SM^3: Self-Supervised Multi-Task Modeling with Multi-View 2D Images for Articulated Objects
ICRA 2024(2024)
摘要
Reconstructing real-world objects and estimating their movable joint structures are pivotal technologies within the field of robotics. Previous research has predominantly focused on supervised approaches, relying on extensively annotated datasets to model articulated objects within limited categories. However, this approach falls short of effectively addressing the diversity present in the real world. To tackle this issue, we propose a self-supervised interaction perception method, referred to as SM3, which leverages multi-view RGB images captured before and after interaction to model articulated objects, identify movable parts, and infer the parameters of their rotating joints. By constructing 3D geometries and textures from the captured 2D images, SM3 achieves integrated optimization of movable parts and joint parameters during the reconstruction process, obviating the need for annotations. Furthermore, we introduce the MMArt dataset, an extension of PartNet-Mobility, encompassing multi-view and multi-modal data of articulated objects spanning diverse categories. Evaluations demonstrate that UM3 surpasses existing benchmarks across various categories and objects, while its adaptability in real-world scenarios has been duly validated.
更多查看译文
关键词
Deep Learning for Visual Perception,Computer Vision for Automation,Simulation and Animation
AI 理解论文
溯源树
样例
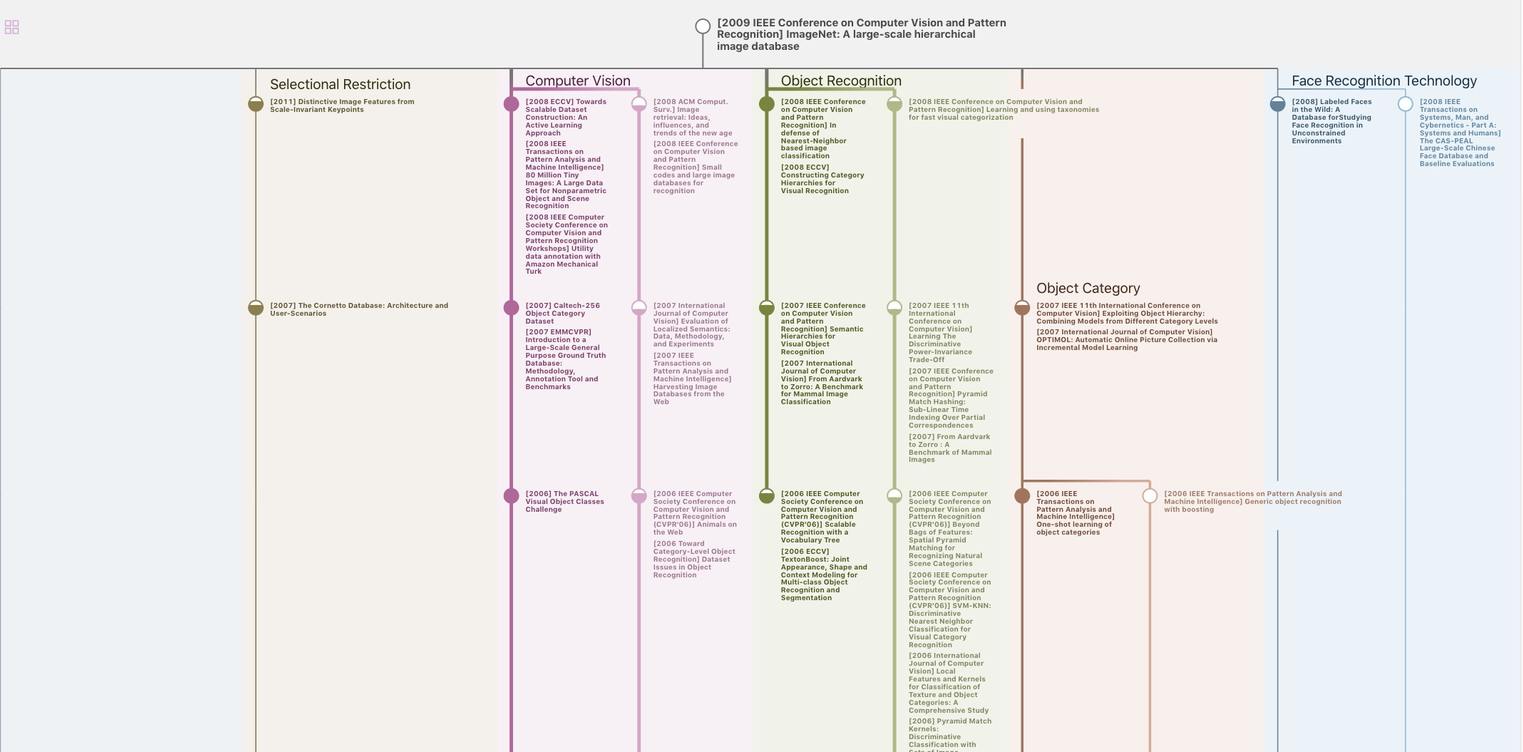
生成溯源树,研究论文发展脉络
Chat Paper
正在生成论文摘要