Graph Classification Method Based on Fuzzy Entropy Causality in a Network Formal Context
IEEE Transactions on Fuzzy Systems(2024)
Abstract
Graph classification has always been a research hotspot in the field of graph neural networks and related areas. However, due to the complexity of graph data, finding a feasible algorithm that balance efficiency and accuracy remains a challenge. In this paper, a graph classification method based on fuzzy entropy causality is introduced by combining formal concept analysis, causality, and graph convolution. Firstly, the network formal context is combined with graph convolutional neural networks, forming “graph network formal context,” providing a solid foundation for the convergence of these two theoretical domains. Additionally, a high-order graph convolutional neural network (High-Order GCN) is thoroughly studied, leading to the design of high-order cluster information aggregation algorithm (HO-CIA) and high-order aggregation hierarchical graph pooling algorithm (HAGP). These innovations comprehensively capture fundamental information in graphs. Simultaneously, combining fuzzy entropy with Pearl's causality, creating a fuzzy entropy causality classification method (FECCM). Finally, to validate the effectiveness of the proposed model, classification performance comparison experiments are conducted using 12 graph datasets and 9 UCI classification datasets, demonstrating the advanced nature of HAGP-FECCM.
MoreTranslated text
Key words
Causality,formal concept analysis,fuzzy entropy,graph classification,network formal context
AI Read Science
Must-Reading Tree
Example
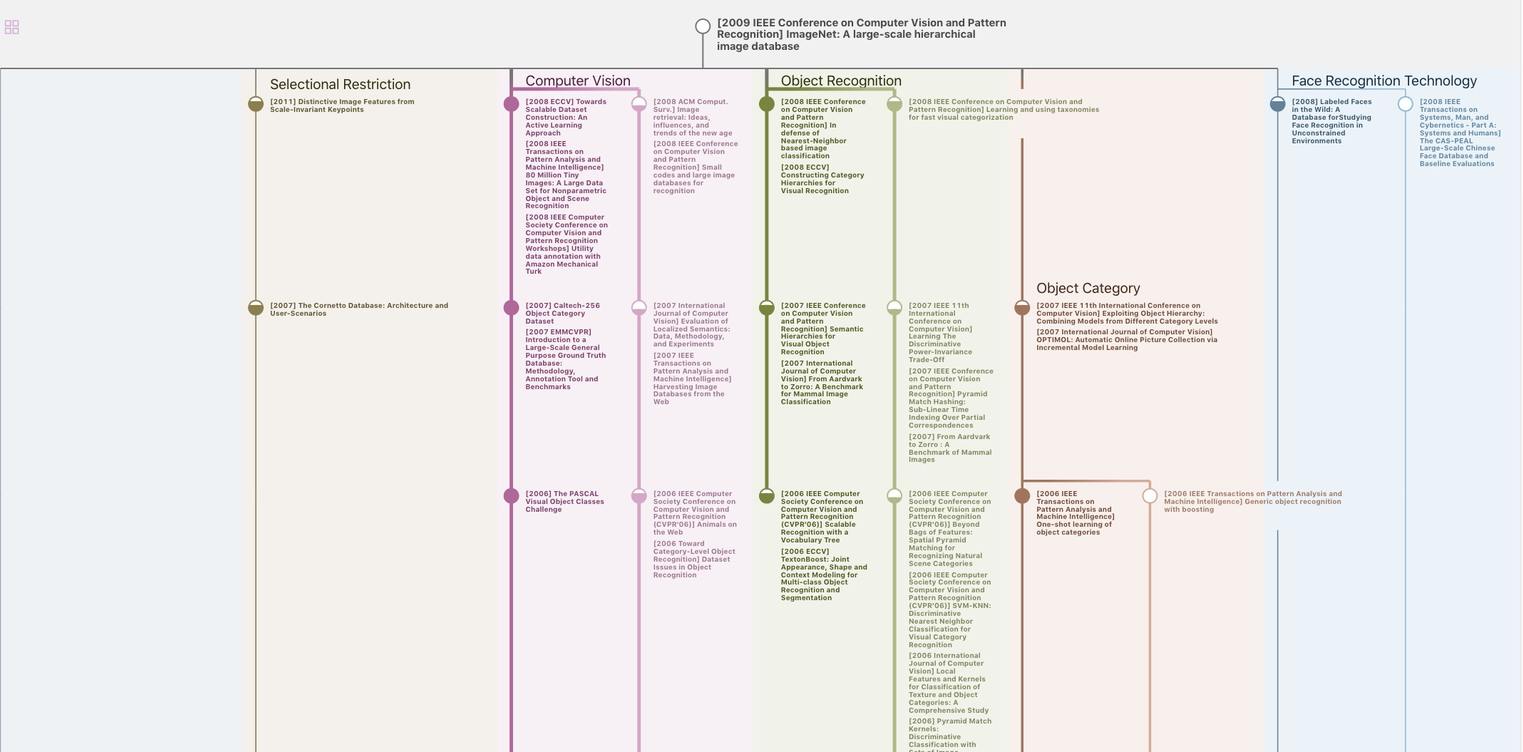
Generate MRT to find the research sequence of this paper
Chat Paper
Summary is being generated by the instructions you defined