InterRep: A Visual Interaction Representation for Robotic Grasping
ICRA 2024(2024)
Abstract
Recently, pre-trained vision models have gained significant attention in motor control, showcasing impressive performance across diverse robotic learning tasks. While previous works predominantly concentrate on the significance of the pre-training phase, the equally important task of extracting more effective representations based on existing pre-trained visual models remains unexplored. To better leverage the representation capabilities of pre-trained models for robotic grasping, we propose InterRep, a novel interaction representation method that possesses not only the strengths of pre-trained models, known for their robustness in noisy environments and their proficiency in recognizing essential features, but also the capacity of capturing dynamic interaction details and local geometric features during the grasping process. Based on the novel representation, we introduce a deep reinforcement learning method to learn generalizable grasping policies. The experimental results demonstrate that our proposed representation outperforms the baselines in terms of both training speed and generalization. For the generalized grasping tasks with dexterous robotic hands, our method boasts a success rate nearly 20% higher than methods using the global features of the entire image from pre-trained models. In addition, our proposed representation method demonstrates promising performance when applied to a different robotic hand and task. It also exhibits excellent performance on real robots with a success rate of 70%.
MoreTranslated text
Key words
Grasping,Representation Learning,Reinforcement Learning
AI Read Science
Must-Reading Tree
Example
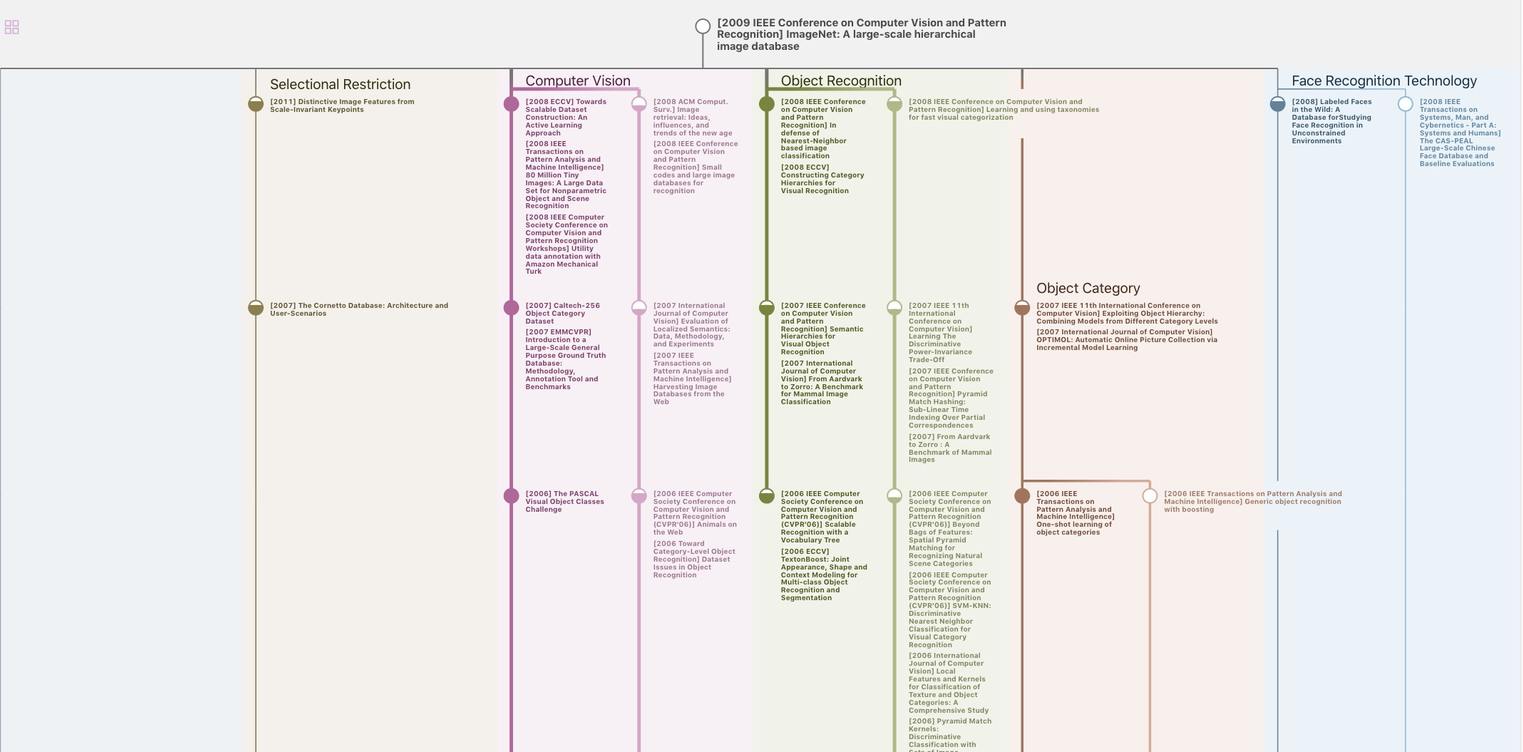
Generate MRT to find the research sequence of this paper
Chat Paper
Summary is being generated by the instructions you defined