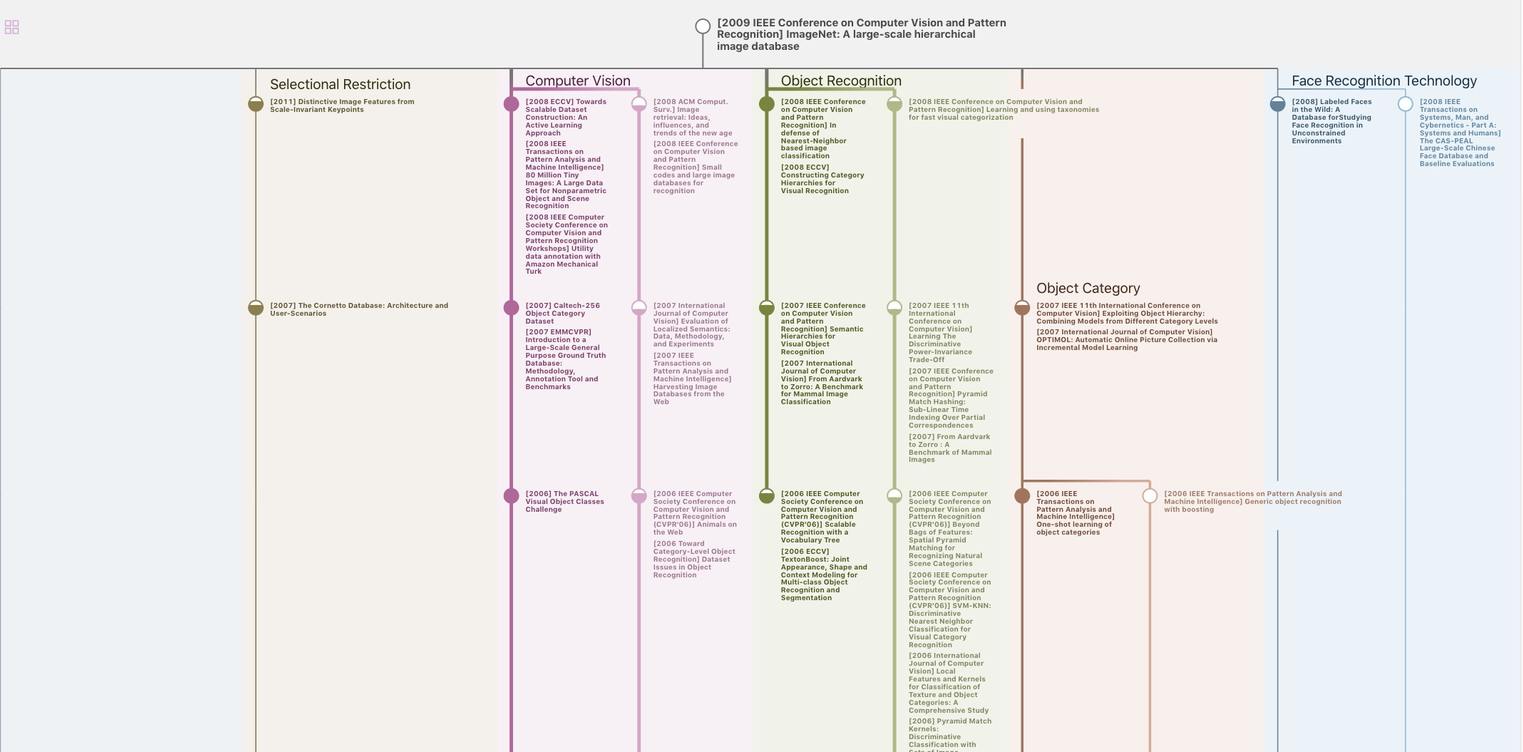
生成溯源树,研究论文发展脉络
Chat Paper
正在生成论文摘要