Hierarchical Human-To-Robot Imitation Learning for Long-Horizon Tasks Via Cross-Domain Skill Alignment
ICRA 2024(2024)
Abstract
For a general-purpose robot, it is desirable to imitate human demonstration videos that can effectively solve long-horizon tasks and perform novel ones. Recent advances in skill-based imitation learning have shown that extracting skill embedding from raw human videos is a promising paradigm to enable robots to cope with long-horizon tasks. However, generalization to unseen tasks in a different domain with a human prompt video poses a significant challenge due to the big embodiment and environment difference. To this end, we present Hierarchical Human-to-Robot Imitation Learning (H2RIL) that learns the mapping of cross-domain sensorimotor skills and utilizes it to generalize to unseen tasks given a human video in a different environment. To allow for generalizing zero-shot across environments and embodiments, H2RIL leverages task-agnostic play data for low-level policy training and paired human-robot data for both semantic and temporal skill embedding alignment. Extensive experiments in a simulated kitchen environment demonstrate that H2RIL significantly outperforms other prior baselines and is capable of generalizing to composable new tasks and adapting to Out-of-Distribution (OOD) tasks.
MoreTranslated text
Key words
Imitation Learning,Deep Learning Methods,Representation Learning
AI Read Science
Must-Reading Tree
Example
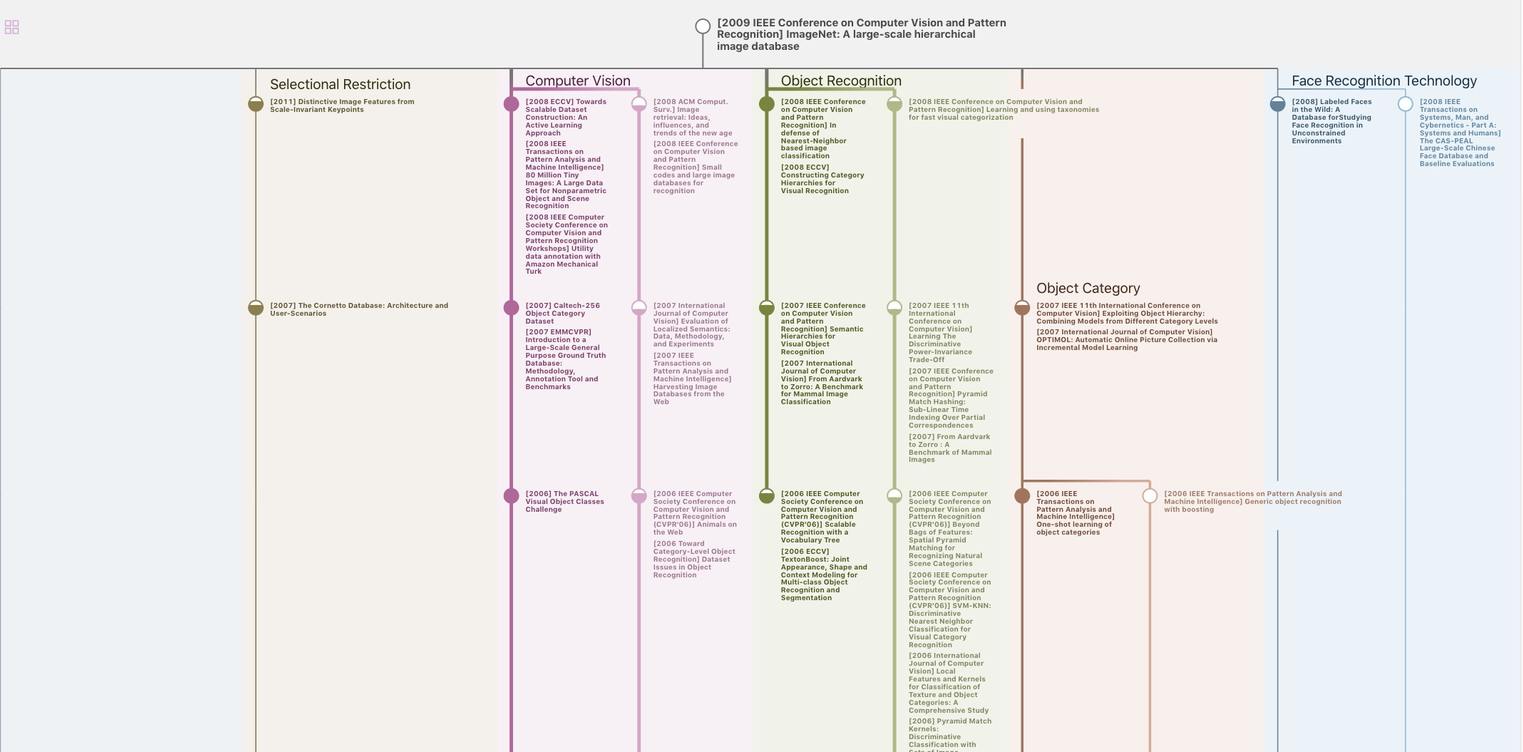
Generate MRT to find the research sequence of this paper
Chat Paper
Summary is being generated by the instructions you defined