Stranger Danger! Identifying and Avoiding Unpredictable Pedestrians in RL-Based Social Robot Navigation
ICRA 2024(2024)
Key words
Human-Aware Motion Planning,Reinforcement Learning
AI Read Science
Must-Reading Tree
Example
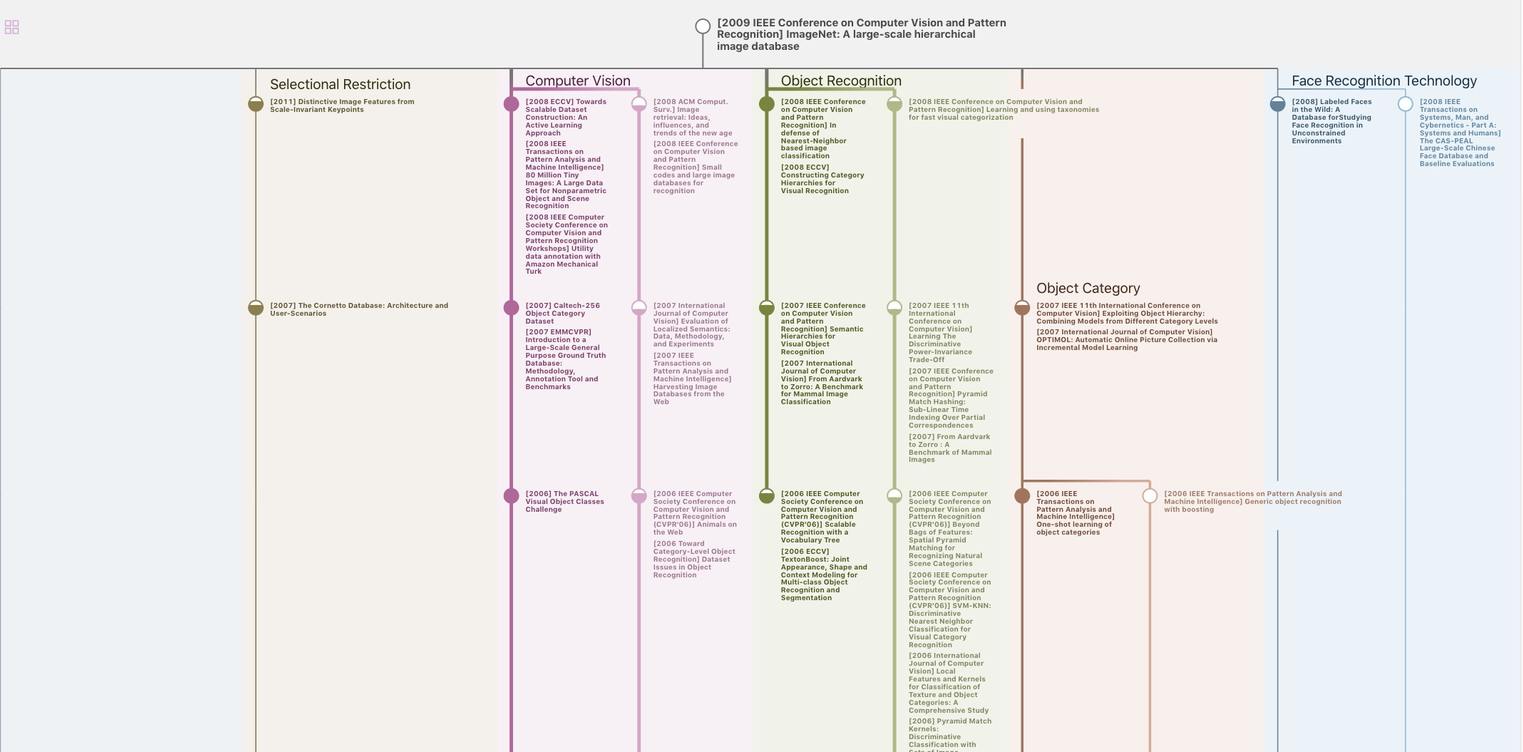
Generate MRT to find the research sequence of this paper
Chat Paper
Summary is being generated by the instructions you defined