CausalAgents: A Robustness Benchmark for Motion Forecasting
ICRA 2024(2024)
Abstract
As machine learning models become increasingly prevalent in motion forecasting for autonomous vehicles (AVs), it is critical to ensure that model predictions are safe and reliable. In this paper, we examine the robustness of motion forecasting to non-causal perturbations. We construct a new benchmark for evaluating and improving model robustness by applying perturbations to existing data. Specifically, we conduct an extensive labeling effort to identify causal agents, or agents whose presence influences human drivers' behavior, in the Waymo Open Motion Dataset (WOMD), and we use these labels to perturb the data by deleting non-causal agents from the scene. We evaluate a diverse set of state-of-the-art deep-learning models on our proposed benchmark and find that all evaluated models exhibit large shifts under non-causal perturbation: we observe a surprising 25-38% relative change in minADE as compared to the original. In addition, we investigate techniques to improve model robustness, including increasing the training dataset size and using targeted data augmentations that randomly drop non-causal agents throughout training. Finally, we release the causal agent labels as an extension to WOMD and the robustness benchmarks to aid the community in building more reliable and safe deep-learning models for motion forecasting.
MoreTranslated text
Key words
Intelligent Transportation Systems,Deep Learning Methods,Performance Evaluation and Benchmarking
AI Read Science
Must-Reading Tree
Example
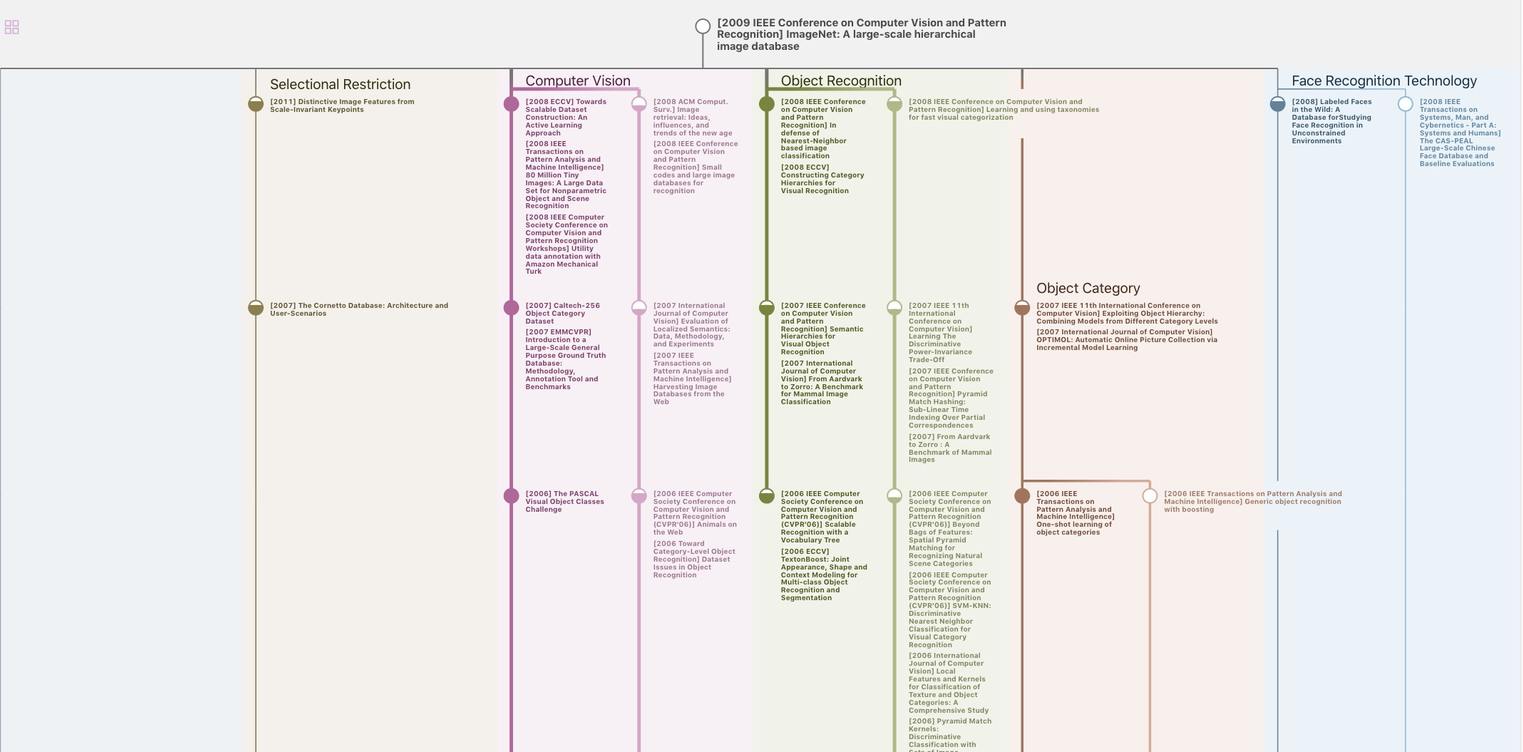
Generate MRT to find the research sequence of this paper
Chat Paper
Summary is being generated by the instructions you defined