Tactile Embeddings for Multi-Task Learning
ICRA 2024(2024)
摘要
Tactile sensing plays a pivotal role in human perception and manipulation tasks, allowing us to intuitively understand task dynamics and adapt our actions in real time. Transferring such tactile intelligence to robotic systems would help intelligent agents understand task constraints and accurately interpret the dynamics of both the objects they are interacting with and their own operations. While significant progress has been made in imbuing robots with this tactile intelligence, challenges persist in effectively utilizing tactile information due to the diversity of tactile sensor form factors, manipulation tasks, and learning objectives involved. To address this challenge, we present a unified tactile embedding space capable of predicting a variety of task-centric qualities over multiple manipulation tasks. We collect tactile data from human demonstrations across various tasks and leverage this data to construct a shared latent space for task stage classification, object dynamics estimation, and tactile dynamics prediction. Through experiments and ablation studies, we demonstrate the effectiveness of our shared tactile latent space for more accurate and adaptable tactile networks, showing an improvement of up to 84% over the single-task training.
更多查看译文
关键词
Force and Tactile Sensing,Perception for Grasping and Manipulation
AI 理解论文
溯源树
样例
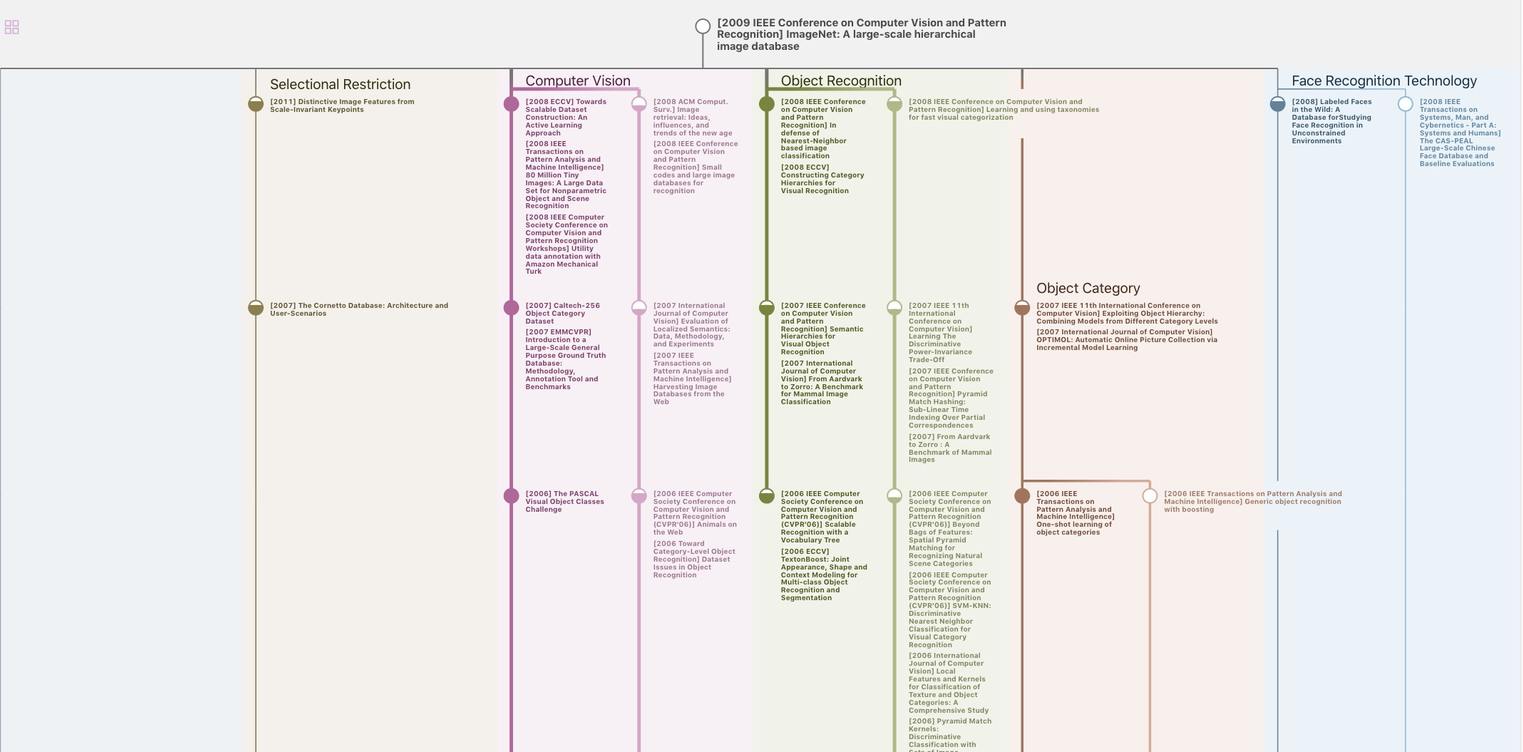
生成溯源树,研究论文发展脉络
Chat Paper
正在生成论文摘要